Multioutput classification in Keras - how to get multivariate probabilities and deal with unseen classes
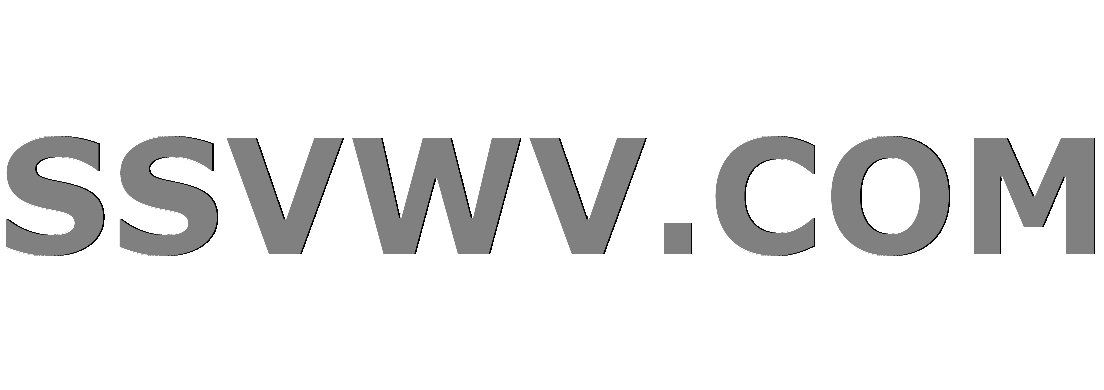
Multi tool use
$begingroup$
I'm struggling to design in Keras a deep neural network for multioutput classification model. The network works in tandem with external logic in a kind of feedback loop: in each iteration the external module generates the training set, on which the network is trained and then in next iteration the network supports the module in another round of training set generation. I'm rather at rookie+ level and I have encountered 2 problems I do not know how to deal with:
1) how to make a network predict vectors that will not show up in training set, but still account for underlying correlations between vector components
2) how to yield a multivariate distribution on multioutput space.
To be more specific, the "ground truth" of the model is $N$-length vector of binary labels: $y in { 0, 1}^{N} $. This represents some yes/no $N$-dimensional decision and is generated by the external module. The key issue is the yes/no components are highly correlated, $N$ maybe large and the groud truth is not "deterministic" - i.e. it's just some approximation that is expected to get better over the feedback learning process described above.
So far I have designed a simple classification network based on Keras sequence model with $N$ output layer units.
Restating my problems in more specific way:
ad 1) there are $2^{N}$ possible output vectors ("metaclasses"). As $N$ is large, almost surely not all of them will appear in my training set. But I want my network to be flexible enough to be able to come up with unseen metaclasses but still take care of high correlations between single yes/no components.
ad 2) I would like the network to output a few (say three) most probable vectors. In other words, I would like it to yield a (obviously some cut-off) distribution over vectors.
keras multilabel-classification
New contributor
micsza is a new contributor to this site. Take care in asking for clarification, commenting, and answering.
Check out our Code of Conduct.
$endgroup$
add a comment |
$begingroup$
I'm struggling to design in Keras a deep neural network for multioutput classification model. The network works in tandem with external logic in a kind of feedback loop: in each iteration the external module generates the training set, on which the network is trained and then in next iteration the network supports the module in another round of training set generation. I'm rather at rookie+ level and I have encountered 2 problems I do not know how to deal with:
1) how to make a network predict vectors that will not show up in training set, but still account for underlying correlations between vector components
2) how to yield a multivariate distribution on multioutput space.
To be more specific, the "ground truth" of the model is $N$-length vector of binary labels: $y in { 0, 1}^{N} $. This represents some yes/no $N$-dimensional decision and is generated by the external module. The key issue is the yes/no components are highly correlated, $N$ maybe large and the groud truth is not "deterministic" - i.e. it's just some approximation that is expected to get better over the feedback learning process described above.
So far I have designed a simple classification network based on Keras sequence model with $N$ output layer units.
Restating my problems in more specific way:
ad 1) there are $2^{N}$ possible output vectors ("metaclasses"). As $N$ is large, almost surely not all of them will appear in my training set. But I want my network to be flexible enough to be able to come up with unseen metaclasses but still take care of high correlations between single yes/no components.
ad 2) I would like the network to output a few (say three) most probable vectors. In other words, I would like it to yield a (obviously some cut-off) distribution over vectors.
keras multilabel-classification
New contributor
micsza is a new contributor to this site. Take care in asking for clarification, commenting, and answering.
