using unsupervised learning algorithms on images
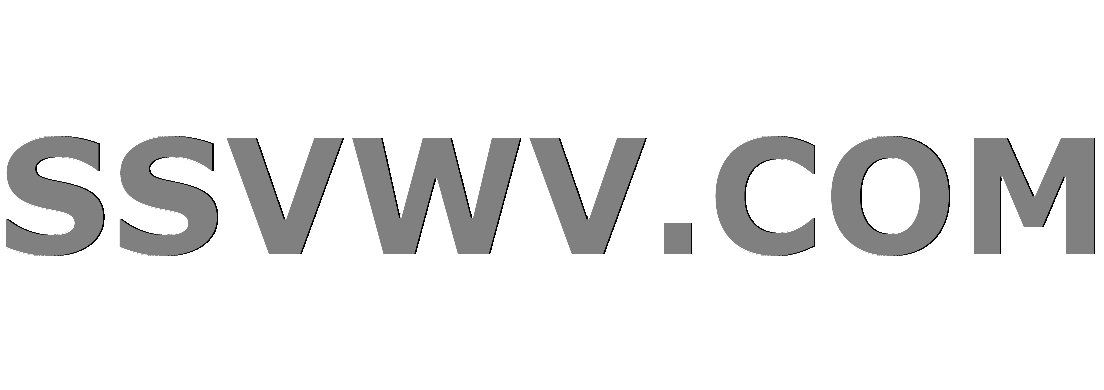
Multi tool use
$begingroup$
I am working on a project to classify images of types of cloth (shirt, tshirt, pant etc). While this is a standard supervised classification problem, the accuracy of the neural network is not good. This is because of the close similarity of the types of cloth that I am trying to classify.
I am working with 9 classes with around 10,000 images per class. For the classification problem I tried using CNN to classify the images. But over fitting took place with a good training accuracy (around 95%), but not so great validation accuracy (around 77%).
I wanted to know if there was any way I could create clusters based on the type of cloth using some unsupervised learning algorithm like K Means or DBScan.
python neural-network unsupervised-learning
$endgroup$
bumped to the homepage by Community♦ yesterday
This question has answers that may be good or bad; the system has marked it active so that they can be reviewed.
add a comment |
$begingroup$
I am working on a project to classify images of types of cloth (shirt, tshirt, pant etc). While this is a standard supervised classification problem, the accuracy of the neural network is not good. This is because of the close similarity of the types of cloth that I am trying to classify.
I am working with 9 classes with around 10,000 images per class. For the classification problem I tried using CNN to classify the images. But over fitting took place with a good training accuracy (around 95%), but not so great validation accuracy (around 77%).
I wanted to know if there was any way I could create clusters based on the type of cloth using some unsupervised learning algorithm like K Means or DBScan.
python neural-network unsupervised-learning
$endgroup$
bumped to the homepage by Community♦ yesterday
This question has answers that may be good or bad; the system has marked it active so that they can be reviewed.
$begingroup$
Did you try data augmentation (rotating your images....)
$endgroup$
– Robin Nicole
Dec 12 '18 at 20:04
$begingroup$
Unsupervised learning is not going to perform better than a well trained CNN for so many images. You should reduce overfitting on your CNN. For example try a smaller model, or Data Augmentation, or adding dropout, or tuning batchsize/learningrate. Or use a pretrained model that you finetune
$endgroup$
– jonnor
yesterday
add a comment |
$begingroup$
I am working on a project to classify images of types of cloth (shirt, tshirt, pant etc). While this is a standard supervised classification problem, the accuracy of the neural network is not good. This is because of the close similarity of the types of cloth that I am trying to classify.
I am working with 9 classes with around 10,000 images per class. For the classification problem I tried using CNN to classify the images. But over fitting took place with a good training accuracy (around 95%), but not so great validation accuracy (around 77%).
I wanted to know if there was any way I could create clusters based on the type of cloth using some unsupervised learning algorithm like K Means or DBScan.
python neural-network unsupervised-learning
$endgroup$
I am working on a project to classify images of types of cloth (shirt, tshirt, pant etc). While this is a standard supervised classification problem, the accuracy of the neural network is not good. This is because of the close similarity of the types of cloth that I am trying to classify.
I am working with 9 classes with around 10,000 images per class. For the classification problem I tried using CNN to classify the images. But over fitting took place with a good training accuracy (around 95%), but not so great validation accuracy (around 77%).
