A question on realizable sample complexity
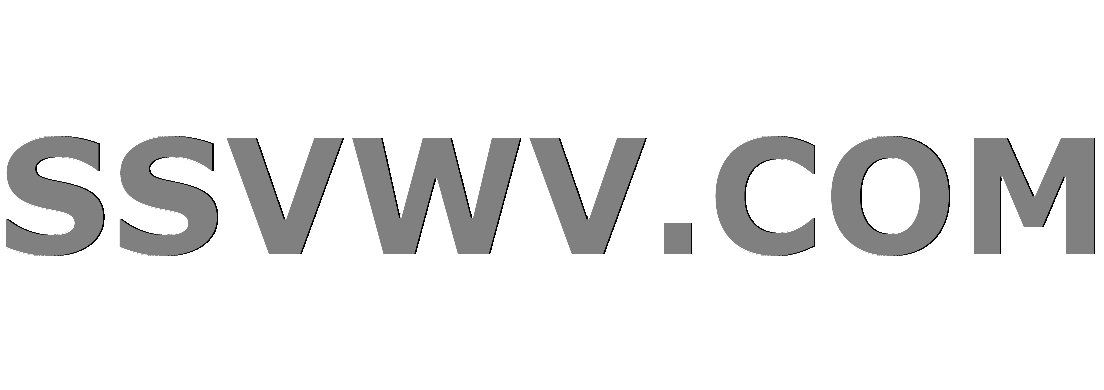
Multi tool use
$begingroup$
I came across the following exercise, and I just can't seem to crack it:
Let $l$ be some loss function such that $l leq 1$. Let $H$ be some hypothesis class, and let $A$ be a learning algorithm. show that:
$m^{text{stat, r}}_H (epsilon) = Oleft(m^{text{stat, r}}_H (epsilon/2, 1/2)cdot log(1/epsilon) + frac{log(1/epsilon)}{epsilon^2}right)$
Where $m^{text{stat, r}}_H (epsilon)$ is the minimal number $m$ such that for any realizable distribution over training examples $D$ we have that:
$$mathbb{E}_{S sim D^m}left[ l_D(A(S)) right]leq epsilon$$
And where $m^{text{stat, r}}_H (epsilon, delta)$ is the minimal number $m$ such that for any realizable distribution over training examples $D$ we have that:
$$P_{S sim D^m}left( l_D(A(S)) geq epsilon right) leq delta$$
Thanks a lot in advance!
machine-learning theory
New contributor
Nadav Schweiger is a new contributor to this site. Take care in asking for clarification, commenting, and answering.
Check out our Code of Conduct.
$endgroup$
add a comment |
$begingroup$
I came across the following exercise, and I just can't seem to crack it:
Let $l$ be some loss function such that $l leq 1$. Let $H$ be some hypothesis class, and let $A$ be a learning algorithm. show that:
$m^{text{stat, r}}_H (epsilon) = Oleft(m^{text{stat, r}}_H (epsilon/2, 1/2)cdot log(1/epsilon) + frac{log(1/epsilon)}{epsilon^2}right)$
Where $m^{text{stat, r}}_H (epsilon)$ is the minimal number $m$ such that for any realizable distribution over training examples $D$ we have that:
$$mathbb{E}_{S sim D^m}left[ l_D(A(S)) right]leq epsilon$$
And where $m^{text{stat, r}}_H (epsilon, delta)$ is the minimal number $m$ such that for any realizable distribution over training examples $D$ we have that:
$$P_{S sim D^m}left( l_D(A(S)) geq epsilon right) leq delta$$
Thanks a lot in advance!
machine-learning theory
New contributor
Nadav Schweiger is a new contributor to this site. Take care in asking for clarification, commenting, and answering.
Check out our Code of Conduct.
$endgroup$
add a comment |
$begingroup$
I came across the following exercise, and I just can't seem to crack it:
Let $l$ be some loss function such that $l leq 1$. Let $H$ be some hypothesis class, and let $A$ be a learning algorithm. show that:
$m^{text{stat, r}}_H (epsilon) = Oleft(m^{text{stat, r}}_H (epsilon/2, 1/2)cdot log(1/epsilon) + frac{log(1/epsilon)}{epsilon^2}right)$
Where $m^{text{stat, r}}_H (epsilon)$ is the minimal number $m$ such that for any realizable distribution over training examples $D$ we have that:
$$mathbb{E}_{S sim D^m}left[ l_D(A(S)) right]leq epsilon$$
And where $m^{text{stat, r}}_H (epsilon, delta)$ is the minimal number $m$ such that for any realizable distribution over training examples $D$ we have that:
$$P_{S sim D^m}left( l_D(A(S)) geq epsilon right) leq delta$$
Thanks a lot in advance!
machine-learning theory
New contributor
Nadav Schweiger is a new contributor to this site. Take care in asking for clarification, commenting, and answering.