Check out our Code of Conduct.
$endgroup$
add a comment |
$begingroup$
I'm struggling to design in Keras a deep neural network for multioutput classification model. The network works in tandem with external logic in a kind of feedback loop: in each iteration the external module generates the training set, on which the network is trained and then in next iteration the network supports the module in another round of training set generation. I'm rather at rookie+ level and I have encountered 2 problems I do not know how to deal with:
1) how to make a network predict vectors that will not show up in training set, but still account for underlying correlations between vector components
2) how to yield a multivariate distribution on multioutput space.
To be more specific, the "ground truth" of the model is $N$-length vector of binary labels: $y in { 0, 1}^{N} $. This represents some yes/no $N$-dimensional decision and is generated by the external module. The key issue is the yes/no components are highly correlated, $N$ maybe large and the groud truth is not "deterministic" - i.e. it's just some approximation that is expected to get better over the feedback learning process described above.
So far I have designed a simple classification network based on Keras sequence model with $N$ output layer units.
Restating my problems in more specific way:
ad 1) there are $2^{N}$ possible output vectors ("metaclasses"). As $N$ is large, almost surely not all of them will appear in my training set. But I want my network to be flexible enough to be able to come up with unseen metaclasses but still take care of high correlations between single yes/no components.
ad 2) I would like the network to output a few (say three) most probable vectors. In other words, I would like it to yield a (obviously some cut-off) distribution over vectors.
keras multilabel-classification
New contributor
micsza is a new contributor to this site. Take care in asking for clarification, commenting, and answering.
Check out our Code of Conduct.
$endgroup$
I'm struggling to design in Keras a deep neural network for multioutput classification model. The network works in tandem with external logic in a kind of feedback loop: in each iteration the external module generates the training set, on which the network is trained and then in next iteration the network supports the module in another round of training set generation. I'm rather at rookie+ level and I have encountered 2 problems I do not know how to deal with:
1) how to make a network predict vectors that will not show up in training set, but still account for underlying correlations between vector components
2) how to yield a multivariate distribution on multioutput space.
To be more specific, the "ground truth" of the model is $N$-length vector of binary labels: $y in { 0, 1}^{N} $. This represents some yes/no $N$-dimensional decision and is generated by the external module. The key issue is the yes/no components are highly correlated, $N$ maybe large and the groud truth is not "deterministic" - i.e. it's just some approximation that is expected to get better over the feedback learning process described above.
So far I have designed a simple classification network based on Keras sequence model with $N$ output layer units.
Restating my problems in more specific way:
ad 1) there are $2^{N}$ possible output vectors ("metaclasses"). As $N$ is large, almost surely not all of them will appear in my training set. But I want my network to be flexible enough to be able to come up with unseen metaclasses but still take care of high correlations between single yes/no components.
ad 2) I would like the network to output a few (say three) most probable vectors. In other words, I would like it to yield a (obviously some cut-off) distribution over vectors.
keras multilabel-classification
keras multilabel-classification
New contributor
micsza is a new contributor to this site. Take care in asking for clarification, commenting, and answering.
Check out our Code of Conduct.
New contributor
micsza is a new contributor to this site. Take care in asking for clarification, commenting, and answering.
Check out our Code of Conduct.
New contributor
micsza is a new contributor to this site. Take care in asking for clarification, commenting, and answering.
Check out our Code of Conduct.
asked 14 hours ago
micszamicsza
61
61
New contributor
micsza is a new contributor to this site. Take care in asking for clarification, commenting, and answering.
Check out our Code of Conduct.
New contributor
micsza is a new contributor to this site. Take care in asking for clarification, commenting, and answering.
Check out our Code of Conduct.
micsza is a new contributor to this site. Take care in asking for clarification, commenting, and answering.