I wanted to know if there was any way I could create clusters based on the type of cloth using some unsupervised learning algorithm like K Means or DBScan.
python neural-network unsupervised-learning
python neural-network unsupervised-learning
edited Aug 14 '18 at 10:22


Stephen Rauch♦
1,52551330
1,52551330
asked Aug 14 '18 at 4:21
SashaankSashaank
1
1
bumped to the homepage by Community♦ yesterday
This question has answers that may be good or bad; the system has marked it active so that they can be reviewed.
bumped to the homepage by Community♦ yesterday
This question has answers that may be good or bad; the system has marked it active so that they can be reviewed.
$begingroup$
Did you try data augmentation (rotating your images....)
$endgroup$
– Robin Nicole
Dec 12 '18 at 20:04
$begingroup$
Unsupervised learning is not going to perform better than a well trained CNN for so many images. You should reduce overfitting on your CNN. For example try a smaller model, or Data Augmentation, or adding dropout, or tuning batchsize/learningrate. Or use a pretrained model that you finetune
$endgroup$
– jonnor
yesterday
add a comment |
$begingroup$
Did you try data augmentation (rotating your images....)
$endgroup$
– Robin Nicole
Dec 12 '18 at 20:04
$begingroup$
Unsupervised learning is not going to perform better than a well trained CNN for so many images. You should reduce overfitting on your CNN. For example try a smaller model, or Data Augmentation, or adding dropout, or tuning batchsize/learningrate. Or use a pretrained model that you finetune
$endgroup$
– jonnor
yesterday
$begingroup$
Did you try data augmentation (rotating your images....)
$endgroup$
– Robin Nicole
Dec 12 '18 at 20:04
$begingroup$
Did you try data augmentation (rotating your images....)
$endgroup$
– Robin Nicole
Dec 12 '18 at 20:04
$begingroup$
Unsupervised learning is not going to perform better than a well trained CNN for so many images. You should reduce overfitting on your CNN. For example try a smaller model, or Data Augmentation, or adding dropout, or tuning batchsize/learningrate. Or use a pretrained model that you finetune
$endgroup$
– jonnor
yesterday
$begingroup$
Unsupervised learning is not going to perform better than a well trained CNN for so many images. You should reduce overfitting on your CNN. For example try a smaller model, or Data Augmentation, or adding dropout, or tuning batchsize/learningrate. Or use a pretrained model that you finetune
$endgroup$
– jonnor
yesterday
add a comment |
1 Answer
1
active
oldest
votes
$begingroup$
Have you included dropout in your model? It can help avoid overfitting issue.
For your problem, yes, you can use auto-encoders, GAN, etc. for feature learning.
However, I'm not sure if unsupervised learning can help, since it's more like a training issue. Your have label with your data so supervised learning is ideal, plus supervised learning generally shows better performance than unsupervised in image classification. You might want to check the false classification examples in your dataset, and try to alter the CNN structure based on that, which would be a more direct way.
$endgroup$
$begingroup$
Yes I have used dropout for my network. but That does not seem to have much effect. The problem is if you are familiar with Indian clothing (kurta is very similar to salwar) And since my dataset comprises of both the types of clothing, the program does not work well. should i try increasing the data size though i dod not know if that will have that big of an impact
$endgroup$
– Sashaank
Aug 14 '18 at 6:07
$begingroup$
I checked google for them, it seems the main difference is the shape. CNN should be able to recognize such difference. Usually I will try to take the data for these two label out and train CNN for them only, and then see if can classify between them. If true, it means the degradation of model is caused by the introduction of multi-class classification. Otherwise, it's simply caused by the model structure, and you might want to work on that.
$endgroup$
– plpopk
Aug 14 '18 at 7:00
$begingroup$
I will try that. thanks. Any idea on how to deal with multi classes?
$endgroup$
– Sashaank
Aug 14 '18 at 7:54
$begingroup$
Check if you used softmax activation. At the moment, what come to my mind is either adjust the cost function or add extra models (e.g. combine with a binary classification model which works well).
