How to create an ensemble that gives precedence to a specific classifier
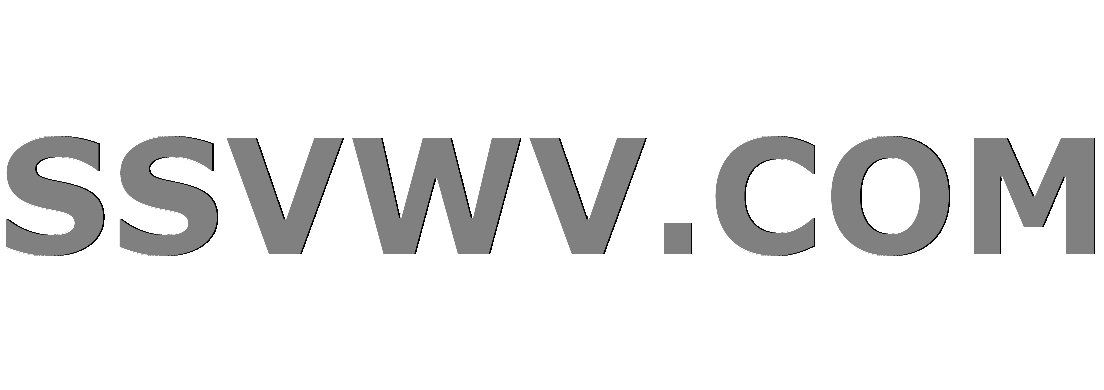
Multi tool use
$begingroup$
Suppose that in a binary classification task, I have separate classifiers A
, B
, and C
. If I use A
alone, I will get a high precision, but low recall. In other words, the number of true positives are very high, but it also incorrectly tags the rest of the labels as False
. B
, and C
have much lower precision, but when used separately, they may (or may not) result in better recall. How can I define an ensemble classifier that gives precedence to classifier A
only in cases where it labels the data as True
and give more weight to the predictions of other classifiers when A
predicts the label as False
.
The idea is, A
is already outperforming others in catching true positives and I only want to improve the recall without hurting precision.
classification prediction ensemble-modeling binary ensemble
$endgroup$
bumped to the homepage by Community♦ yesterday
This question has answers that may be good or bad; the system has marked it active so that they can be reviewed.
add a comment |
$begingroup$
Suppose that in a binary classification task, I have separate classifiers A
, B
, and C
. If I use A
alone, I will get a high precision, but low recall. In other words, the number of true positives are very high, but it also incorrectly tags the rest of the labels as False
. B
, and C
have much lower precision, but when used separately, they may (or may not) result in better recall. How can I define an ensemble classifier that gives precedence to classifier A
only in cases where it labels the data as True
and give more weight to the predictions of other classifiers when A
predicts the label as False
.
The idea is, A
is already outperforming others in catching true positives and I only want to improve the recall without hurting precision.
classification prediction ensemble-modeling binary ensemble
$endgroup$
bumped to the homepage by Community♦ yesterday
This question has answers that may be good or bad; the system has marked it active so that they can be reviewed.
4
$begingroup$
Ensemble models learn the correct weights for you. Read about boosting and stacking. You can tune the ensemble classifier to yield the recall/precision trade-off you desire. Welcome to the site!
$endgroup$
– Emre
Jan 11 '18 at 19:27
$begingroup$
can you describe the data? what kind of classifiers you are using?
$endgroup$
– Bashar Haddad
Apr 12 '18 at 0:35
add a comment |
$begingroup$
Suppose that in a binary classification task, I have separate classifiers A
, B
, and C
. If I use A
alone, I will get a high precision, but low recall. In other words, the number of true positives are very high, but it also incorrectly tags the rest of the labels as False
. B
, and C
have much lower precision, but when used separately, they may (or may not) result in better recall. How can I define an ensemble classifier that gives precedence to classifier A
only in cases where it labels the data as True
and give more weight to the predictions of other classifiers when A
predicts the label as False
.
