Story Tag Prediction - Optional Labels
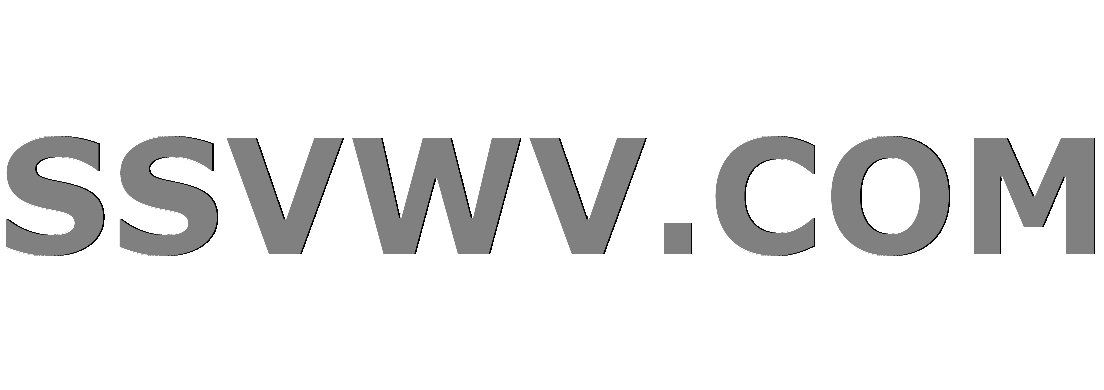
Multi tool use
$begingroup$
I'm currently working on a prediction for fiction. I have a database with fiction, which are each described with different story tags. My idea is to use a neural network that can tell you by processing a new story which tags are relevant.
The problem is, that the original data wasn't generated but added by users. A story in the woods could be tagged with trees, nature etc. Another story that also takes place in the woods might not be tagged with nature, even though the tag applies. This might confuse the neural network. Is there a way to prevent this form happening?
Thank you!
nlp
New contributor
JoschJava is a new contributor to this site. Take care in asking for clarification, commenting, and answering.
Check out our Code of Conduct.
$endgroup$
add a comment |
$begingroup$
I'm currently working on a prediction for fiction. I have a database with fiction, which are each described with different story tags. My idea is to use a neural network that can tell you by processing a new story which tags are relevant.
The problem is, that the original data wasn't generated but added by users. A story in the woods could be tagged with trees, nature etc. Another story that also takes place in the woods might not be tagged with nature, even though the tag applies. This might confuse the neural network. Is there a way to prevent this form happening?
Thank you!
nlp
New contributor
JoschJava is a new contributor to this site. Take care in asking for clarification, commenting, and answering.
Check out our Code of Conduct.
$endgroup$
$begingroup$
Are you aiming at multi-label classification?
$endgroup$
– pythinker
yesterday
add a comment |
$begingroup$
I'm currently working on a prediction for fiction. I have a database with fiction, which are each described with different story tags. My idea is to use a neural network that can tell you by processing a new story which tags are relevant.
The problem is, that the original data wasn't generated but added by users. A story in the woods could be tagged with trees, nature etc. Another story that also takes place in the woods might not be tagged with nature, even though the tag applies. This might confuse the neural network. Is there a way to prevent this form happening?
Thank you!
nlp
New contributor
JoschJava is a new contributor to this site. Take care in asking for clarification, commenting, and answering.
Check out our Code of Conduct.
$endgroup$
I'm currently working on a prediction for fiction. I have a database with fiction, which are each described with different story tags. My idea is to use a neural network that can tell you by processing a new story which tags are relevant.
The problem is, that the original data wasn't generated but added by users. A story in the woods could be tagged with trees, nature etc. Another story that also takes place in the woods might not be tagged with nature, even though the tag applies. This might confuse the neural network. Is there a way to prevent this form happening?
Thank you!
nlp
nlp
New contributor
JoschJava is a new contributor to this site. Take care in asking for clarification, commenting, and answering.
Check out our Code of Conduct.
New contributor
JoschJava is a new contributor to this site. Take care in asking for clarification, commenting, and answering.
Check out our Code of Conduct.
New contributor
JoschJava is a new contributor to this site. Take care in asking for clarification, commenting, and answering.
