overfit a Random Forest
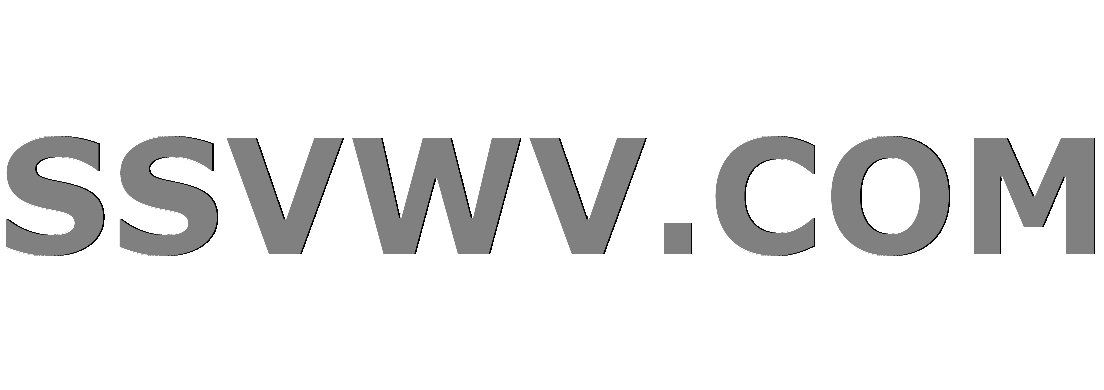
Multi tool use
$begingroup$
I am trying to overfit to the maximum a random forest classifier using scikit-learn to make some tests.
Does somebody know what hyperparameters I can tune to do that? Or does somebody know which other model I could apply to achieve a overfitted to the maximum a non-linear model?
random-forest overfitting hyperparameter-tuning
$endgroup$
add a comment |
$begingroup$
I am trying to overfit to the maximum a random forest classifier using scikit-learn to make some tests.
Does somebody know what hyperparameters I can tune to do that? Or does somebody know which other model I could apply to achieve a overfitted to the maximum a non-linear model?
random-forest overfitting hyperparameter-tuning
$endgroup$
add a comment |
$begingroup$
I am trying to overfit to the maximum a random forest classifier using scikit-learn to make some tests.
Does somebody know what hyperparameters I can tune to do that? Or does somebody know which other model I could apply to achieve a overfitted to the maximum a non-linear model?
random-forest overfitting hyperparameter-tuning
$endgroup$
I am trying to overfit to the maximum a random forest classifier using scikit-learn to make some tests.
Does somebody know what hyperparameters I can tune to do that? Or does somebody know which other model I could apply to achieve a overfitted to the maximum a non-linear model?
random-forest overfitting hyperparameter-tuning
random-forest overfitting hyperparameter-tuning
edited Sep 3 '18 at 14:23


Stephen Rauch♦
1,52551330
1,52551330
asked Sep 3 '18 at 9:06
Paul VblPaul Vbl
161
161
add a comment |
add a comment |
2 Answers
2
active
oldest
votes
$begingroup$
Decision Trees are definitely easier to overfit than Random Forests. The averaging effect (see bagging) is meant to combat overfitting.
Other than that I think the default parameters will overfit.