$endgroup$
– plpopk
Aug 14 '18 at 8:32
add a comment |
Your Answer
StackExchange.ready(function() {
var channelOptions = {
tags: "".split(" "),
id: "557"
};
initTagRenderer("".split(" "), "".split(" "), channelOptions);
StackExchange.using("externalEditor", function() {
// Have to fire editor after snippets, if snippets enabled
if (StackExchange.settings.snippets.snippetsEnabled) {
StackExchange.using("snippets", function() {
createEditor();
});
}
else {
createEditor();
}
});
function createEditor() {
StackExchange.prepareEditor({
heartbeatType: 'answer',
autoActivateHeartbeat: false,
convertImagesToLinks: false,
noModals: true,
showLowRepImageUploadWarning: true,
reputationToPostImages: null,
bindNavPrevention: true,
postfix: "",
imageUploader: {
brandingHtml: "Powered by u003ca class="icon-imgur-white" href="https://imgur.com/"u003eu003c/au003e",
contentPolicyHtml: "User contributions licensed under u003ca href="https://creativecommons.org/licenses/by-sa/3.0/"u003ecc by-sa 3.0 with attribution requiredu003c/au003e u003ca href="https://stackoverflow.com/legal/content-policy"u003e(content policy)u003c/au003e",
allowUrls: true
},
onDemand: true,
discardSelector: ".discard-answer"
,immediatelyShowMarkdownHelp:true
});
}
});
Sign up or log in
StackExchange.ready(function () {
StackExchange.helpers.onClickDraftSave('#login-link');
});
Sign up using Google
Sign up using Facebook
Sign up using Email and Password
Post as a guest
Required, but never shown
StackExchange.ready(
function () {
StackExchange.openid.initPostLogin('.new-post-login', 'https%3a%2f%2fdatascience.stackexchange.com%2fquestions%2f36906%2fusing-unsupervised-learning-algorithms-on-images%23new-answer', 'question_page');
}
);
Post as a guest
Required, but never shown
1 Answer
1
active
oldest
votes
1 Answer
1
active
oldest
votes
active
oldest
votes
active
oldest
votes
$begingroup$
Have you included dropout in your model? It can help avoid overfitting issue.
For your problem, yes, you can use auto-encoders, GAN, etc. for feature learning.
However, I'm not sure if unsupervised learning can help, since it's more like a training issue. Your have label with your data so supervised learning is ideal, plus supervised learning generally shows better performance than unsupervised in image classification. You might want to check the false classification examples in your dataset, and try to alter the CNN structure based on that, which would be a more direct way.
$endgroup$
$begingroup$
Yes I have used dropout for my network. but That does not seem to have much effect. The problem is if you are familiar with Indian clothing (kurta is very similar to salwar) And since my dataset comprises of both the types of clothing, the program does not work well. should i try increasing the data size though i dod not know if that will have that big of an impact
$endgroup$
– Sashaank
Aug 14 '18 at 6:07
$begingroup$
I checked google for them, it seems the main difference is the shape. CNN should be able to recognize such difference. Usually I will try to take the data for these two label out and train CNN for them only, and then see if can classify between them. If true, it means the degradation of model is caused by the introduction of multi-class classification. Otherwise, it's simply caused by the model structure, and you might want to work on that.
$endgroup$
– plpopk
Aug 14 '18 at 7:00
$begingroup$
I will try that. thanks. Any idea on how to deal with multi classes?
$endgroup$
– Sashaank
Aug 14 '18 at 7:54
$begingroup$
Check if you used softmax activation. At the moment, what come to my mind is either adjust the cost function or add extra models (e.g. combine with a binary classification model which works well).
$endgroup$
– plpopk
Aug 14 '18 at 8:32
add a comment |
$begingroup$
Have you included dropout in your model? It can help avoid overfitting issue.
For your problem, yes, you can use auto-encoders, GAN, etc. for feature learning.
However, I'm not sure if unsupervised learning can help, since it's more like a training issue. Your have label with your data so supervised learning is ideal, plus supervised learning generally shows better performance than unsupervised in image classification. You might want to check the false classification examples in your dataset, and try to alter the CNN structure based on that, which would be a more direct way.
$endgroup$
$begingroup$
Yes I have used dropout for my network. but That does not seem to have much effect. The problem is if you are familiar with Indian clothing (kurta is very similar to salwar) And since my dataset comprises of both the types of clothing, the program does not work well. should i try increasing the data size though i dod not know if that will have that big of an impact
$endgroup$
– Sashaank
Aug 14 '18 at 6:07
$begingroup$
I checked google for them, it seems the main difference is the shape. CNN should be able to recognize such difference. Usually I will try to take the data for these two label out and train CNN for them only, and then see if can classify between them. If true, it means the degradation of model is caused by the introduction of multi-class classification. Otherwise, it's simply caused by the model structure, and you might want to work on that.
