PyMC3: how to efficiently regress on many variables?
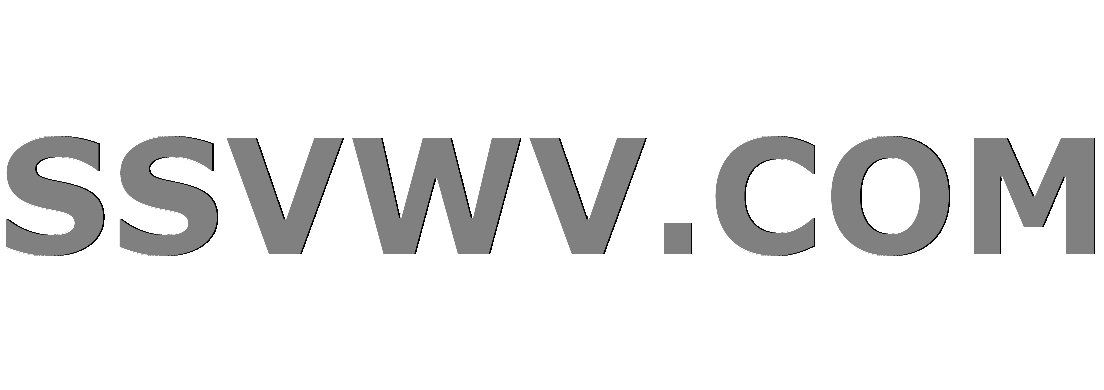
Multi tool use
$begingroup$
I am sorry ahead of time if this seems like a basic question, but I had difficulty finding resources online addressing this. In PyMC3, when building a basic model of a few variables, it is easy to define each of them, like alpha=pm.Normal('alpha',mu=0,st=1)
, and manually add them all with each other. However, what are the standard approaches when one is dealing with dozens/hundreds of variables, each needing a prior?
I see that the shape
argument is helpful in defining many priors at once, but after that how do you then add them all together in the mean equation (if your model specifies a Normal distribution), or what if different groups of variables need different priors?
I would heavily appreciate any advice on this matter, as I'm switching over to PyMC3 from regular machine learning and regression.
python bigdata data bayesian
$endgroup$
add a comment |
$begingroup$
I am sorry ahead of time if this seems like a basic question, but I had difficulty finding resources online addressing this. In PyMC3, when building a basic model of a few variables, it is easy to define each of them, like alpha=pm.Normal('alpha',mu=0,st=1)
, and manually add them all with each other. However, what are the standard approaches when one is dealing with dozens/hundreds of variables, each needing a prior?
I see that the shape
argument is helpful in defining many priors at once, but after that how do you then add them all together in the mean equation (if your model specifies a Normal distribution), or what if different groups of variables need different priors?
I would heavily appreciate any advice on this matter, as I'm switching over to PyMC3 from regular machine learning and regression.
python bigdata data bayesian
$endgroup$
add a comment |
$begingroup$
I am sorry ahead of time if this seems like a basic question, but I had difficulty finding resources online addressing this. In PyMC3, when building a basic model of a few variables, it is easy to define each of them, like alpha=pm.Normal('alpha',mu=0,st=1)
, and manually add them all with each other. However, what are the standard approaches when one is dealing with dozens/hundreds of variables, each needing a prior?
I see that the shape
argument is helpful in defining many priors at once, but after that how do you then add them all together in the mean equation (if your model specifies a Normal distribution), or what if different groups of variables need different priors?
I would heavily appreciate any advice on this matter, as I'm switching over to PyMC3 from regular machine learning and regression.
python bigdata data bayesian
$endgroup$
I am sorry ahead of time if this seems like a basic question, but I had difficulty finding resources online addressing this. In PyMC3, when building a basic model of a few variables, it is easy to define each of them, like alpha=pm.Normal('alpha',mu=0,st=1)
, and manually add them all with each other. However, what are the standard approaches when one is dealing with dozens/hundreds of variables, each needing a prior?
I see that the shape
argument is helpful in defining many priors at once, but after that how do you then add them all together in the mean equation (if your model specifies a Normal distribution), or what if different groups of variables need different priors?