Example:
from sklearn.tree import DecisionTreeRegressor
# Create a dataset
x = np.linspace(0, 10 * np.pi, 50).reshape(-1,1)
y = x + 3 * np.sin(x)
noise = np.random.random(50).reshape(-1,1)
noise -= noise.mean() # center noise at 0
noisy = y + noise * 2
# Define a Decision Tree (with default parameters)
dtr = DecisionTreeRegressor()
dtr.fit(x, noisy)
y_dtr = dtr.predict(x)
# Draw the two plots
plt.figure(figsize=(14, 4))
ax1 = plt.subplot(121)
ax1.plot(np.linspace(0, 10 * np.pi, 100),
np.linspace(0, 10 * np.pi, 100) + 3 * np.sin(np.linspace(0, 10 * np.pi, 100)),
color='gray', label='desired fit', zorder=-1, alpha=0.5)
ax1.plot(x, y_dtr, color='#ff7f0e', label='decision tree', zorder=-1)
ax1.scatter(x, noisy, label='data')
ax1.set_xlabel('x')
ax1.set_ylabel('y')
ax1.set_title('Model Overfit')
ax1.spines['right'].set_visible(False)
ax1.spines['top'].set_visible(False)
ax1.yaxis.set_ticks_position('left')
ax1.xaxis.set_ticks_position('bottom')
ax1.legend()
ax2 = plt.subplot(122)
ax2.plot(np.linspace(0, 10 * np.pi, 100),
np.linspace(0, 10 * np.pi, 100) + 3 * np.sin(np.linspace(0, 10 * np.pi, 100)),
color='gray', label='desired fit', zorder=-1, alpha=0.5)
ax2.plot(x, y_dtr, color='#ff7f0e', label='decision tree', zorder=-1)
ax2.set_xlabel('x')
ax2.set_ylabel('y')
ax2.set_title('Same graph')
ax2.spines['right'].set_visible(False)
ax2.spines['top'].set_visible(False)
ax2.yaxis.set_ticks_position('left')
ax2.xaxis.set_ticks_position('bottom')
ax2.legend()
Running the code below will produce the following figure:
$endgroup$
add a comment |
$begingroup$
I was doing very similar exercise. I've generated the synthetic dataset:
y = 10 * x + noise
and fitted one Random Forest model with full trees and one with pruned:
# ranadom forest with full trees
rf = RandomForestRegressor(n_estimators=50)
# random forest with pruned trees
rf = RandomForestRegressor(n_estimators=50, min_samples_leaf=25)
I got following predictions on test data:
As you can see the Random Forest with full trees clearly overfit while Random Forest with pruned trees generalize much better. Here is a link for my full experiment.
$endgroup$
add a comment |
Your Answer
StackExchange.ifUsing("editor", function () {
return StackExchange.using("mathjaxEditing", function () {
StackExchange.MarkdownEditor.creationCallbacks.add(function (editor, postfix) {
StackExchange.mathjaxEditing.prepareWmdForMathJax(editor, postfix, [["$", "$"], ["\\(","\\)"]]);
});
});
}, "mathjax-editing");
StackExchange.ready(function() {
var channelOptions = {
tags: "".split(" "),
id: "557"
};
initTagRenderer("".split(" "), "".split(" "), channelOptions);
StackExchange.using("externalEditor", function() {
// Have to fire editor after snippets, if snippets enabled
if (StackExchange.settings.snippets.snippetsEnabled) {
StackExchange.using("snippets", function() {
createEditor();
});
}
else {
createEditor();
}
});
function createEditor() {
StackExchange.prepareEditor({
heartbeatType: 'answer',
autoActivateHeartbeat: false,
convertImagesToLinks: false,
noModals: true,
showLowRepImageUploadWarning: true,
reputationToPostImages: null,
bindNavPrevention: true,
postfix: "",
imageUploader: {
brandingHtml: "Powered by u003ca class="icon-imgur-white" href="https://imgur.com/"u003eu003c/au003e",
contentPolicyHtml: "User contributions licensed under u003ca href="https://creativecommons.org/licenses/by-sa/3.0/"u003ecc by-sa 3.0 with attribution requiredu003c/au003e u003ca href="https://stackoverflow.com/legal/content-policy"u003e(content policy)u003c/au003e",
allowUrls: true
},
onDemand: true,
discardSelector: ".discard-answer"
,immediatelyShowMarkdownHelp:true
});
}
});
Sign up or log in
StackExchange.ready(function () {
StackExchange.helpers.onClickDraftSave('#login-link');
});
Sign up using Google
Sign up using Facebook
Sign up using Email and Password
Post as a guest
Required, but never shown
StackExchange.ready(
function () {
StackExchange.openid.initPostLogin('.new-post-login', 'https%3a%2f%2fdatascience.stackexchange.com%2fquestions%2f37744%2foverfit-a-random-forest%23new-answer', 'question_page');
}
);
Post as a guest
Required, but never shown
2 Answers
2
active
oldest
votes
2 Answers
2
active
oldest
votes
active
oldest
votes
active
oldest
votes
$begingroup$
Decision Trees are definitely easier to overfit than Random Forests. The averaging effect (see bagging) is meant to combat overfitting.
Other than that I think the default parameters will overfit.
