Understanding the Gini/AUC metric as out-of-development performance metric
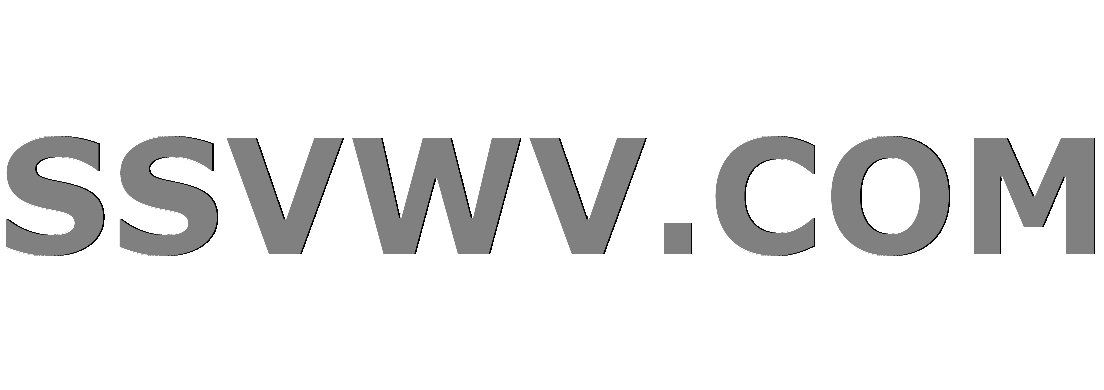
Multi tool use
$begingroup$
Assume we develop a model for a binary classification task that reaches a certain Gini/AUROC estimate on the validation ( or training ) sample, among others. This is an overall good metric, often used for evaluating the ability of the model to separate the samples into, say, goods vs bads.
Further, assume this model is adequate and will be used for further collection of new samples with a certain cutoff value. What should be expected Gini/AUC estimates on the newly collected sample?
From what I'm noticing, on the training sample there were clear cases where the model was able to distinguish and separate it with large probabilities. On the other hand, with applied cuttoff, say, <50%, the new sample with collect only those cases where no such clear separation is possible (because if it would, the case might not get collected). With such approach, for me it seems logical that the overall separation in the new sample will be lower, resulting in lower out-of-development-period Gini/AUC.
Is this the expected behaviour in normal production environments? Am I understanding things correctly?
Note: I understand that there are other simple metrics, such as sensitivity/specificity, hoslem.test and others, allowing for measuring and visualising True/False Positives. However, I have found that Gini/AUC is often a key metric when discussing and comparing classification models.
classification metric
$endgroup$
add a comment |
$begingroup$
Assume we develop a model for a binary classification task that reaches a certain Gini/AUROC estimate on the validation ( or training ) sample, among others. This is an overall good metric, often used for evaluating the ability of the model to separate the samples into, say, goods vs bads.
Further, assume this model is adequate and will be used for further collection of new samples with a certain cutoff value. What should be expected Gini/AUC estimates on the newly collected sample?
From what I'm noticing, on the training sample there were clear cases where the model was able to distinguish and separate it with large probabilities. On the other hand, with applied cuttoff, say, <50%, the new sample with collect only those cases where no such clear separation is possible (because if it would, the case might not get collected). With such approach, for me it seems logical that the overall separation in the new sample will be lower, resulting in lower out-of-development-period Gini/AUC.
Is this the expected behaviour in normal production environments? Am I understanding things correctly?
Note: I understand that there are other simple metrics, such as sensitivity/specificity, hoslem.test and others, allowing for measuring and visualising True/False Positives. However, I have found that Gini/AUC is often a key metric when discussing and comparing classification models.
classification metric
$endgroup$
add a comment |
$begingroup$
Assume we develop a model for a binary classification task that reaches a certain Gini/AUROC estimate on the validation ( or training ) sample, among others. This is an overall good metric, often used for evaluating the ability of the model to separate the samples into, say, goods vs bads.
Further, assume this model is adequate and will be used for further collection of new samples with a certain cutoff value. What should be expected Gini/AUC estimates on the newly collected sample?