Check out our Code of Conduct.
$endgroup$
I came across the following exercise, and I just can't seem to crack it:
Let $l$ be some loss function such that $l leq 1$. Let $H$ be some hypothesis class, and let $A$ be a learning algorithm. show that:
$m^{text{stat, r}}_H (epsilon) = Oleft(m^{text{stat, r}}_H (epsilon/2, 1/2)cdot log(1/epsilon) + frac{log(1/epsilon)}{epsilon^2}right)$
Where $m^{text{stat, r}}_H (epsilon)$ is the minimal number $m$ such that for any realizable distribution over training examples $D$ we have that:
$$mathbb{E}_{S sim D^m}left[ l_D(A(S)) right]leq epsilon$$
And where $m^{text{stat, r}}_H (epsilon, delta)$ is the minimal number $m$ such that for any realizable distribution over training examples $D$ we have that:
$$P_{S sim D^m}left( l_D(A(S)) geq epsilon right) leq delta$$
Thanks a lot in advance!
machine-learning theory
machine-learning theory
New contributor
Nadav Schweiger is a new contributor to this site. Take care in asking for clarification, commenting, and answering.
Check out our Code of Conduct.
New contributor
Nadav Schweiger is a new contributor to this site. Take care in asking for clarification, commenting, and answering.
Check out our Code of Conduct.
New contributor
Nadav Schweiger is a new contributor to this site. Take care in asking for clarification, commenting, and answering.
Check out our Code of Conduct.
asked 2 days ago
Nadav SchweigerNadav Schweiger
1
1
New contributor
Nadav Schweiger is a new contributor to this site. Take care in asking for clarification, commenting, and answering.
Check out our Code of Conduct.
New contributor
Nadav Schweiger is a new contributor to this site. Take care in asking for clarification, commenting, and answering.
Check out our Code of Conduct.
Nadav Schweiger is a new contributor to this site. Take care in asking for clarification, commenting, and answering.
Check out our Code of Conduct.
add a comment |
add a comment |
1 Answer
1
active
oldest
votes
$begingroup$
We want to prove:
If H is PAC learnable, then $forall epsilon, exists C, forall m geq m_2:=Clog(1/epsilon)(m_1+1/epsilon^2), E[L] leq epsilon mbox{ (a)}$
where $m_1:=m(epsilon/2,1/2)$
Since $L leq 1$, we have $E[L] leq 1$. So proof is trivial for $epsilon geq 1$. Let $epsilon in (0, 1)$.
Find an equivalence for $(a)$:
$begin{align*}
E[L] &= int_{l geq epsilon / 2}ldP + int_{l< epsilon / 2} ldP leq int_{l geq epsilon / 2}dP + int_{l< epsilon / 2} frac{epsilon}{2} dP\
&= P(L geq epsilon/2) + frac{epsilon}{2} P(L <epsilon/2)\
&= (1 - epsilon/2)P(L geq epsilon/2) + epsilon/2 < epsilon
Leftrightarrow P(L geq epsilon/2) < epsilon/(2 - epsilon)
end{align*}$
Therefore, if
$forall epsilon, forall m geq m_3:=m(epsilon/2, epsilon/(2 - epsilon)), P(L geq epsilon/2) < epsilon/(2 - epsilon)mbox{ (b)}$ holds,
$forall epsilon, forall m geq m(epsilon)=m_3, E[L] leq epsilon mbox{ (c)}$ holds too (and vice versa)
Prove $(b) Rightarrow (a)$:
Using The Fundamental Theorem of Statistical Learning for PAC learnable H with VC dimension $d$, we have:
$begin{align*}
(&epsilon/2, 1/2)mbox{-learnable H with } m_1 Leftrightarrow exists C_1 > 0, m_1 geq C_1frac{d + log(2)}{epsilon/2}\
&Leftrightarrow log(1/epsilon)(m_1 + 1/epsilon^2) geq frac{log(1/epsilon)(C_1d + C_1log(2) + 1/(2epsilon))}{epsilon/2}
end{align*}$
which uses $1/epsilon > 1$ and $log(1/epsilon) > 0$.