Example:
from sklearn.tree import DecisionTreeRegressor
# Create a dataset
x = np.linspace(0, 10 * np.pi, 50).reshape(-1,1)
y = x + 3 * np.sin(x)
noise = np.random.random(50).reshape(-1,1)
noise -= noise.mean() # center noise at 0
noisy = y + noise * 2
# Define a Decision Tree (with default parameters)
dtr = DecisionTreeRegressor()
dtr.fit(x, noisy)
y_dtr = dtr.predict(x)
# Draw the two plots
plt.figure(figsize=(14, 4))
ax1 = plt.subplot(121)
ax1.plot(np.linspace(0, 10 * np.pi, 100),
np.linspace(0, 10 * np.pi, 100) + 3 * np.sin(np.linspace(0, 10 * np.pi, 100)),
color='gray', label='desired fit', zorder=-1, alpha=0.5)
ax1.plot(x, y_dtr, color='#ff7f0e', label='decision tree', zorder=-1)
ax1.scatter(x, noisy, label='data')
ax1.set_xlabel('x')
ax1.set_ylabel('y')
ax1.set_title('Model Overfit')
ax1.spines['right'].set_visible(False)
ax1.spines['top'].set_visible(False)
ax1.yaxis.set_ticks_position('left')
ax1.xaxis.set_ticks_position('bottom')
ax1.legend()
ax2 = plt.subplot(122)
ax2.plot(np.linspace(0, 10 * np.pi, 100),
np.linspace(0, 10 * np.pi, 100) + 3 * np.sin(np.linspace(0, 10 * np.pi, 100)),
color='gray', label='desired fit', zorder=-1, alpha=0.5)
ax2.plot(x, y_dtr, color='#ff7f0e', label='decision tree', zorder=-1)
ax2.set_xlabel('x')
ax2.set_ylabel('y')
ax2.set_title('Same graph')
ax2.spines['right'].set_visible(False)
ax2.spines['top'].set_visible(False)
ax2.yaxis.set_ticks_position('left')
ax2.xaxis.set_ticks_position('bottom')
ax2.legend()
Running the code below will produce the following figure:
$endgroup$
add a comment |
$begingroup$
Decision Trees are definitely easier to overfit than Random Forests. The averaging effect (see bagging) is meant to combat overfitting.
Other than that I think the default parameters will overfit.
Example:
from sklearn.tree import DecisionTreeRegressor
# Create a dataset
x = np.linspace(0, 10 * np.pi, 50).reshape(-1,1)
y = x + 3 * np.sin(x)
noise = np.random.random(50).reshape(-1,1)
noise -= noise.mean() # center noise at 0
noisy = y + noise * 2
# Define a Decision Tree (with default parameters)
dtr = DecisionTreeRegressor()
dtr.fit(x, noisy)
y_dtr = dtr.predict(x)
# Draw the two plots
plt.figure(figsize=(14, 4))
ax1 = plt.subplot(121)
ax1.plot(np.linspace(0, 10 * np.pi, 100),
np.linspace(0, 10 * np.pi, 100) + 3 * np.sin(np.linspace(0, 10 * np.pi, 100)),
color='gray', label='desired fit', zorder=-1, alpha=0.5)
ax1.plot(x, y_dtr, color='#ff7f0e', label='decision tree', zorder=-1)
ax1.scatter(x, noisy, label='data')
ax1.set_xlabel('x')
ax1.set_ylabel('y')
ax1.set_title('Model Overfit')
ax1.spines['right'].set_visible(False)
ax1.spines['top'].set_visible(False)
ax1.yaxis.set_ticks_position('left')
ax1.xaxis.set_ticks_position('bottom')
ax1.legend()
ax2 = plt.subplot(122)
ax2.plot(np.linspace(0, 10 * np.pi, 100),
np.linspace(0, 10 * np.pi, 100) + 3 * np.sin(np.linspace(0, 10 * np.pi, 100)),
color='gray', label='desired fit', zorder=-1, alpha=0.5)
ax2.plot(x, y_dtr, color='#ff7f0e', label='decision tree', zorder=-1)
ax2.set_xlabel('x')
ax2.set_ylabel('y')
ax2.set_title('Same graph')
ax2.spines['right'].set_visible(False)
ax2.spines['top'].set_visible(False)
ax2.yaxis.set_ticks_position('left')
ax2.xaxis.set_ticks_position('bottom')
ax2.legend()
Running the code below will produce the following figure:
$endgroup$
add a comment |
$begingroup$
Decision Trees are definitely easier to overfit than Random Forests. The averaging effect (see bagging) is meant to combat overfitting.
