Random Forests Feature Selection on Time Series Data
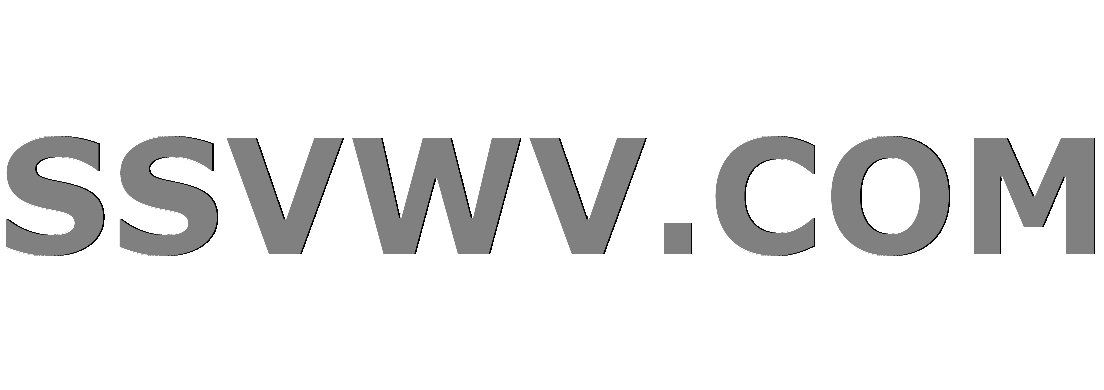
Multi tool use
$begingroup$
I have a dataset with N amount of features, each one with 500 instances in time.
Let's say that I have for example, the features x, y, v_x, v_y, a_x, a_y, j_x, j_y
. In one sample I have 500 instances (rows in a table), for each feature. In another sample, I got other 500 instances, and a class.
I'd like to select a subset of the features automatically with the Random Forests algorithm. The problem is that the algorithm (I'm using ScikitLearn, RandomForestClassifier), accepts a matrix (2D array) as X input, of size [N_samples, N_features]. If I give the array as it is, that is a vector (len 500) for the feature x
, another (len 500) for the feature y
, etc., I get a N_samples x N_features x 500 array, which is incompatible with the requirements of RandomForestClassifier.
I tried to unroll the matrix in a vector, like having so 500 x N_features array, but in that way, in the reduction, it considers all the elements independent feature, and breaks my structure.
How can I reduce the features (by selection) (possibly using this algorithm, but open to other libraries and/or algorithms) keeping the time instances consistent?
My goal is to do classification, so forecasting resources are limitedly useful to me. Also I have the requirement that each sample has those occurrences, and I don't have them as separate samples unfortunately.
python scikit-learn time-series feature-selection random-forest
New contributor
user1714647 is a new contributor to this site. Take care in asking for clarification, commenting, and answering.
Check out our Code of Conduct.
$endgroup$
add a comment |
$begingroup$
I have a dataset with N amount of features, each one with 500 instances in time.
Let's say that I have for example, the features x, y, v_x, v_y, a_x, a_y, j_x, j_y
. In one sample I have 500 instances (rows in a table), for each feature. In another sample, I got other 500 instances, and a class.
I'd like to select a subset of the features automatically with the Random Forests algorithm. The problem is that the algorithm (I'm using ScikitLearn, RandomForestClassifier), accepts a matrix (2D array) as X input, of size [N_samples, N_features]. If I give the array as it is, that is a vector (len 500) for the feature x
, another (len 500) for the feature y
, etc., I get a N_samples x N_features x 500 array, which is incompatible with the requirements of RandomForestClassifier.
I tried to unroll the matrix in a vector, like having so 500 x N_features array, but in that way, in the reduction, it considers all the elements independent feature, and breaks my structure.
How can I reduce the features (by selection) (possibly using this algorithm, but open to other libraries and/or algorithms) keeping the time instances consistent?
My goal is to do classification, so forecasting resources are limitedly useful to me. Also I have the requirement that each sample has those occurrences, and I don't have them as separate samples unfortunately.
python scikit-learn time-series feature-selection random-forest
New contributor
user1714647 is a new contributor to this site. Take care in asking for clarification, commenting, and answering.
Check out our Code of Conduct.
$endgroup$
$begingroup$
Welcome to this site! If you want to treat 500 values per feature as "all or nothing", i.e. not breaking the structure, one way is to use the average for each feature thus reducing 500 to 1.
$endgroup$
– Esmailian
1 hour ago
$begingroup$
But the features bring a semantic which kind of gets lost if I just do the average. But I tried a similar thing. I ran the DTW distance for each feature against the a feature-sequence of a target sample (avg of 3-4 target samples), where target's class is the one of active classes (in binarized comparison, one vs all other classes), and still no success. On the class I'm interested I get up to 0.50 precision and 1.00 recall, if I take out the difficult class out, less if I have it
$endgroup$
– user1714647
1 hour ago
add a comment |
$begingroup$
I have a dataset with N amount of features, each one with 500 instances in time.