Now we use an inequality without proof (plot the function here)
$forall x > 1, forall d, C_1 geq 0, exists C_2 > 0, f(x)=frac{log(x)(C_1d+C_1log(2)+x/2)}{dlog(2x)+log(2x-1)} geq C_2$
Setting $x=1/epsilon$, we continue as:
$begin{align*}
&... overset{exists C_2}{geq} C_2frac{dlog(2/epsilon) + log((2-epsilon)/epsilon)}{epsilon/2} overset{exists C_3}{geq} frac{1}{C_3} m_3
Leftrightarrow (epsilon/2, epsilon/(2-epsilon))mbox{-learnable H with }m_3
end{align*}$
By setting $m_2 := C_3log(1/epsilon)(m_1 + 1/epsilon^2)$, we have $m_2 geq m_3$, thus
$begin{align*}
&forall epsilon, forall m geq m_3, P(L geq epsilon/2) < epsilon/(2 - epsilon)\
&Rightarrow forall epsilon, exists C, forall m geq m_2 := Clog(1/epsilon)(m_1 + 1/epsilon^2) geq m_3, P(L geq epsilon/2) < epsilon/(2 - epsilon)\
&Rightarrow forall epsilon, exists C, forall m geq m_2 := Clog(1/epsilon)(m_1 + 1/epsilon^2), E[L] < epsilon
end{align*}$.
Proof is complete.
New contributor
Esmailian is a new contributor to this site. Take care in asking for clarification, commenting, and answering.
Check out our Code of Conduct.
$endgroup$
add a comment |
Your Answer
StackExchange.ifUsing("editor", function () {
return StackExchange.using("mathjaxEditing", function () {
StackExchange.MarkdownEditor.creationCallbacks.add(function (editor, postfix) {
StackExchange.mathjaxEditing.prepareWmdForMathJax(editor, postfix, [["$", "$"], ["\\(","\\)"]]);
});
});
}, "mathjax-editing");
StackExchange.ready(function() {
var channelOptions = {
tags: "".split(" "),
id: "557"
};
initTagRenderer("".split(" "), "".split(" "), channelOptions);
StackExchange.using("externalEditor", function() {
// Have to fire editor after snippets, if snippets enabled
if (StackExchange.settings.snippets.snippetsEnabled) {
StackExchange.using("snippets", function() {
createEditor();
});
}
else {
createEditor();
}
});
function createEditor() {
StackExchange.prepareEditor({
heartbeatType: 'answer',
autoActivateHeartbeat: false,
convertImagesToLinks: false,
noModals: true,
showLowRepImageUploadWarning: true,
reputationToPostImages: null,
bindNavPrevention: true,
postfix: "",
imageUploader: {
brandingHtml: "Powered by u003ca class="icon-imgur-white" href="https://imgur.com/"u003eu003c/au003e",
contentPolicyHtml: "User contributions licensed under u003ca href="https://creativecommons.org/licenses/by-sa/3.0/"u003ecc by-sa 3.0 with attribution requiredu003c/au003e u003ca href="https://stackoverflow.com/legal/content-policy"u003e(content policy)u003c/au003e",
allowUrls: true
},
onDemand: true,
discardSelector: ".discard-answer"
,immediatelyShowMarkdownHelp:true
});
}
});
Nadav Schweiger is a new contributor. Be nice, and check out our Code of Conduct.
Sign up or log in
StackExchange.ready(function () {
StackExchange.helpers.onClickDraftSave('#login-link');
});
Sign up using Google
Sign up using Facebook
Sign up using Email and Password
Post as a guest
Required, but never shown
StackExchange.ready(
function () {
StackExchange.openid.initPostLogin('.new-post-login', 'https%3a%2f%2fdatascience.stackexchange.com%2fquestions%2f46638%2fa-question-on-realizable-sample-complexity%23new-answer', 'question_page');
}
);
Post as a guest
Required, but never shown
1 Answer
1
active
oldest
votes
1 Answer
1
active
oldest
votes
active
oldest
votes
active
oldest
votes
$begingroup$
We want to prove:
If H is PAC learnable, then $forall epsilon, exists C, forall m geq m_2:=Clog(1/epsilon)(m_1+1/epsilon^2), E[L] leq epsilon mbox{ (a)}$
where $m_1:=m(epsilon/2,1/2)$
Since $L leq 1$, we have $E[L] leq 1$. So proof is trivial for $epsilon geq 1$. Let $epsilon in (0, 1)$.