I would heavily appreciate any advice on this matter, as I'm switching over to PyMC3 from regular machine learning and regression.
python bigdata data bayesian
python bigdata data bayesian
asked 1 hour ago
Coolio2654Coolio2654
1183
1183
add a comment |
add a comment |
0
active
oldest
votes
Your Answer
StackExchange.ifUsing("editor", function () {
return StackExchange.using("mathjaxEditing", function () {
StackExchange.MarkdownEditor.creationCallbacks.add(function (editor, postfix) {
StackExchange.mathjaxEditing.prepareWmdForMathJax(editor, postfix, [["$", "$"], ["\\(","\\)"]]);
});
});
}, "mathjax-editing");
StackExchange.ready(function() {
var channelOptions = {
tags: "".split(" "),
id: "557"
};
initTagRenderer("".split(" "), "".split(" "), channelOptions);
StackExchange.using("externalEditor", function() {
// Have to fire editor after snippets, if snippets enabled
if (StackExchange.settings.snippets.snippetsEnabled) {
StackExchange.using("snippets", function() {
createEditor();
});
}
else {
createEditor();
}
});
function createEditor() {
StackExchange.prepareEditor({
heartbeatType: 'answer',
autoActivateHeartbeat: false,
convertImagesToLinks: false,
noModals: true,
showLowRepImageUploadWarning: true,
reputationToPostImages: null,
bindNavPrevention: true,
postfix: "",
imageUploader: {
brandingHtml: "Powered by u003ca class="icon-imgur-white" href="https://imgur.com/"u003eu003c/au003e",
contentPolicyHtml: "User contributions licensed under u003ca href="https://creativecommons.org/licenses/by-sa/3.0/"u003ecc by-sa 3.0 with attribution requiredu003c/au003e u003ca href="https://stackoverflow.com/legal/content-policy"u003e(content policy)u003c/au003e",
allowUrls: true
},
onDemand: true,
discardSelector: ".discard-answer"
,immediatelyShowMarkdownHelp:true
});
}
});
Sign up or log in
StackExchange.ready(function () {
StackExchange.helpers.onClickDraftSave('#login-link');
});
Sign up using Google
Sign up using Facebook
Sign up using Email and Password
Post as a guest
Required, but never shown
StackExchange.ready(
function () {
StackExchange.openid.initPostLogin('.new-post-login', 'https%3a%2f%2fdatascience.stackexchange.com%2fquestions%2f44313%2fpymc3-how-to-efficiently-regress-on-many-variables%23new-answer', 'question_page');
}
);
Post as a guest
Required, but never shown
0
active
oldest
votes
0
active
oldest
votes
active
oldest
votes
active
oldest
votes
Thanks for contributing an answer to Data Science Stack Exchange!
- Please be sure to answer the question. Provide details and share your research!
But avoid …
- Asking for help, clarification, or responding to other answers.
- Making statements based on opinion; back them up with references or personal experience.
Use MathJax to format equations. MathJax reference.
To learn more, see our tips on writing great answers.
Sign up or log in
StackExchange.ready(function () {
StackExchange.helpers.onClickDraftSave('#login-link');
});
Sign up using Google
Sign up using Facebook
Sign up using Email and Password
Post as a guest
Required, but never shown
StackExchange.ready(
function () {
StackExchange.openid.initPostLogin('.new-post-login', 'https%3a%2f%2fdatascience.stackexchange.com%2fquestions%2f44313%2fpymc3-how-to-efficiently-regress-on-many-variables%23new-answer', 'question_page');
}
);
Post as a guest
Required, but never shown
Sign up or log in
StackExchange.ready(function () {
StackExchange.helpers.onClickDraftSave('#login-link');
});
Sign up using Google
Sign up using Facebook
Sign up using Email and Password
Post as a guest
Required, but never shown
Sign up or log in
StackExchange.ready(function () {
StackExchange.helpers.onClickDraftSave('#login-link');
});
Sign up using Google
Sign up using Facebook
Sign up using Email and Password
Post as a guest
Required, but never shown
Sign up or log in
StackExchange.ready(function () {
StackExchange.helpers.onClickDraftSave('#login-link');
});
Sign up using Google
Sign up using Facebook
Sign up using Email and Password
Sign up using Google
Sign up using Facebook
Sign up using Email and Password
Post as a guest
Required, but never shown
Required, but never shown
Required, but never shown
Required, but never shown
Required, but never shown
Required, but never shown
Required, but never shown
Required, but never shown
Required, but never shown
xxBHCdxdawAEMQuEpIGUjM,7Z lGf,j Y5e