The idea is, A
is already outperforming others in catching true positives and I only want to improve the recall without hurting precision.
classification prediction ensemble-modeling binary ensemble
$endgroup$
Suppose that in a binary classification task, I have separate classifiers A
, B
, and C
. If I use A
alone, I will get a high precision, but low recall. In other words, the number of true positives are very high, but it also incorrectly tags the rest of the labels as False
. B
, and C
have much lower precision, but when used separately, they may (or may not) result in better recall. How can I define an ensemble classifier that gives precedence to classifier A
only in cases where it labels the data as True
and give more weight to the predictions of other classifiers when A
predicts the label as False
.
The idea is, A
is already outperforming others in catching true positives and I only want to improve the recall without hurting precision.
classification prediction ensemble-modeling binary ensemble
classification prediction ensemble-modeling binary ensemble
asked Jan 11 '18 at 19:13
Clement AttleeClement Attlee
111
111
bumped to the homepage by Community♦ yesterday
This question has answers that may be good or bad; the system has marked it active so that they can be reviewed.
bumped to the homepage by Community♦ yesterday
This question has answers that may be good or bad; the system has marked it active so that they can be reviewed.
4
$begingroup$
Ensemble models learn the correct weights for you. Read about boosting and stacking. You can tune the ensemble classifier to yield the recall/precision trade-off you desire. Welcome to the site!
$endgroup$
– Emre
Jan 11 '18 at 19:27
$begingroup$
can you describe the data? what kind of classifiers you are using?
$endgroup$
– Bashar Haddad
Apr 12 '18 at 0:35
add a comment |
4
$begingroup$
Ensemble models learn the correct weights for you. Read about boosting and stacking. You can tune the ensemble classifier to yield the recall/precision trade-off you desire. Welcome to the site!
$endgroup$
– Emre
Jan 11 '18 at 19:27
$begingroup$
can you describe the data? what kind of classifiers you are using?
$endgroup$
– Bashar Haddad
Apr 12 '18 at 0:35
4
4
$begingroup$
Ensemble models learn the correct weights for you. Read about boosting and stacking. You can tune the ensemble classifier to yield the recall/precision trade-off you desire. Welcome to the site!
$endgroup$
– Emre
Jan 11 '18 at 19:27
$begingroup$
Ensemble models learn the correct weights for you. Read about boosting and stacking. You can tune the ensemble classifier to yield the recall/precision trade-off you desire. Welcome to the site!
$endgroup$
– Emre
Jan 11 '18 at 19:27
$begingroup$
can you describe the data? what kind of classifiers you are using?
$endgroup$
– Bashar Haddad
Apr 12 '18 at 0:35
$begingroup$
can you describe the data? what kind of classifiers you are using?
$endgroup$
– Bashar Haddad
Apr 12 '18 at 0:35
add a comment |
2 Answers
2
active
oldest
votes
$begingroup$
Feature-Weighted Linear Stacking might be what you are looking for.
FWLS combines model predictions linearly using coefficients that are
themselves linear functions of meta-features.
In your example you can use the meta-feature "Does A
label the example as True
?"
$endgroup$
add a comment |
$begingroup$
based on your description, it looks like different models have different biases. two important questions: do you have any data imbalance problem? what kind of models you are using? using stacking based classifier is beneficial if you have different biases. Try to use a simple stack based classifier.
for your level-1 classifier, use different models (e.g. SVM-L, SVM-NL, DT, RF, ... etc). For your meta-data, use probabilities and for the meta-classifier use Random Forest.
if you have data imbalance problem using stack based classifier is a little bit more challenging.