Find an equivalence for $(a)$:
$begin{align*}
E[L] &= int_{l geq epsilon / 2}ldP + int_{l< epsilon / 2} ldP leq int_{l geq epsilon / 2}dP + int_{l< epsilon / 2} frac{epsilon}{2} dP\
&= P(L geq epsilon/2) + frac{epsilon}{2} P(L <epsilon/2)\
&= (1 - epsilon/2)P(L geq epsilon/2) + epsilon/2 < epsilon
Leftrightarrow P(L geq epsilon/2) < epsilon/(2 - epsilon)
end{align*}$
Therefore, if
$forall epsilon, forall m geq m_3:=m(epsilon/2, epsilon/(2 - epsilon)), P(L geq epsilon/2) < epsilon/(2 - epsilon)mbox{ (b)}$ holds,
$forall epsilon, forall m geq m(epsilon)=m_3, E[L] leq epsilon mbox{ (c)}$ holds too (and vice versa)
Prove $(b) Rightarrow (a)$:
Using The Fundamental Theorem of Statistical Learning for PAC learnable H with VC dimension $d$, we have:
$begin{align*}
(&epsilon/2, 1/2)mbox{-learnable H with } m_1 Leftrightarrow exists C_1 > 0, m_1 geq C_1frac{d + log(2)}{epsilon/2}\
&Leftrightarrow log(1/epsilon)(m_1 + 1/epsilon^2) geq frac{log(1/epsilon)(C_1d + C_1log(2) + 1/(2epsilon))}{epsilon/2}
end{align*}$
which uses $1/epsilon > 1$ and $log(1/epsilon) > 0$.
Now we use an inequality without proof (plot the function here)
$forall x > 1, forall d, C_1 geq 0, exists C_2 > 0, f(x)=frac{log(x)(C_1d+C_1log(2)+x/2)}{dlog(2x)+log(2x-1)} geq C_2$
Setting $x=1/epsilon$, we continue as:
$begin{align*}
&... overset{exists C_2}{geq} C_2frac{dlog(2/epsilon) + log((2-epsilon)/epsilon)}{epsilon/2} overset{exists C_3}{geq} frac{1}{C_3} m_3
Leftrightarrow (epsilon/2, epsilon/(2-epsilon))mbox{-learnable H with }m_3
end{align*}$
By setting $m_2 := C_3log(1/epsilon)(m_1 + 1/epsilon^2)$, we have $m_2 geq m_3$, thus
$begin{align*}
&forall epsilon, forall m geq m_3, P(L geq epsilon/2) < epsilon/(2 - epsilon)\
&Rightarrow forall epsilon, exists C, forall m geq m_2 := Clog(1/epsilon)(m_1 + 1/epsilon^2) geq m_3, P(L geq epsilon/2) < epsilon/(2 - epsilon)\
&Rightarrow forall epsilon, exists C, forall m geq m_2 := Clog(1/epsilon)(m_1 + 1/epsilon^2), E[L] < epsilon
end{align*}$.
Proof is complete.
New contributor
Esmailian is a new contributor to this site. Take care in asking for clarification, commenting, and answering.
Check out our Code of Conduct.
$endgroup$
add a comment |
$begingroup$
We want to prove:
If H is PAC learnable, then $forall epsilon, exists C, forall m geq m_2:=Clog(1/epsilon)(m_1+1/epsilon^2), E[L] leq epsilon mbox{ (a)}$
where $m_1:=m(epsilon/2,1/2)$
Since $L leq 1$, we have $E[L] leq 1$. So proof is trivial for $epsilon geq 1$. Let $epsilon in (0, 1)$.