Check out our Code of Conduct.
asked yesterday
JoschJavaJoschJava
61
61
New contributor
JoschJava is a new contributor to this site. Take care in asking for clarification, commenting, and answering.
Check out our Code of Conduct.
New contributor
JoschJava is a new contributor to this site. Take care in asking for clarification, commenting, and answering.
Check out our Code of Conduct.
JoschJava is a new contributor to this site. Take care in asking for clarification, commenting, and answering.
Check out our Code of Conduct.
$begingroup$
Are you aiming at multi-label classification?
$endgroup$
– pythinker
yesterday
add a comment |
$begingroup$
Are you aiming at multi-label classification?
$endgroup$
– pythinker
yesterday
$begingroup$
Are you aiming at multi-label classification?
$endgroup$
– pythinker
yesterday
$begingroup$
Are you aiming at multi-label classification?
$endgroup$
– pythinker
yesterday
add a comment |
1 Answer
1
active
oldest
votes
$begingroup$
Welcome to our community!
If I understood correctly, you don't trust the labels on your training dataset.
The problem is, that the original data wasn't generated but added by users.
That is not properly a problem, actually almost every dataset was created by human labeling. Your problem is that you don't trust the judgement of those users and you think that data might be incomplete.
It is true that this might affect your model's performance. But, you should try either way. Sometimes the model learns to label even better than the training dataset:
Recently I looked the master's dissertation of a friend (Wesley L. Passos,M.Sc. by UFRJ) which used deep learning to detect tires on drone images (for Aedes Aegyptis prevention procedures). The dataset was created by our group my manually annotating images with bounding boxes and while we missed some of the tires that were pretty well hidden the D-CNN model was capable of detecting those hard subjects.
Note: This dissertation was recently accepted and isn't available online yet. Once it does, I will update this answer with the proper reference.
Since wrangling with data is our everyday job this is a good opportunity for you to put in practice what you have learned:
Clean your data by either removing incomplete samples or filling missing values. This is a common part of our jobs as data scientists.
If you feel that the amount of work is a bit overwhelming you can try clustering instead or semi-supervised methods to speed up the cleaning process.
Also you can try posting your data online for further contributing.
Curiosity Note:
Crowds are usually more intelligent than individual, so with problem statistical treatment data can actually be better annotated by crowds, using mean answers or by voting. Check for The Wisdom of Crowds (Not the TV Show).
$endgroup$
add a comment |
Your Answer
StackExchange.ifUsing("editor", function () {
return StackExchange.using("mathjaxEditing", function () {
StackExchange.MarkdownEditor.creationCallbacks.add(function (editor, postfix) {
StackExchange.mathjaxEditing.prepareWmdForMathJax(editor, postfix, [["$", "$"], ["\\(","\\)"]]);
});
});
}, "mathjax-editing");
StackExchange.ready(function() {
var channelOptions = {
tags: "".split(" "),
id: "557"
};
initTagRenderer("".split(" "), "".split(" "), channelOptions);
StackExchange.using("externalEditor", function() {
// Have to fire editor after snippets, if snippets enabled
if (StackExchange.settings.snippets.snippetsEnabled) {
StackExchange.using("snippets", function() {
createEditor();
});
}
else {
createEditor();
}
});
function createEditor() {
StackExchange.prepareEditor({
heartbeatType: 'answer',
autoActivateHeartbeat: false,
convertImagesToLinks: false,
noModals: true,
showLowRepImageUploadWarning: true,
reputationToPostImages: null,
bindNavPrevention: true,
postfix: "",
imageUploader: {
brandingHtml: "Powered by u003ca class="icon-imgur-white" href="https://imgur.com/"u003eu003c/au003e",
contentPolicyHtml: "User contributions licensed under u003ca href="https://creativecommons.org/licenses/by-sa/3.0/"u003ecc by-sa 3.0 with attribution requiredu003c/au003e u003ca href="https://stackoverflow.com/legal/content-policy"u003e(content policy)u003c/au003e",
allowUrls: true
},
onDemand: true,
discardSelector: ".discard-answer"
,immediatelyShowMarkdownHelp:true
});
}
});
JoschJava is a new contributor. Be nice, and check out our Code of Conduct.