From what I'm noticing, on the training sample there were clear cases where the model was able to distinguish and separate it with large probabilities. On the other hand, with applied cuttoff, say, <50%, the new sample with collect only those cases where no such clear separation is possible (because if it would, the case might not get collected). With such approach, for me it seems logical that the overall separation in the new sample will be lower, resulting in lower out-of-development-period Gini/AUC.
Is this the expected behaviour in normal production environments? Am I understanding things correctly?
Note: I understand that there are other simple metrics, such as sensitivity/specificity, hoslem.test and others, allowing for measuring and visualising True/False Positives. However, I have found that Gini/AUC is often a key metric when discussing and comparing classification models.
classification metric
$endgroup$
Assume we develop a model for a binary classification task that reaches a certain Gini/AUROC estimate on the validation ( or training ) sample, among others. This is an overall good metric, often used for evaluating the ability of the model to separate the samples into, say, goods vs bads.
Further, assume this model is adequate and will be used for further collection of new samples with a certain cutoff value. What should be expected Gini/AUC estimates on the newly collected sample?
From what I'm noticing, on the training sample there were clear cases where the model was able to distinguish and separate it with large probabilities. On the other hand, with applied cuttoff, say, <50%, the new sample with collect only those cases where no such clear separation is possible (because if it would, the case might not get collected). With such approach, for me it seems logical that the overall separation in the new sample will be lower, resulting in lower out-of-development-period Gini/AUC.
Is this the expected behaviour in normal production environments? Am I understanding things correctly?
Note: I understand that there are other simple metrics, such as sensitivity/specificity, hoslem.test and others, allowing for measuring and visualising True/False Positives. However, I have found that Gini/AUC is often a key metric when discussing and comparing classification models.
classification metric
classification metric
edited Dec 3 '18 at 10:01
Nutle
asked Dec 3 '18 at 9:51
NutleNutle
18117
18117
add a comment |
add a comment |
1 Answer
1
active
oldest
votes
$begingroup$
The advantage which train/test/validation dataset separation has is that you separate your dataset into:
- The individuals which you know the exogenous variables and the output: Training
- The individuals which you know the exogenous variables and the output (but you suppose you don't know which the output is): Test
- The individuals you know the exogenous variables but not the output: Validation
Every DS or ML model is made so it is prepared to receive a validation dataset in the future and try to get every metric just almost as good as if it was the train dataset.
The test dataset has the objective of simulating the situation of having data but not output, and then you have the output to measure the behaviour and comparing the modelled vs real output.
So, for a concrete answer:
The behaviour you should expect from the validation (or newly collected sample) is the same as the test dataset.
Given that the underlying phenomenon and sampling technique remains the same.
For more information:
https://towardsdatascience.com/train-validation-and-test-sets-72cb40cba9e7
New contributor
Juan Esteban de la Calle is a new contributor to this site. Take care in asking for clarification, commenting, and answering.
Check out our Code of Conduct.
$endgroup$
$begingroup$
Thanks. My main question in a way is about AUC as a metric, which is a full sample based metric. Naturally, its properties should change when the validation sample is censored (applied cutoff rule), but how much change is expected? I understand there are other metrics, but gini/auc are too popular to ignore :)
$endgroup$
– Nutle
yesterday
$begingroup$
In other words, after applying cutoff, as in your bolded sentence, the sampling technique will not be the same anymore. So, whats then?
$endgroup$
– Nutle
yesterday
1
$begingroup$
There is no metric which is more or less sensitive to overfitting than others, so from AUC/Gini you should expect the same: You should expect the same decreased as when comparing test vs train datasets.
$endgroup$
– Juan Esteban de la Calle
yesterday
$begingroup$
If gini measures separability between (say) two classes, goods and bads, would you agree that after applying a certain cutoff, removing cases that are certainly bad, the gini of the new sample will surely be lower? Since if we remove the low hanging fruit, the remaining level of separability must decrease - because in other case, I would proceed to remove them until the separation is not clear/certain enough?