Let's say that I have for example, the features x, y, v_x, v_y, a_x, a_y, j_x, j_y
. In one sample I have 500 instances (rows in a table), for each feature. In another sample, I got other 500 instances, and a class.
I'd like to select a subset of the features automatically with the Random Forests algorithm. The problem is that the algorithm (I'm using ScikitLearn, RandomForestClassifier), accepts a matrix (2D array) as X input, of size [N_samples, N_features]. If I give the array as it is, that is a vector (len 500) for the feature x
, another (len 500) for the feature y
, etc., I get a N_samples x N_features x 500 array, which is incompatible with the requirements of RandomForestClassifier.
I tried to unroll the matrix in a vector, like having so 500 x N_features array, but in that way, in the reduction, it considers all the elements independent feature, and breaks my structure.
How can I reduce the features (by selection) (possibly using this algorithm, but open to other libraries and/or algorithms) keeping the time instances consistent?
My goal is to do classification, so forecasting resources are limitedly useful to me. Also I have the requirement that each sample has those occurrences, and I don't have them as separate samples unfortunately.
python scikit-learn time-series feature-selection random-forest
New contributor
user1714647 is a new contributor to this site. Take care in asking for clarification, commenting, and answering.
Check out our Code of Conduct.
$endgroup$
I have a dataset with N amount of features, each one with 500 instances in time.
Let's say that I have for example, the features x, y, v_x, v_y, a_x, a_y, j_x, j_y
. In one sample I have 500 instances (rows in a table), for each feature. In another sample, I got other 500 instances, and a class.
I'd like to select a subset of the features automatically with the Random Forests algorithm. The problem is that the algorithm (I'm using ScikitLearn, RandomForestClassifier), accepts a matrix (2D array) as X input, of size [N_samples, N_features]. If I give the array as it is, that is a vector (len 500) for the feature x
, another (len 500) for the feature y
, etc., I get a N_samples x N_features x 500 array, which is incompatible with the requirements of RandomForestClassifier.
I tried to unroll the matrix in a vector, like having so 500 x N_features array, but in that way, in the reduction, it considers all the elements independent feature, and breaks my structure.
How can I reduce the features (by selection) (possibly using this algorithm, but open to other libraries and/or algorithms) keeping the time instances consistent?
My goal is to do classification, so forecasting resources are limitedly useful to me. Also I have the requirement that each sample has those occurrences, and I don't have them as separate samples unfortunately.
python scikit-learn time-series feature-selection random-forest
python scikit-learn time-series feature-selection random-forest
New contributor
user1714647 is a new contributor to this site. Take care in asking for clarification, commenting, and answering.
Check out our Code of Conduct.
New contributor
user1714647 is a new contributor to this site. Take care in asking for clarification, commenting, and answering.
Check out our Code of Conduct.
New contributor
user1714647 is a new contributor to this site. Take care in asking for clarification, commenting, and answering.
Check out our Code of Conduct.
asked 2 hours ago
user1714647user1714647
101
101
New contributor
user1714647 is a new contributor to this site. Take care in asking for clarification, commenting, and answering.
Check out our Code of Conduct.
New contributor
user1714647 is a new contributor to this site. Take care in asking for clarification, commenting, and answering.
Check out our Code of Conduct.
user1714647 is a new contributor to this site. Take care in asking for clarification, commenting, and answering.
Check out our Code of Conduct.
$begingroup$
Welcome to this site! If you want to treat 500 values per feature as "all or nothing", i.e. not breaking the structure, one way is to use the average for each feature thus reducing 500 to 1.
$endgroup$
– Esmailian
1 hour ago
$begingroup$
But the features bring a semantic which kind of gets lost if I just do the average. But I tried a similar thing. I ran the DTW distance for each feature against the a feature-sequence of a target sample (avg of 3-4 target samples), where target's class is the one of active classes (in binarized comparison, one vs all other classes), and still no success. On the class I'm interested I get up to 0.50 precision and 1.00 recall, if I take out the difficult class out, less if I have it
$endgroup$
– user1714647
1 hour ago
add a comment |
$begingroup$
Welcome to this site! If you want to treat 500 values per feature as "all or nothing", i.e. not breaking the structure, one way is to use the average for each feature thus reducing 500 to 1.