Sign up or log in
StackExchange.ready(function () {
StackExchange.helpers.onClickDraftSave('#login-link');
});
Sign up using Google
Sign up using Facebook
Sign up using Email and Password
Post as a guest
Required, but never shown
StackExchange.ready(
function () {
StackExchange.openid.initPostLogin('.new-post-login', 'https%3a%2f%2fdatascience.stackexchange.com%2fquestions%2f48966%2fstory-tag-prediction-optional-labels%23new-answer', 'question_page');
}
);
Post as a guest
Required, but never shown
1 Answer
1
active
oldest
votes
1 Answer
1
active
oldest
votes
active
oldest
votes
active
oldest
votes
$begingroup$
Welcome to our community!
If I understood correctly, you don't trust the labels on your training dataset.
The problem is, that the original data wasn't generated but added by users.
That is not properly a problem, actually almost every dataset was created by human labeling. Your problem is that you don't trust the judgement of those users and you think that data might be incomplete.
It is true that this might affect your model's performance. But, you should try either way. Sometimes the model learns to label even better than the training dataset:
Recently I looked the master's dissertation of a friend (Wesley L. Passos,M.Sc. by UFRJ) which used deep learning to detect tires on drone images (for Aedes Aegyptis prevention procedures). The dataset was created by our group my manually annotating images with bounding boxes and while we missed some of the tires that were pretty well hidden the D-CNN model was capable of detecting those hard subjects.
Note: This dissertation was recently accepted and isn't available online yet. Once it does, I will update this answer with the proper reference.
Since wrangling with data is our everyday job this is a good opportunity for you to put in practice what you have learned:
Clean your data by either removing incomplete samples or filling missing values. This is a common part of our jobs as data scientists.
If you feel that the amount of work is a bit overwhelming you can try clustering instead or semi-supervised methods to speed up the cleaning process.
Also you can try posting your data online for further contributing.
Curiosity Note:
Crowds are usually more intelligent than individual, so with problem statistical treatment data can actually be better annotated by crowds, using mean answers or by voting. Check for The Wisdom of Crowds (Not the TV Show).
$endgroup$
add a comment |
$begingroup$
Welcome to our community!
If I understood correctly, you don't trust the labels on your training dataset.
The problem is, that the original data wasn't generated but added by users.
That is not properly a problem, actually almost every dataset was created by human labeling. Your problem is that you don't trust the judgement of those users and you think that data might be incomplete.
It is true that this might affect your model's performance. But, you should try either way. Sometimes the model learns to label even better than the training dataset:
Recently I looked the master's dissertation of a friend (Wesley L. Passos,M.Sc. by UFRJ) which used deep learning to detect tires on drone images (for Aedes Aegyptis prevention procedures). The dataset was created by our group my manually annotating images with bounding boxes and while we missed some of the tires that were pretty well hidden the D-CNN model was capable of detecting those hard subjects.
Note: This dissertation was recently accepted and isn't available online yet. Once it does, I will update this answer with the proper reference.
Since wrangling with data is our everyday job this is a good opportunity for you to put in practice what you have learned:
Clean your data by either removing incomplete samples or filling missing values. This is a common part of our jobs as data scientists.
If you feel that the amount of work is a bit overwhelming you can try clustering instead or semi-supervised methods to speed up the cleaning process.
Also you can try posting your data online for further contributing.
Curiosity Note:
Crowds are usually more intelligent than individual, so with problem statistical treatment data can actually be better annotated by crowds, using mean answers or by voting. Check for The Wisdom of Crowds (Not the TV Show).
$endgroup$
add a comment |
$begingroup$
Welcome to our community!
If I understood correctly, you don't trust the labels on your training dataset.
The problem is, that the original data wasn't generated but added by users.
That is not properly a problem, actually almost every dataset was created by human labeling. Your problem is that you don't trust the judgement of those users and you think that data might be incomplete.
It is true that this might affect your model's performance. But, you should try either way. Sometimes the model learns to label even better than the training dataset:
Recently I looked the master's dissertation of a friend (Wesley L. Passos,M.Sc. by UFRJ) which used deep learning to detect tires on drone images (for Aedes Aegyptis prevention procedures). The dataset was created by our group my manually annotating images with bounding boxes and while we missed some of the tires that were pretty well hidden the D-CNN model was capable of detecting those hard subjects.
Note: This dissertation was recently accepted and isn't available online yet. Once it does, I will update this answer with the proper reference.