$endgroup$
– Nutle
yesterday
$begingroup$
Let me recap, you collect future samples based on the results of your previous model?
$endgroup$
– Juan Esteban de la Calle
yesterday
|
show 3 more comments
Your Answer
StackExchange.ready(function() {
var channelOptions = {
tags: "".split(" "),
id: "557"
};
initTagRenderer("".split(" "), "".split(" "), channelOptions);
StackExchange.using("externalEditor", function() {
// Have to fire editor after snippets, if snippets enabled
if (StackExchange.settings.snippets.snippetsEnabled) {
StackExchange.using("snippets", function() {
createEditor();
});
}
else {
createEditor();
}
});
function createEditor() {
StackExchange.prepareEditor({
heartbeatType: 'answer',
autoActivateHeartbeat: false,
convertImagesToLinks: false,
noModals: true,
showLowRepImageUploadWarning: true,
reputationToPostImages: null,
bindNavPrevention: true,
postfix: "",
imageUploader: {
brandingHtml: "Powered by u003ca class="icon-imgur-white" href="https://imgur.com/"u003eu003c/au003e",
contentPolicyHtml: "User contributions licensed under u003ca href="https://creativecommons.org/licenses/by-sa/3.0/"u003ecc by-sa 3.0 with attribution requiredu003c/au003e u003ca href="https://stackoverflow.com/legal/content-policy"u003e(content policy)u003c/au003e",
allowUrls: true
},
onDemand: true,
discardSelector: ".discard-answer"
,immediatelyShowMarkdownHelp:true
});
}
});
Sign up or log in
StackExchange.ready(function () {
StackExchange.helpers.onClickDraftSave('#login-link');
});
Sign up using Google
Sign up using Facebook
Sign up using Email and Password
Post as a guest
Required, but never shown
StackExchange.ready(
function () {
StackExchange.openid.initPostLogin('.new-post-login', 'https%3a%2f%2fdatascience.stackexchange.com%2fquestions%2f42029%2funderstanding-the-gini-auc-metric-as-out-of-development-performance-metric%23new-answer', 'question_page');
}
);
Post as a guest
Required, but never shown
1 Answer
1
active
oldest
votes
1 Answer
1
active
oldest
votes
active
oldest
votes
active
oldest
votes
$begingroup$
The advantage which train/test/validation dataset separation has is that you separate your dataset into:
- The individuals which you know the exogenous variables and the output: Training
- The individuals which you know the exogenous variables and the output (but you suppose you don't know which the output is): Test
- The individuals you know the exogenous variables but not the output: Validation
Every DS or ML model is made so it is prepared to receive a validation dataset in the future and try to get every metric just almost as good as if it was the train dataset.
The test dataset has the objective of simulating the situation of having data but not output, and then you have the output to measure the behaviour and comparing the modelled vs real output.
So, for a concrete answer:
The behaviour you should expect from the validation (or newly collected sample) is the same as the test dataset.
Given that the underlying phenomenon and sampling technique remains the same.
For more information:
https://towardsdatascience.com/train-validation-and-test-sets-72cb40cba9e7
New contributor
Juan Esteban de la Calle is a new contributor to this site. Take care in asking for clarification, commenting, and answering.
Check out our Code of Conduct.
$endgroup$
$begingroup$
Thanks. My main question in a way is about AUC as a metric, which is a full sample based metric. Naturally, its properties should change when the validation sample is censored (applied cutoff rule), but how much change is expected? I understand there are other metrics, but gini/auc are too popular to ignore :)
$endgroup$
– Nutle
yesterday
$begingroup$
In other words, after applying cutoff, as in your bolded sentence, the sampling technique will not be the same anymore. So, whats then?
$endgroup$
– Nutle
yesterday
1
$begingroup$
There is no metric which is more or less sensitive to overfitting than others, so from AUC/Gini you should expect the same: You should expect the same decreased as when comparing test vs train datasets.