$endgroup$
add a comment |
Your Answer
StackExchange.ifUsing("editor", function () {
return StackExchange.using("mathjaxEditing", function () {
StackExchange.MarkdownEditor.creationCallbacks.add(function (editor, postfix) {
StackExchange.mathjaxEditing.prepareWmdForMathJax(editor, postfix, [["$", "$"], ["\\(","\\)"]]);
});
});
}, "mathjax-editing");
StackExchange.ready(function() {
var channelOptions = {
tags: "".split(" "),
id: "557"
};
initTagRenderer("".split(" "), "".split(" "), channelOptions);
StackExchange.using("externalEditor", function() {
// Have to fire editor after snippets, if snippets enabled
if (StackExchange.settings.snippets.snippetsEnabled) {
StackExchange.using("snippets", function() {
createEditor();
});
}
else {
createEditor();
}
});
function createEditor() {
StackExchange.prepareEditor({
heartbeatType: 'answer',
autoActivateHeartbeat: false,
convertImagesToLinks: false,
noModals: true,
showLowRepImageUploadWarning: true,
reputationToPostImages: null,
bindNavPrevention: true,
postfix: "",
imageUploader: {
brandingHtml: "Powered by u003ca class="icon-imgur-white" href="https://imgur.com/"u003eu003c/au003e",
contentPolicyHtml: "User contributions licensed under u003ca href="https://creativecommons.org/licenses/by-sa/3.0/"u003ecc by-sa 3.0 with attribution requiredu003c/au003e u003ca href="https://stackoverflow.com/legal/content-policy"u003e(content policy)u003c/au003e",
allowUrls: true
},
onDemand: true,
discardSelector: ".discard-answer"
,immediatelyShowMarkdownHelp:true
});
}
});
Sign up or log in
StackExchange.ready(function () {
StackExchange.helpers.onClickDraftSave('#login-link');
});
Sign up using Google
Sign up using Facebook
Sign up using Email and Password
Post as a guest
Required, but never shown
StackExchange.ready(
function () {
StackExchange.openid.initPostLogin('.new-post-login', 'https%3a%2f%2fdatascience.stackexchange.com%2fquestions%2f26531%2fhow-to-create-an-ensemble-that-gives-precedence-to-a-specific-classifier%23new-answer', 'question_page');
}
);
Post as a guest
Required, but never shown
2 Answers
2
active
oldest
votes
2 Answers
2
active
oldest
votes
active
oldest
votes
active
oldest
votes
$begingroup$
Feature-Weighted Linear Stacking might be what you are looking for.
FWLS combines model predictions linearly using coefficients that are
themselves linear functions of meta-features.
In your example you can use the meta-feature "Does A
label the example as True
?"
$endgroup$
add a comment |
$begingroup$
Feature-Weighted Linear Stacking might be what you are looking for.
FWLS combines model predictions linearly using coefficients that are
themselves linear functions of meta-features.
In your example you can use the meta-feature "Does A
label the example as True
?"
$endgroup$
add a comment |
$begingroup$
Feature-Weighted Linear Stacking might be what you are looking for.
FWLS combines model predictions linearly using coefficients that are
themselves linear functions of meta-features.
In your example you can use the meta-feature "Does A
label the example as True
?"
$endgroup$
Feature-Weighted Linear Stacking might be what you are looking for.
FWLS combines model predictions linearly using coefficients that are
themselves linear functions of meta-features.
In your example you can use the meta-feature "Does A
label the example as True
?"
answered Jan 11 '18 at 20:06


ImranImran
1,756619
1,756619
add a comment |
add a comment |
$begingroup$
based on your description, it looks like different models have different biases. two important questions: do you have any data imbalance problem? what kind of models you are using? using stacking based classifier is beneficial if you have different biases. Try to use a simple stack based classifier.
for your level-1 classifier, use different models (e.g. SVM-L, SVM-NL, DT, RF, ... etc). For your meta-data, use probabilities and for the meta-classifier use Random Forest.
if you have data imbalance problem using stack based classifier is a little bit more challenging.