Other than that I think the default parameters will overfit.
Example:
from sklearn.tree import DecisionTreeRegressor
# Create a dataset
x = np.linspace(0, 10 * np.pi, 50).reshape(-1,1)
y = x + 3 * np.sin(x)
noise = np.random.random(50).reshape(-1,1)
noise -= noise.mean() # center noise at 0
noisy = y + noise * 2
# Define a Decision Tree (with default parameters)
dtr = DecisionTreeRegressor()
dtr.fit(x, noisy)
y_dtr = dtr.predict(x)
# Draw the two plots
plt.figure(figsize=(14, 4))
ax1 = plt.subplot(121)
ax1.plot(np.linspace(0, 10 * np.pi, 100),
np.linspace(0, 10 * np.pi, 100) + 3 * np.sin(np.linspace(0, 10 * np.pi, 100)),
color='gray', label='desired fit', zorder=-1, alpha=0.5)
ax1.plot(x, y_dtr, color='#ff7f0e', label='decision tree', zorder=-1)
ax1.scatter(x, noisy, label='data')
ax1.set_xlabel('x')
ax1.set_ylabel('y')
ax1.set_title('Model Overfit')
ax1.spines['right'].set_visible(False)
ax1.spines['top'].set_visible(False)
ax1.yaxis.set_ticks_position('left')
ax1.xaxis.set_ticks_position('bottom')
ax1.legend()
ax2 = plt.subplot(122)
ax2.plot(np.linspace(0, 10 * np.pi, 100),
np.linspace(0, 10 * np.pi, 100) + 3 * np.sin(np.linspace(0, 10 * np.pi, 100)),
color='gray', label='desired fit', zorder=-1, alpha=0.5)
ax2.plot(x, y_dtr, color='#ff7f0e', label='decision tree', zorder=-1)
ax2.set_xlabel('x')
ax2.set_ylabel('y')
ax2.set_title('Same graph')
ax2.spines['right'].set_visible(False)
ax2.spines['top'].set_visible(False)
ax2.yaxis.set_ticks_position('left')
ax2.xaxis.set_ticks_position('bottom')
ax2.legend()
Running the code below will produce the following figure:
$endgroup$
Decision Trees are definitely easier to overfit than Random Forests. The averaging effect (see bagging) is meant to combat overfitting.
Other than that I think the default parameters will overfit.
Example:
from sklearn.tree import DecisionTreeRegressor
# Create a dataset
x = np.linspace(0, 10 * np.pi, 50).reshape(-1,1)
y = x + 3 * np.sin(x)
noise = np.random.random(50).reshape(-1,1)
noise -= noise.mean() # center noise at 0
noisy = y + noise * 2
# Define a Decision Tree (with default parameters)
dtr = DecisionTreeRegressor()
dtr.fit(x, noisy)
y_dtr = dtr.predict(x)
# Draw the two plots
plt.figure(figsize=(14, 4))
ax1 = plt.subplot(121)
ax1.plot(np.linspace(0, 10 * np.pi, 100),
np.linspace(0, 10 * np.pi, 100) + 3 * np.sin(np.linspace(0, 10 * np.pi, 100)),
color='gray', label='desired fit', zorder=-1, alpha=0.5)
ax1.plot(x, y_dtr, color='#ff7f0e', label='decision tree', zorder=-1)
ax1.scatter(x, noisy, label='data')
ax1.set_xlabel('x')
ax1.set_ylabel('y')
ax1.set_title('Model Overfit')
ax1.spines['right'].set_visible(False)
ax1.spines['top'].set_visible(False)
ax1.yaxis.set_ticks_position('left')
ax1.xaxis.set_ticks_position('bottom')
ax1.legend()
ax2 = plt.subplot(122)
ax2.plot(np.linspace(0, 10 * np.pi, 100),
np.linspace(0, 10 * np.pi, 100) + 3 * np.sin(np.linspace(0, 10 * np.pi, 100)),
color='gray', label='desired fit', zorder=-1, alpha=0.5)
ax2.plot(x, y_dtr, color='#ff7f0e', label='decision tree', zorder=-1)
ax2.set_xlabel('x')
ax2.set_ylabel('y')
ax2.set_title('Same graph')
ax2.spines['right'].set_visible(False)
ax2.spines['top'].set_visible(False)
ax2.yaxis.set_ticks_position('left')
ax2.xaxis.set_ticks_position('bottom')
ax2.legend()
Running the code below will produce the following figure:
answered Sep 3 '18 at 9:53
Djib2011Djib2011
2,62731125
2,62731125
add a comment |
add a comment |
$begingroup$
I was doing very similar exercise. I've generated the synthetic dataset:
y = 10 * x + noise
and fitted one Random Forest model with full trees and one with pruned:
# ranadom forest with full trees
rf = RandomForestRegressor(n_estimators=50)
# random forest with pruned trees
rf = RandomForestRegressor(n_estimators=50, min_samples_leaf=25)
I got following predictions on test data:
As you can see the Random Forest with full trees clearly overfit while Random Forest with pruned trees generalize much better. Here is a link for my full experiment.