Since wrangling with data is our everyday job this is a good opportunity for you to put in practice what you have learned:
Clean your data by either removing incomplete samples or filling missing values. This is a common part of our jobs as data scientists.
If you feel that the amount of work is a bit overwhelming you can try clustering instead or semi-supervised methods to speed up the cleaning process.
Also you can try posting your data online for further contributing.
Curiosity Note:
Crowds are usually more intelligent than individual, so with problem statistical treatment data can actually be better annotated by crowds, using mean answers or by voting. Check for The Wisdom of Crowds (Not the TV Show).
$endgroup$
Welcome to our community!
If I understood correctly, you don't trust the labels on your training dataset.
The problem is, that the original data wasn't generated but added by users.
That is not properly a problem, actually almost every dataset was created by human labeling. Your problem is that you don't trust the judgement of those users and you think that data might be incomplete.
It is true that this might affect your model's performance. But, you should try either way. Sometimes the model learns to label even better than the training dataset:
Recently I looked the master's dissertation of a friend (Wesley L. Passos,M.Sc. by UFRJ) which used deep learning to detect tires on drone images (for Aedes Aegyptis prevention procedures). The dataset was created by our group my manually annotating images with bounding boxes and while we missed some of the tires that were pretty well hidden the D-CNN model was capable of detecting those hard subjects.
Note: This dissertation was recently accepted and isn't available online yet. Once it does, I will update this answer with the proper reference.
Since wrangling with data is our everyday job this is a good opportunity for you to put in practice what you have learned:
Clean your data by either removing incomplete samples or filling missing values. This is a common part of our jobs as data scientists.
If you feel that the amount of work is a bit overwhelming you can try clustering instead or semi-supervised methods to speed up the cleaning process.
Also you can try posting your data online for further contributing.
Curiosity Note:
Crowds are usually more intelligent than individual, so with problem statistical treatment data can actually be better annotated by crowds, using mean answers or by voting. Check for The Wisdom of Crowds (Not the TV Show).
answered yesterday
Pedro Henrique MonfortePedro Henrique Monforte
388111
388111
add a comment |
add a comment |
JoschJava is a new contributor. Be nice, and check out our Code of Conduct.
JoschJava is a new contributor. Be nice, and check out our Code of Conduct.
JoschJava is a new contributor. Be nice, and check out our Code of Conduct.
JoschJava is a new contributor. Be nice, and check out our Code of Conduct.
Thanks for contributing an answer to Data Science Stack Exchange!
- Please be sure to answer the question. Provide details and share your research!
But avoid …
- Asking for help, clarification, or responding to other answers.
- Making statements based on opinion; back them up with references or personal experience.
Use MathJax to format equations. MathJax reference.
To learn more, see our tips on writing great answers.
Sign up or log in
StackExchange.ready(function () {
StackExchange.helpers.onClickDraftSave('#login-link');
});
Sign up using Google
Sign up using Facebook
Sign up using Email and Password
Post as a guest
Required, but never shown
StackExchange.ready(
function () {
StackExchange.openid.initPostLogin('.new-post-login', 'https%3a%2f%2fdatascience.stackexchange.com%2fquestions%2f48966%2fstory-tag-prediction-optional-labels%23new-answer', 'question_page');
}
);
Post as a guest
Required, but never shown
Sign up or log in
StackExchange.ready(function () {
StackExchange.helpers.onClickDraftSave('#login-link');
});
Sign up using Google
Sign up using Facebook
Sign up using Email and Password
Post as a guest
Required, but never shown
Sign up or log in
StackExchange.ready(function () {
StackExchange.helpers.onClickDraftSave('#login-link');
});
Sign up using Google
Sign up using Facebook
Sign up using Email and Password
Post as a guest
Required, but never shown
Sign up or log in
StackExchange.ready(function () {
StackExchange.helpers.onClickDraftSave('#login-link');
});
Sign up using Google
Sign up using Facebook
Sign up using Email and Password
Sign up using Google
Sign up using Facebook
Sign up using Email and Password
Post as a guest
Required, but never shown
Required, but never shown
Required, but never shown
Required, but never shown
Required, but never shown
Required, but never shown
Required, but never shown
Required, but never shown
Required, but never shown
Id C 1t0gwgDG
$begingroup$
Are you aiming at multi-label classification?
$endgroup$
– pythinker
yesterday