$endgroup$
– Juan Esteban de la Calle
yesterday
$begingroup$
If gini measures separability between (say) two classes, goods and bads, would you agree that after applying a certain cutoff, removing cases that are certainly bad, the gini of the new sample will surely be lower? Since if we remove the low hanging fruit, the remaining level of separability must decrease - because in other case, I would proceed to remove them until the separation is not clear/certain enough?
$endgroup$
– Nutle
yesterday
$begingroup$
Let me recap, you collect future samples based on the results of your previous model?
$endgroup$
– Juan Esteban de la Calle
yesterday
|
show 3 more comments
$begingroup$
The advantage which train/test/validation dataset separation has is that you separate your dataset into:
- The individuals which you know the exogenous variables and the output: Training
- The individuals which you know the exogenous variables and the output (but you suppose you don't know which the output is): Test
- The individuals you know the exogenous variables but not the output: Validation
Every DS or ML model is made so it is prepared to receive a validation dataset in the future and try to get every metric just almost as good as if it was the train dataset.
The test dataset has the objective of simulating the situation of having data but not output, and then you have the output to measure the behaviour and comparing the modelled vs real output.
So, for a concrete answer:
The behaviour you should expect from the validation (or newly collected sample) is the same as the test dataset.
Given that the underlying phenomenon and sampling technique remains the same.
For more information:
https://towardsdatascience.com/train-validation-and-test-sets-72cb40cba9e7
New contributor
Juan Esteban de la Calle is a new contributor to this site. Take care in asking for clarification, commenting, and answering.
Check out our Code of Conduct.
$endgroup$
$begingroup$
Thanks. My main question in a way is about AUC as a metric, which is a full sample based metric. Naturally, its properties should change when the validation sample is censored (applied cutoff rule), but how much change is expected? I understand there are other metrics, but gini/auc are too popular to ignore :)
$endgroup$
– Nutle
yesterday
$begingroup$
In other words, after applying cutoff, as in your bolded sentence, the sampling technique will not be the same anymore. So, whats then?
$endgroup$
– Nutle
yesterday
1
$begingroup$
There is no metric which is more or less sensitive to overfitting than others, so from AUC/Gini you should expect the same: You should expect the same decreased as when comparing test vs train datasets.
$endgroup$
– Juan Esteban de la Calle
yesterday
$begingroup$
If gini measures separability between (say) two classes, goods and bads, would you agree that after applying a certain cutoff, removing cases that are certainly bad, the gini of the new sample will surely be lower? Since if we remove the low hanging fruit, the remaining level of separability must decrease - because in other case, I would proceed to remove them until the separation is not clear/certain enough?
$endgroup$
– Nutle
yesterday
$begingroup$
Let me recap, you collect future samples based on the results of your previous model?
$endgroup$
– Juan Esteban de la Calle
yesterday
|
show 3 more comments
$begingroup$
The advantage which train/test/validation dataset separation has is that you separate your dataset into:
- The individuals which you know the exogenous variables and the output: Training
- The individuals which you know the exogenous variables and the output (but you suppose you don't know which the output is): Test
- The individuals you know the exogenous variables but not the output: Validation
Every DS or ML model is made so it is prepared to receive a validation dataset in the future and try to get every metric just almost as good as if it was the train dataset.
The test dataset has the objective of simulating the situation of having data but not output, and then you have the output to measure the behaviour and comparing the modelled vs real output.
So, for a concrete answer:
The behaviour you should expect from the validation (or newly collected sample) is the same as the test dataset.
Given that the underlying phenomenon and sampling technique remains the same.
For more information:
https://towardsdatascience.com/train-validation-and-test-sets-72cb40cba9e7
New contributor
Juan Esteban de la Calle is a new contributor to this site. Take care in asking for clarification, commenting, and answering.
Check out our Code of Conduct.
$endgroup$
The advantage which train/test/validation dataset separation has is that you separate your dataset into:
- The individuals which you know the exogenous variables and the output: Training
- The individuals which you know the exogenous variables and the output (but you suppose you don't know which the output is): Test
- The individuals you know the exogenous variables but not the output: Validation
Every DS or ML model is made so it is prepared to receive a validation dataset in the future and try to get every metric just almost as good as if it was the train dataset.