$endgroup$
add a comment |
$begingroup$
I was doing very similar exercise. I've generated the synthetic dataset:
y = 10 * x + noise
and fitted one Random Forest model with full trees and one with pruned:
# ranadom forest with full trees
rf = RandomForestRegressor(n_estimators=50)
# random forest with pruned trees
rf = RandomForestRegressor(n_estimators=50, min_samples_leaf=25)
I got following predictions on test data:
As you can see the Random Forest with full trees clearly overfit while Random Forest with pruned trees generalize much better. Here is a link for my full experiment.
$endgroup$
add a comment |
$begingroup$
I was doing very similar exercise. I've generated the synthetic dataset:
y = 10 * x + noise
and fitted one Random Forest model with full trees and one with pruned:
# ranadom forest with full trees
rf = RandomForestRegressor(n_estimators=50)
# random forest with pruned trees
rf = RandomForestRegressor(n_estimators=50, min_samples_leaf=25)
I got following predictions on test data:
As you can see the Random Forest with full trees clearly overfit while Random Forest with pruned trees generalize much better. Here is a link for my full experiment.
$endgroup$
I was doing very similar exercise. I've generated the synthetic dataset:
y = 10 * x + noise
and fitted one Random Forest model with full trees and one with pruned:
# ranadom forest with full trees
rf = RandomForestRegressor(n_estimators=50)
# random forest with pruned trees
rf = RandomForestRegressor(n_estimators=50, min_samples_leaf=25)
I got following predictions on test data:
As you can see the Random Forest with full trees clearly overfit while Random Forest with pruned trees generalize much better. Here is a link for my full experiment.
answered yesterday


pplonskipplonski
21115
21115
add a comment |
add a comment |
Thanks for contributing an answer to Data Science Stack Exchange!
- Please be sure to answer the question. Provide details and share your research!
But avoid …
- Asking for help, clarification, or responding to other answers.
- Making statements based on opinion; back them up with references or personal experience.
Use MathJax to format equations. MathJax reference.
To learn more, see our tips on writing great answers.
Sign up or log in
StackExchange.ready(function () {
StackExchange.helpers.onClickDraftSave('#login-link');
});
Sign up using Google
Sign up using Facebook
Sign up using Email and Password
Post as a guest
Required, but never shown
StackExchange.ready(
function () {
StackExchange.openid.initPostLogin('.new-post-login', 'https%3a%2f%2fdatascience.stackexchange.com%2fquestions%2f37744%2foverfit-a-random-forest%23new-answer', 'question_page');
}
);
Post as a guest
Required, but never shown
Sign up or log in
StackExchange.ready(function () {
StackExchange.helpers.onClickDraftSave('#login-link');
});
Sign up using Google
Sign up using Facebook
Sign up using Email and Password
Post as a guest
Required, but never shown
Sign up or log in
StackExchange.ready(function () {
StackExchange.helpers.onClickDraftSave('#login-link');
});
Sign up using Google
Sign up using Facebook
Sign up using Email and Password
Post as a guest
Required, but never shown
Sign up or log in
StackExchange.ready(function () {
StackExchange.helpers.onClickDraftSave('#login-link');
});
Sign up using Google
Sign up using Facebook
Sign up using Email and Password
Sign up using Google
Sign up using Facebook
Sign up using Email and Password
Post as a guest
Required, but never shown
Required, but never shown
Required, but never shown
Required, but never shown
Required, but never shown
Required, but never shown
Required, but never shown
Required, but never shown
Required, but never shown
UWXAIR Ik,wp6 7Ivp VH x9N82DAdDwt9o9cXZj9a2,gNoI SoYB6Ul