$endgroup$
– plpopk
Aug 14 '18 at 7:00
$begingroup$
I will try that. thanks. Any idea on how to deal with multi classes?
$endgroup$
– Sashaank
Aug 14 '18 at 7:54
$begingroup$
Check if you used softmax activation. At the moment, what come to my mind is either adjust the cost function or add extra models (e.g. combine with a binary classification model which works well).
$endgroup$
– plpopk
Aug 14 '18 at 8:32
add a comment |
$begingroup$
Have you included dropout in your model? It can help avoid overfitting issue.
For your problem, yes, you can use auto-encoders, GAN, etc. for feature learning.
However, I'm not sure if unsupervised learning can help, since it's more like a training issue. Your have label with your data so supervised learning is ideal, plus supervised learning generally shows better performance than unsupervised in image classification. You might want to check the false classification examples in your dataset, and try to alter the CNN structure based on that, which would be a more direct way.
$endgroup$
Have you included dropout in your model? It can help avoid overfitting issue.
For your problem, yes, you can use auto-encoders, GAN, etc. for feature learning.
However, I'm not sure if unsupervised learning can help, since it's more like a training issue. Your have label with your data so supervised learning is ideal, plus supervised learning generally shows better performance than unsupervised in image classification. You might want to check the false classification examples in your dataset, and try to alter the CNN structure based on that, which would be a more direct way.
answered Aug 14 '18 at 5:31
plpopkplpopk
1038
1038
$begingroup$
Yes I have used dropout for my network. but That does not seem to have much effect. The problem is if you are familiar with Indian clothing (kurta is very similar to salwar) And since my dataset comprises of both the types of clothing, the program does not work well. should i try increasing the data size though i dod not know if that will have that big of an impact
$endgroup$
– Sashaank
Aug 14 '18 at 6:07
$begingroup$
I checked google for them, it seems the main difference is the shape. CNN should be able to recognize such difference. Usually I will try to take the data for these two label out and train CNN for them only, and then see if can classify between them. If true, it means the degradation of model is caused by the introduction of multi-class classification. Otherwise, it's simply caused by the model structure, and you might want to work on that.
$endgroup$
– plpopk
Aug 14 '18 at 7:00
$begingroup$
I will try that. thanks. Any idea on how to deal with multi classes?
$endgroup$
– Sashaank
Aug 14 '18 at 7:54
$begingroup$
Check if you used softmax activation. At the moment, what come to my mind is either adjust the cost function or add extra models (e.g. combine with a binary classification model which works well).
$endgroup$
– plpopk
Aug 14 '18 at 8:32
add a comment |
$begingroup$
Yes I have used dropout for my network. but That does not seem to have much effect. The problem is if you are familiar with Indian clothing (kurta is very similar to salwar) And since my dataset comprises of both the types of clothing, the program does not work well. should i try increasing the data size though i dod not know if that will have that big of an impact
$endgroup$
– Sashaank
Aug 14 '18 at 6:07
$begingroup$
I checked google for them, it seems the main difference is the shape. CNN should be able to recognize such difference. Usually I will try to take the data for these two label out and train CNN for them only, and then see if can classify between them. If true, it means the degradation of model is caused by the introduction of multi-class classification. Otherwise, it's simply caused by the model structure, and you might want to work on that.
$endgroup$
– plpopk
Aug 14 '18 at 7:00
$begingroup$
I will try that. thanks. Any idea on how to deal with multi classes?
$endgroup$
– Sashaank
Aug 14 '18 at 7:54
$begingroup$
Check if you used softmax activation. At the moment, what come to my mind is either adjust the cost function or add extra models (e.g. combine with a binary classification model which works well).