The test dataset has the objective of simulating the situation of having data but not output, and then you have the output to measure the behaviour and comparing the modelled vs real output.
So, for a concrete answer:
The behaviour you should expect from the validation (or newly collected sample) is the same as the test dataset.
Given that the underlying phenomenon and sampling technique remains the same.
For more information:
https://towardsdatascience.com/train-validation-and-test-sets-72cb40cba9e7
New contributor
Juan Esteban de la Calle is a new contributor to this site. Take care in asking for clarification, commenting, and answering.
Check out our Code of Conduct.
New contributor
Juan Esteban de la Calle is a new contributor to this site. Take care in asking for clarification, commenting, and answering.
Check out our Code of Conduct.
answered yesterday
Juan Esteban de la CalleJuan Esteban de la Calle
687
687
New contributor
Juan Esteban de la Calle is a new contributor to this site. Take care in asking for clarification, commenting, and answering.
Check out our Code of Conduct.
New contributor
Juan Esteban de la Calle is a new contributor to this site. Take care in asking for clarification, commenting, and answering.
Check out our Code of Conduct.
Juan Esteban de la Calle is a new contributor to this site. Take care in asking for clarification, commenting, and answering.
Check out our Code of Conduct.
$begingroup$
Thanks. My main question in a way is about AUC as a metric, which is a full sample based metric. Naturally, its properties should change when the validation sample is censored (applied cutoff rule), but how much change is expected? I understand there are other metrics, but gini/auc are too popular to ignore :)
$endgroup$
– Nutle
yesterday
$begingroup$
In other words, after applying cutoff, as in your bolded sentence, the sampling technique will not be the same anymore. So, whats then?
$endgroup$
– Nutle
yesterday
1
$begingroup$
There is no metric which is more or less sensitive to overfitting than others, so from AUC/Gini you should expect the same: You should expect the same decreased as when comparing test vs train datasets.
$endgroup$
– Juan Esteban de la Calle
yesterday
$begingroup$
If gini measures separability between (say) two classes, goods and bads, would you agree that after applying a certain cutoff, removing cases that are certainly bad, the gini of the new sample will surely be lower? Since if we remove the low hanging fruit, the remaining level of separability must decrease - because in other case, I would proceed to remove them until the separation is not clear/certain enough?
$endgroup$
– Nutle
yesterday
$begingroup$
Let me recap, you collect future samples based on the results of your previous model?
$endgroup$
– Juan Esteban de la Calle
yesterday
|
show 3 more comments
$begingroup$
Thanks. My main question in a way is about AUC as a metric, which is a full sample based metric. Naturally, its properties should change when the validation sample is censored (applied cutoff rule), but how much change is expected? I understand there are other metrics, but gini/auc are too popular to ignore :)
$endgroup$
– Nutle
yesterday
$begingroup$
In other words, after applying cutoff, as in your bolded sentence, the sampling technique will not be the same anymore. So, whats then?
$endgroup$
– Nutle
yesterday
1
$begingroup$
There is no metric which is more or less sensitive to overfitting than others, so from AUC/Gini you should expect the same: You should expect the same decreased as when comparing test vs train datasets.
$endgroup$
– Juan Esteban de la Calle
yesterday
$begingroup$
If gini measures separability between (say) two classes, goods and bads, would you agree that after applying a certain cutoff, removing cases that are certainly bad, the gini of the new sample will surely be lower? Since if we remove the low hanging fruit, the remaining level of separability must decrease - because in other case, I would proceed to remove them until the separation is not clear/certain enough?
$endgroup$
– Nutle
yesterday
$begingroup$
Let me recap, you collect future samples based on the results of your previous model?
$endgroup$
– Juan Esteban de la Calle
yesterday
$begingroup$
Thanks. My main question in a way is about AUC as a metric, which is a full sample based metric. Naturally, its properties should change when the validation sample is censored (applied cutoff rule), but how much change is expected? I understand there are other metrics, but gini/auc are too popular to ignore :)
$endgroup$
– Nutle
yesterday
$begingroup$
Thanks. My main question in a way is about AUC as a metric, which is a full sample based metric. Naturally, its properties should change when the validation sample is censored (applied cutoff rule), but how much change is expected? I understand there are other metrics, but gini/auc are too popular to ignore :)
$endgroup$
– Nutle
yesterday
$begingroup$
In other words, after applying cutoff, as in your bolded sentence, the sampling technique will not be the same anymore. So, whats then?