Check out our Code of Conduct.
add a comment |
add a comment |
0
active
oldest
votes
Your Answer
StackExchange.ifUsing("editor", function () {
return StackExchange.using("mathjaxEditing", function () {
StackExchange.MarkdownEditor.creationCallbacks.add(function (editor, postfix) {
StackExchange.mathjaxEditing.prepareWmdForMathJax(editor, postfix, [["$", "$"], ["\\(","\\)"]]);
});
});
}, "mathjax-editing");
StackExchange.ready(function() {
var channelOptions = {
tags: "".split(" "),
id: "557"
};
initTagRenderer("".split(" "), "".split(" "), channelOptions);
StackExchange.using("externalEditor", function() {
// Have to fire editor after snippets, if snippets enabled
if (StackExchange.settings.snippets.snippetsEnabled) {
StackExchange.using("snippets", function() {
createEditor();
});
}
else {
createEditor();
}
});
function createEditor() {
StackExchange.prepareEditor({
heartbeatType: 'answer',
autoActivateHeartbeat: false,
convertImagesToLinks: false,
noModals: true,
showLowRepImageUploadWarning: true,
reputationToPostImages: null,
bindNavPrevention: true,
postfix: "",
imageUploader: {
brandingHtml: "Powered by u003ca class="icon-imgur-white" href="https://imgur.com/"u003eu003c/au003e",
contentPolicyHtml: "User contributions licensed under u003ca href="https://creativecommons.org/licenses/by-sa/3.0/"u003ecc by-sa 3.0 with attribution requiredu003c/au003e u003ca href="https://stackoverflow.com/legal/content-policy"u003e(content policy)u003c/au003e",
allowUrls: true
},
onDemand: true,
discardSelector: ".discard-answer"
,immediatelyShowMarkdownHelp:true
});
}
});
micsza is a new contributor. Be nice, and check out our Code of Conduct.
Sign up or log in
StackExchange.ready(function () {
StackExchange.helpers.onClickDraftSave('#login-link');
});
Sign up using Google
Sign up using Facebook
Sign up using Email and Password
Post as a guest
Required, but never shown
StackExchange.ready(
function () {
StackExchange.openid.initPostLogin('.new-post-login', 'https%3a%2f%2fdatascience.stackexchange.com%2fquestions%2f45312%2fmultioutput-classification-in-keras-how-to-get-multivariate-probabilities-and%23new-answer', 'question_page');
}
);
Post as a guest
Required, but never shown
0
active
oldest
votes
0
active
oldest
votes
active
oldest
votes
active
oldest
votes
micsza is a new contributor. Be nice, and check out our Code of Conduct.
micsza is a new contributor. Be nice, and check out our Code of Conduct.
micsza is a new contributor. Be nice, and check out our Code of Conduct.
micsza is a new contributor. Be nice, and check out our Code of Conduct.
Thanks for contributing an answer to Data Science Stack Exchange!
- Please be sure to answer the question. Provide details and share your research!
But avoid …
- Asking for help, clarification, or responding to other answers.
- Making statements based on opinion; back them up with references or personal experience.
Use MathJax to format equations. MathJax reference.
To learn more, see our tips on writing great answers.
Sign up or log in
StackExchange.ready(function () {
StackExchange.helpers.onClickDraftSave('#login-link');
});
Sign up using Google
Sign up using Facebook
Sign up using Email and Password
Post as a guest
Required, but never shown
StackExchange.ready(
function () {
StackExchange.openid.initPostLogin('.new-post-login', 'https%3a%2f%2fdatascience.stackexchange.com%2fquestions%2f45312%2fmultioutput-classification-in-keras-how-to-get-multivariate-probabilities-and%23new-answer', 'question_page');
}
);
Post as a guest
Required, but never shown
Sign up or log in
StackExchange.ready(function () {
StackExchange.helpers.onClickDraftSave('#login-link');
});
Sign up using Google
Sign up using Facebook
Sign up using Email and Password
Post as a guest
Required, but never shown
Sign up or log in
StackExchange.ready(function () {
StackExchange.helpers.onClickDraftSave('#login-link');
});
Sign up using Google
Sign up using Facebook
Sign up using Email and Password
Post as a guest
Required, but never shown
Sign up or log in
StackExchange.ready(function () {
StackExchange.helpers.onClickDraftSave('#login-link');
});
Sign up using Google
Sign up using Facebook
Sign up using Email and Password
Sign up using Google
Sign up using Facebook
Sign up using Email and Password
Post as a guest
Required, but never shown
Required, but never shown
Required, but never shown
Required, but never shown
Required, but never shown
Required, but never shown
Required, but never shown
Required, but never shown
Required, but never shown
bD,N,AYudoK,Y INc,eU44 kRxBAOljr