Find an equivalence for $(a)$:
$begin{align*}
E[L] &= int_{l geq epsilon / 2}ldP + int_{l< epsilon / 2} ldP leq int_{l geq epsilon / 2}dP + int_{l< epsilon / 2} frac{epsilon}{2} dP\
&= P(L geq epsilon/2) + frac{epsilon}{2} P(L <epsilon/2)\
&= (1 - epsilon/2)P(L geq epsilon/2) + epsilon/2 < epsilon
Leftrightarrow P(L geq epsilon/2) < epsilon/(2 - epsilon)
end{align*}$
Therefore, if
$forall epsilon, forall m geq m_3:=m(epsilon/2, epsilon/(2 - epsilon)), P(L geq epsilon/2) < epsilon/(2 - epsilon)mbox{ (b)}$ holds,
$forall epsilon, forall m geq m(epsilon)=m_3, E[L] leq epsilon mbox{ (c)}$ holds too (and vice versa)
Prove $(b) Rightarrow (a)$:
Using The Fundamental Theorem of Statistical Learning for PAC learnable H with VC dimension $d$, we have:
$begin{align*}
(&epsilon/2, 1/2)mbox{-learnable H with } m_1 Leftrightarrow exists C_1 > 0, m_1 geq C_1frac{d + log(2)}{epsilon/2}\
&Leftrightarrow log(1/epsilon)(m_1 + 1/epsilon^2) geq frac{log(1/epsilon)(C_1d + C_1log(2) + 1/(2epsilon))}{epsilon/2}
end{align*}$
which uses $1/epsilon > 1$ and $log(1/epsilon) > 0$.
Now we use an inequality without proof (plot the function here)
$forall x > 1, forall d, C_1 geq 0, exists C_2 > 0, f(x)=frac{log(x)(C_1d+C_1log(2)+x/2)}{dlog(2x)+log(2x-1)} geq C_2$
Setting $x=1/epsilon$, we continue as:
$begin{align*}
&... overset{exists C_2}{geq} C_2frac{dlog(2/epsilon) + log((2-epsilon)/epsilon)}{epsilon/2} overset{exists C_3}{geq} frac{1}{C_3} m_3
Leftrightarrow (epsilon/2, epsilon/(2-epsilon))mbox{-learnable H with }m_3
end{align*}$
By setting $m_2 := C_3log(1/epsilon)(m_1 + 1/epsilon^2)$, we have $m_2 geq m_3$, thus
$begin{align*}
&forall epsilon, forall m geq m_3, P(L geq epsilon/2) < epsilon/(2 - epsilon)\
&Rightarrow forall epsilon, exists C, forall m geq m_2 := Clog(1/epsilon)(m_1 + 1/epsilon^2) geq m_3, P(L geq epsilon/2) < epsilon/(2 - epsilon)\
&Rightarrow forall epsilon, exists C, forall m geq m_2 := Clog(1/epsilon)(m_1 + 1/epsilon^2), E[L] < epsilon
end{align*}$.
Proof is complete.
New contributor
Esmailian is a new contributor to this site. Take care in asking for clarification, commenting, and answering.
Check out our Code of Conduct.
$endgroup$
add a comment |
$begingroup$
We want to prove:
If H is PAC learnable, then $forall epsilon, exists C, forall m geq m_2:=Clog(1/epsilon)(m_1+1/epsilon^2), E[L] leq epsilon mbox{ (a)}$
where $m_1:=m(epsilon/2,1/2)$
Since $L leq 1$, we have $E[L] leq 1$. So proof is trivial for $epsilon geq 1$. Let $epsilon in (0, 1)$.
Find an equivalence for $(a)$:
$begin{align*}
E[L] &= int_{l geq epsilon / 2}ldP + int_{l< epsilon / 2} ldP leq int_{l geq epsilon / 2}dP + int_{l< epsilon / 2} frac{epsilon}{2} dP\
&= P(L geq epsilon/2) + frac{epsilon}{2} P(L <epsilon/2)\
&= (1 - epsilon/2)P(L geq epsilon/2) + epsilon/2 < epsilon
Leftrightarrow P(L geq epsilon/2) < epsilon/(2 - epsilon)
end{align*}$
Therefore, if
$forall epsilon, forall m geq m_3:=m(epsilon/2, epsilon/(2 - epsilon)), P(L geq epsilon/2) < epsilon/(2 - epsilon)mbox{ (b)}$ holds,
$forall epsilon, forall m geq m(epsilon)=m_3, E[L] leq epsilon mbox{ (c)}$ holds too (and vice versa)
Prove $(b) Rightarrow (a)$:
Using The Fundamental Theorem of Statistical Learning for PAC learnable H with VC dimension $d$, we have:
$begin{align*}
(&epsilon/2, 1/2)mbox{-learnable H with } m_1 Leftrightarrow exists C_1 > 0, m_1 geq C_1frac{d + log(2)}{epsilon/2}\
&Leftrightarrow log(1/epsilon)(m_1 + 1/epsilon^2) geq frac{log(1/epsilon)(C_1d + C_1log(2) + 1/(2epsilon))}{epsilon/2}
end{align*}$
which uses $1/epsilon > 1$ and $log(1/epsilon) > 0$.