$endgroup$
– Nutle
yesterday
$begingroup$
In other words, after applying cutoff, as in your bolded sentence, the sampling technique will not be the same anymore. So, whats then?
$endgroup$
– Nutle
yesterday
1
1
$begingroup$
There is no metric which is more or less sensitive to overfitting than others, so from AUC/Gini you should expect the same: You should expect the same decreased as when comparing test vs train datasets.
$endgroup$
– Juan Esteban de la Calle
yesterday
$begingroup$
There is no metric which is more or less sensitive to overfitting than others, so from AUC/Gini you should expect the same: You should expect the same decreased as when comparing test vs train datasets.
$endgroup$
– Juan Esteban de la Calle
yesterday
$begingroup$
If gini measures separability between (say) two classes, goods and bads, would you agree that after applying a certain cutoff, removing cases that are certainly bad, the gini of the new sample will surely be lower? Since if we remove the low hanging fruit, the remaining level of separability must decrease - because in other case, I would proceed to remove them until the separation is not clear/certain enough?
$endgroup$
– Nutle
yesterday
$begingroup$
If gini measures separability between (say) two classes, goods and bads, would you agree that after applying a certain cutoff, removing cases that are certainly bad, the gini of the new sample will surely be lower? Since if we remove the low hanging fruit, the remaining level of separability must decrease - because in other case, I would proceed to remove them until the separation is not clear/certain enough?
$endgroup$
– Nutle
yesterday
$begingroup$
Let me recap, you collect future samples based on the results of your previous model?
$endgroup$
– Juan Esteban de la Calle
yesterday
$begingroup$
Let me recap, you collect future samples based on the results of your previous model?
$endgroup$
– Juan Esteban de la Calle
yesterday
|
show 3 more comments
Thanks for contributing an answer to Data Science Stack Exchange!
- Please be sure to answer the question. Provide details and share your research!
But avoid …
- Asking for help, clarification, or responding to other answers.
- Making statements based on opinion; back them up with references or personal experience.
Use MathJax to format equations. MathJax reference.
To learn more, see our tips on writing great answers.
Sign up or log in
StackExchange.ready(function () {
StackExchange.helpers.onClickDraftSave('#login-link');
});
Sign up using Google
Sign up using Facebook
Sign up using Email and Password
Post as a guest
Required, but never shown
StackExchange.ready(
function () {
StackExchange.openid.initPostLogin('.new-post-login', 'https%3a%2f%2fdatascience.stackexchange.com%2fquestions%2f42029%2funderstanding-the-gini-auc-metric-as-out-of-development-performance-metric%23new-answer', 'question_page');
}
);
Post as a guest
Required, but never shown
Sign up or log in
StackExchange.ready(function () {
StackExchange.helpers.onClickDraftSave('#login-link');
});
Sign up using Google
Sign up using Facebook
Sign up using Email and Password
Post as a guest
Required, but never shown
Sign up or log in
StackExchange.ready(function () {
StackExchange.helpers.onClickDraftSave('#login-link');
});
Sign up using Google
Sign up using Facebook
Sign up using Email and Password
Post as a guest
Required, but never shown
Sign up or log in
StackExchange.ready(function () {
StackExchange.helpers.onClickDraftSave('#login-link');
});
Sign up using Google
Sign up using Facebook
Sign up using Email and Password
Sign up using Google
Sign up using Facebook
Sign up using Email and Password
Post as a guest
Required, but never shown
Required, but never shown
Required, but never shown
Required, but never shown
Required, but never shown
Required, but never shown
Required, but never shown
Required, but never shown
Required, but never shown
nVJ75EasZdNiR6tarAkUPk2IoL2lEbTclQtC