$endgroup$
add a comment |
$begingroup$
based on your description, it looks like different models have different biases. two important questions: do you have any data imbalance problem? what kind of models you are using? using stacking based classifier is beneficial if you have different biases. Try to use a simple stack based classifier.
for your level-1 classifier, use different models (e.g. SVM-L, SVM-NL, DT, RF, ... etc). For your meta-data, use probabilities and for the meta-classifier use Random Forest.
if you have data imbalance problem using stack based classifier is a little bit more challenging.
$endgroup$
add a comment |
$begingroup$
based on your description, it looks like different models have different biases. two important questions: do you have any data imbalance problem? what kind of models you are using? using stacking based classifier is beneficial if you have different biases. Try to use a simple stack based classifier.
for your level-1 classifier, use different models (e.g. SVM-L, SVM-NL, DT, RF, ... etc). For your meta-data, use probabilities and for the meta-classifier use Random Forest.
if you have data imbalance problem using stack based classifier is a little bit more challenging.
$endgroup$
based on your description, it looks like different models have different biases. two important questions: do you have any data imbalance problem? what kind of models you are using? using stacking based classifier is beneficial if you have different biases. Try to use a simple stack based classifier.
for your level-1 classifier, use different models (e.g. SVM-L, SVM-NL, DT, RF, ... etc). For your meta-data, use probabilities and for the meta-classifier use Random Forest.
if you have data imbalance problem using stack based classifier is a little bit more challenging.
answered Apr 12 '18 at 0:39


Bashar HaddadBashar Haddad
1,2621413
1,2621413
add a comment |
add a comment |
Thanks for contributing an answer to Data Science Stack Exchange!
- Please be sure to answer the question. Provide details and share your research!
But avoid …
- Asking for help, clarification, or responding to other answers.
- Making statements based on opinion; back them up with references or personal experience.
Use MathJax to format equations. MathJax reference.
To learn more, see our tips on writing great answers.
Sign up or log in
StackExchange.ready(function () {
StackExchange.helpers.onClickDraftSave('#login-link');
});
Sign up using Google
Sign up using Facebook
Sign up using Email and Password
Post as a guest
Required, but never shown
StackExchange.ready(
function () {
StackExchange.openid.initPostLogin('.new-post-login', 'https%3a%2f%2fdatascience.stackexchange.com%2fquestions%2f26531%2fhow-to-create-an-ensemble-that-gives-precedence-to-a-specific-classifier%23new-answer', 'question_page');
}
);
Post as a guest
Required, but never shown
Sign up or log in
StackExchange.ready(function () {
StackExchange.helpers.onClickDraftSave('#login-link');
});
Sign up using Google
Sign up using Facebook
Sign up using Email and Password
Post as a guest
Required, but never shown
Sign up or log in
StackExchange.ready(function () {
StackExchange.helpers.onClickDraftSave('#login-link');
});
Sign up using Google
Sign up using Facebook
Sign up using Email and Password
Post as a guest
Required, but never shown
Sign up or log in
StackExchange.ready(function () {
StackExchange.helpers.onClickDraftSave('#login-link');
});
Sign up using Google
Sign up using Facebook
Sign up using Email and Password
Sign up using Google
Sign up using Facebook
Sign up using Email and Password
Post as a guest
Required, but never shown
Required, but never shown
Required, but never shown
Required, but never shown
Required, but never shown
Required, but never shown
Required, but never shown
Required, but never shown
Required, but never shown
yYg7E1,lUvqLJyp57,H3wx3
4
$begingroup$
Ensemble models learn the correct weights for you. Read about boosting and stacking. You can tune the ensemble classifier to yield the recall/precision trade-off you desire. Welcome to the site!
$endgroup$
– Emre
Jan 11 '18 at 19:27
$begingroup$
can you describe the data? what kind of classifiers you are using?
$endgroup$
– Bashar Haddad
Apr 12 '18 at 0:35