Now we use an inequality without proof (plot the function here)
$forall x > 1, forall d, C_1 geq 0, exists C_2 > 0, f(x)=frac{log(x)(C_1d+C_1log(2)+x/2)}{dlog(2x)+log(2x-1)} geq C_2$
Setting $x=1/epsilon$, we continue as:
$begin{align*}
&... overset{exists C_2}{geq} C_2frac{dlog(2/epsilon) + log((2-epsilon)/epsilon)}{epsilon/2} overset{exists C_3}{geq} frac{1}{C_3} m_3
Leftrightarrow (epsilon/2, epsilon/(2-epsilon))mbox{-learnable H with }m_3
end{align*}$
By setting $m_2 := C_3log(1/epsilon)(m_1 + 1/epsilon^2)$, we have $m_2 geq m_3$, thus
$begin{align*}
&forall epsilon, forall m geq m_3, P(L geq epsilon/2) < epsilon/(2 - epsilon)\
&Rightarrow forall epsilon, exists C, forall m geq m_2 := Clog(1/epsilon)(m_1 + 1/epsilon^2) geq m_3, P(L geq epsilon/2) < epsilon/(2 - epsilon)\
&Rightarrow forall epsilon, exists C, forall m geq m_2 := Clog(1/epsilon)(m_1 + 1/epsilon^2), E[L] < epsilon
end{align*}$.
Proof is complete.
New contributor
Esmailian is a new contributor to this site. Take care in asking for clarification, commenting, and answering.
Check out our Code of Conduct.
$endgroup$
We want to prove:
If H is PAC learnable, then $forall epsilon, exists C, forall m geq m_2:=Clog(1/epsilon)(m_1+1/epsilon^2), E[L] leq epsilon mbox{ (a)}$
where $m_1:=m(epsilon/2,1/2)$
Since $L leq 1$, we have $E[L] leq 1$. So proof is trivial for $epsilon geq 1$. Let $epsilon in (0, 1)$.
Find an equivalence for $(a)$:
$begin{align*}
E[L] &= int_{l geq epsilon / 2}ldP + int_{l< epsilon / 2} ldP leq int_{l geq epsilon / 2}dP + int_{l< epsilon / 2} frac{epsilon}{2} dP\
&= P(L geq epsilon/2) + frac{epsilon}{2} P(L <epsilon/2)\
&= (1 - epsilon/2)P(L geq epsilon/2) + epsilon/2 < epsilon
Leftrightarrow P(L geq epsilon/2) < epsilon/(2 - epsilon)
end{align*}$
Therefore, if
$forall epsilon, forall m geq m_3:=m(epsilon/2, epsilon/(2 - epsilon)), P(L geq epsilon/2) < epsilon/(2 - epsilon)mbox{ (b)}$ holds,
$forall epsilon, forall m geq m(epsilon)=m_3, E[L] leq epsilon mbox{ (c)}$ holds too (and vice versa)
Prove $(b) Rightarrow (a)$:
Using The Fundamental Theorem of Statistical Learning for PAC learnable H with VC dimension $d$, we have:
$begin{align*}
(&epsilon/2, 1/2)mbox{-learnable H with } m_1 Leftrightarrow exists C_1 > 0, m_1 geq C_1frac{d + log(2)}{epsilon/2}\
&Leftrightarrow log(1/epsilon)(m_1 + 1/epsilon^2) geq frac{log(1/epsilon)(C_1d + C_1log(2) + 1/(2epsilon))}{epsilon/2}
end{align*}$
which uses $1/epsilon > 1$ and $log(1/epsilon) > 0$.