$endgroup$
– plpopk
Aug 14 '18 at 8:32
$begingroup$
Yes I have used dropout for my network. but That does not seem to have much effect. The problem is if you are familiar with Indian clothing (kurta is very similar to salwar) And since my dataset comprises of both the types of clothing, the program does not work well. should i try increasing the data size though i dod not know if that will have that big of an impact
$endgroup$
– Sashaank
Aug 14 '18 at 6:07
$begingroup$
Yes I have used dropout for my network. but That does not seem to have much effect. The problem is if you are familiar with Indian clothing (kurta is very similar to salwar) And since my dataset comprises of both the types of clothing, the program does not work well. should i try increasing the data size though i dod not know if that will have that big of an impact
$endgroup$
– Sashaank
Aug 14 '18 at 6:07
$begingroup$
I checked google for them, it seems the main difference is the shape. CNN should be able to recognize such difference. Usually I will try to take the data for these two label out and train CNN for them only, and then see if can classify between them. If true, it means the degradation of model is caused by the introduction of multi-class classification. Otherwise, it's simply caused by the model structure, and you might want to work on that.
$endgroup$
– plpopk
Aug 14 '18 at 7:00
$begingroup$
I checked google for them, it seems the main difference is the shape. CNN should be able to recognize such difference. Usually I will try to take the data for these two label out and train CNN for them only, and then see if can classify between them. If true, it means the degradation of model is caused by the introduction of multi-class classification. Otherwise, it's simply caused by the model structure, and you might want to work on that.
$endgroup$
– plpopk
Aug 14 '18 at 7:00
$begingroup$
I will try that. thanks. Any idea on how to deal with multi classes?
$endgroup$
– Sashaank
Aug 14 '18 at 7:54
$begingroup$
I will try that. thanks. Any idea on how to deal with multi classes?
$endgroup$
– Sashaank
Aug 14 '18 at 7:54
$begingroup$
Check if you used softmax activation. At the moment, what come to my mind is either adjust the cost function or add extra models (e.g. combine with a binary classification model which works well).
$endgroup$
– plpopk
Aug 14 '18 at 8:32
$begingroup$
Check if you used softmax activation. At the moment, what come to my mind is either adjust the cost function or add extra models (e.g. combine with a binary classification model which works well).
$endgroup$
– plpopk
Aug 14 '18 at 8:32
add a comment |
Thanks for contributing an answer to Data Science Stack Exchange!
- Please be sure to answer the question. Provide details and share your research!
But avoid …
- Asking for help, clarification, or responding to other answers.
- Making statements based on opinion; back them up with references or personal experience.
Use MathJax to format equations. MathJax reference.
To learn more, see our tips on writing great answers.
Sign up or log in
StackExchange.ready(function () {
StackExchange.helpers.onClickDraftSave('#login-link');
});
Sign up using Google
Sign up using Facebook
Sign up using Email and Password
Post as a guest
Required, but never shown
StackExchange.ready(
function () {
StackExchange.openid.initPostLogin('.new-post-login', 'https%3a%2f%2fdatascience.stackexchange.com%2fquestions%2f36906%2fusing-unsupervised-learning-algorithms-on-images%23new-answer', 'question_page');
}
);
Post as a guest
Required, but never shown
Sign up or log in
StackExchange.ready(function () {
StackExchange.helpers.onClickDraftSave('#login-link');
});
Sign up using Google
Sign up using Facebook
Sign up using Email and Password
Post as a guest
Required, but never shown
Sign up or log in
StackExchange.ready(function () {
StackExchange.helpers.onClickDraftSave('#login-link');
});
Sign up using Google
Sign up using Facebook
Sign up using Email and Password
Post as a guest
Required, but never shown
Sign up or log in
StackExchange.ready(function () {
StackExchange.helpers.onClickDraftSave('#login-link');
});
Sign up using Google
Sign up using Facebook
Sign up using Email and Password
Sign up using Google
Sign up using Facebook
Sign up using Email and Password
Post as a guest
Required, but never shown
Required, but never shown
Required, but never shown
Required, but never shown
Required, but never shown
Required, but never shown
Required, but never shown
Required, but never shown
Required, but never shown
u1KJ,vxjK5hNYD 63AtTf6XHZ2lIZS,qFqbG,tb6PxxBmONe3c26jtsdPdAcet4jGQmBFwYKUYxaxSrJkiw HwvA7FMF UFAYSLm5LsM P
$begingroup$
Did you try data augmentation (rotating your images....)
$endgroup$
– Robin Nicole
Dec 12 '18 at 20:04
$begingroup$
Unsupervised learning is not going to perform better than a well trained CNN for so many images. You should reduce overfitting on your CNN. For example try a smaller model, or Data Augmentation, or adding dropout, or tuning batchsize/learningrate. Or use a pretrained model that you finetune
$endgroup$
– jonnor
yesterday