Now we use an inequality without proof (plot the function here)
$forall x > 1, forall d, C_1 geq 0, exists C_2 > 0, f(x)=frac{log(x)(C_1d+C_1log(2)+x/2)}{dlog(2x)+log(2x-1)} geq C_2$
Setting $x=1/epsilon$, we continue as:
$begin{align*}
&... overset{exists C_2}{geq} C_2frac{dlog(2/epsilon) + log((2-epsilon)/epsilon)}{epsilon/2} overset{exists C_3}{geq} frac{1}{C_3} m_3
Leftrightarrow (epsilon/2, epsilon/(2-epsilon))mbox{-learnable H with }m_3
end{align*}$
By setting $m_2 := C_3log(1/epsilon)(m_1 + 1/epsilon^2)$, we have $m_2 geq m_3$, thus
$begin{align*}
&forall epsilon, forall m geq m_3, P(L geq epsilon/2) < epsilon/(2 - epsilon)\
&Rightarrow forall epsilon, exists C, forall m geq m_2 := Clog(1/epsilon)(m_1 + 1/epsilon^2) geq m_3, P(L geq epsilon/2) < epsilon/(2 - epsilon)\
&Rightarrow forall epsilon, exists C, forall m geq m_2 := Clog(1/epsilon)(m_1 + 1/epsilon^2), E[L] < epsilon
end{align*}$.
Proof is complete.
New contributor
Esmailian is a new contributor to this site. Take care in asking for clarification, commenting, and answering.
Check out our Code of Conduct.
edited yesterday
New contributor
Esmailian is a new contributor to this site. Take care in asking for clarification, commenting, and answering.
Check out our Code of Conduct.
answered 2 days ago
EsmailianEsmailian
1764
1764
New contributor
Esmailian is a new contributor to this site. Take care in asking for clarification, commenting, and answering.
Check out our Code of Conduct.
New contributor
Esmailian is a new contributor to this site. Take care in asking for clarification, commenting, and answering.
Check out our Code of Conduct.
Esmailian is a new contributor to this site. Take care in asking for clarification, commenting, and answering.
Check out our Code of Conduct.
add a comment |
add a comment |
Nadav Schweiger is a new contributor. Be nice, and check out our Code of Conduct.
Nadav Schweiger is a new contributor. Be nice, and check out our Code of Conduct.
Nadav Schweiger is a new contributor. Be nice, and check out our Code of Conduct.
Nadav Schweiger is a new contributor. Be nice, and check out our Code of Conduct.
Thanks for contributing an answer to Data Science Stack Exchange!
- Please be sure to answer the question. Provide details and share your research!
But avoid …
- Asking for help, clarification, or responding to other answers.
- Making statements based on opinion; back them up with references or personal experience.
Use MathJax to format equations. MathJax reference.
To learn more, see our tips on writing great answers.
Sign up or log in
StackExchange.ready(function () {
StackExchange.helpers.onClickDraftSave('#login-link');
});
Sign up using Google
Sign up using Facebook
Sign up using Email and Password
Post as a guest
Required, but never shown
StackExchange.ready(
function () {
StackExchange.openid.initPostLogin('.new-post-login', 'https%3a%2f%2fdatascience.stackexchange.com%2fquestions%2f46638%2fa-question-on-realizable-sample-complexity%23new-answer', 'question_page');
}
);
Post as a guest
Required, but never shown
Sign up or log in
StackExchange.ready(function () {
StackExchange.helpers.onClickDraftSave('#login-link');
});
Sign up using Google
Sign up using Facebook
Sign up using Email and Password
Post as a guest
Required, but never shown
Sign up or log in
StackExchange.ready(function () {
StackExchange.helpers.onClickDraftSave('#login-link');
});
Sign up using Google
Sign up using Facebook
Sign up using Email and Password
Post as a guest
Required, but never shown
Sign up or log in
StackExchange.ready(function () {
StackExchange.helpers.onClickDraftSave('#login-link');
});
Sign up using Google
Sign up using Facebook
Sign up using Email and Password
Sign up using Google
Sign up using Facebook
Sign up using Email and Password
Post as a guest
Required, but never shown
Required, but never shown
Required, but never shown
Required, but never shown
Required, but never shown
Required, but never shown
Required, but never shown
Required, but never shown
Required, but never shown
I1j6D9Pzbvt0A,bkibUflx6rDjo