$endgroup$
– Esmailian
1 hour ago
$begingroup$
But the features bring a semantic which kind of gets lost if I just do the average. But I tried a similar thing. I ran the DTW distance for each feature against the a feature-sequence of a target sample (avg of 3-4 target samples), where target's class is the one of active classes (in binarized comparison, one vs all other classes), and still no success. On the class I'm interested I get up to 0.50 precision and 1.00 recall, if I take out the difficult class out, less if I have it
$endgroup$
– user1714647
1 hour ago
$begingroup$
Welcome to this site! If you want to treat 500 values per feature as "all or nothing", i.e. not breaking the structure, one way is to use the average for each feature thus reducing 500 to 1.
$endgroup$
– Esmailian
1 hour ago
$begingroup$
Welcome to this site! If you want to treat 500 values per feature as "all or nothing", i.e. not breaking the structure, one way is to use the average for each feature thus reducing 500 to 1.
$endgroup$
– Esmailian
1 hour ago
$begingroup$
But the features bring a semantic which kind of gets lost if I just do the average. But I tried a similar thing. I ran the DTW distance for each feature against the a feature-sequence of a target sample (avg of 3-4 target samples), where target's class is the one of active classes (in binarized comparison, one vs all other classes), and still no success. On the class I'm interested I get up to 0.50 precision and 1.00 recall, if I take out the difficult class out, less if I have it
$endgroup$
– user1714647
1 hour ago
$begingroup$
But the features bring a semantic which kind of gets lost if I just do the average. But I tried a similar thing. I ran the DTW distance for each feature against the a feature-sequence of a target sample (avg of 3-4 target samples), where target's class is the one of active classes (in binarized comparison, one vs all other classes), and still no success. On the class I'm interested I get up to 0.50 precision and 1.00 recall, if I take out the difficult class out, less if I have it
$endgroup$
– user1714647
1 hour ago
add a comment |
0
active
oldest
votes
Your Answer
StackExchange.ifUsing("editor", function () {
return StackExchange.using("mathjaxEditing", function () {
StackExchange.MarkdownEditor.creationCallbacks.add(function (editor, postfix) {
StackExchange.mathjaxEditing.prepareWmdForMathJax(editor, postfix, [["$", "$"], ["\\(","\\)"]]);
});
});
}, "mathjax-editing");
StackExchange.ready(function() {
var channelOptions = {
tags: "".split(" "),
id: "557"
};
initTagRenderer("".split(" "), "".split(" "), channelOptions);
StackExchange.using("externalEditor", function() {
// Have to fire editor after snippets, if snippets enabled
if (StackExchange.settings.snippets.snippetsEnabled) {
StackExchange.using("snippets", function() {
createEditor();
});
}
else {
createEditor();
}
});
function createEditor() {
StackExchange.prepareEditor({
heartbeatType: 'answer',
autoActivateHeartbeat: false,
convertImagesToLinks: false,
noModals: true,
showLowRepImageUploadWarning: true,
reputationToPostImages: null,
bindNavPrevention: true,
postfix: "",
imageUploader: {
brandingHtml: "Powered by u003ca class="icon-imgur-white" href="https://imgur.com/"u003eu003c/au003e",
contentPolicyHtml: "User contributions licensed under u003ca href="https://creativecommons.org/licenses/by-sa/3.0/"u003ecc by-sa 3.0 with attribution requiredu003c/au003e u003ca href="https://stackoverflow.com/legal/content-policy"u003e(content policy)u003c/au003e",
allowUrls: true
},
onDemand: true,
discardSelector: ".discard-answer"
,immediatelyShowMarkdownHelp:true
});
}
});
user1714647 is a new contributor. Be nice, and check out our Code of Conduct.
Sign up or log in
StackExchange.ready(function () {
StackExchange.helpers.onClickDraftSave('#login-link');
});
Sign up using Google
Sign up using Facebook
Sign up using Email and Password
Post as a guest
Required, but never shown
StackExchange.ready(
function () {
StackExchange.openid.initPostLogin('.new-post-login', 'https%3a%2f%2fdatascience.stackexchange.com%2fquestions%2f48183%2frandom-forests-feature-selection-on-time-series-data%23new-answer', 'question_page');
}
);
Post as a guest
Required, but never shown
0
active
oldest
votes
0
active
oldest
votes
active
oldest
votes
active
oldest
votes
user1714647 is a new contributor. Be nice, and check out our Code of Conduct.
user1714647 is a new contributor. Be nice, and check out our Code of Conduct.
user1714647 is a new contributor. Be nice, and check out our Code of Conduct.
user1714647 is a new contributor. Be nice, and check out our Code of Conduct.
Thanks for contributing an answer to Data Science Stack Exchange!
- Please be sure to answer the question. Provide details and share your research!
But avoid …
- Asking for help, clarification, or responding to other answers.
- Making statements based on opinion; back them up with references or personal experience.
Use MathJax to format equations. MathJax reference.
To learn more, see our tips on writing great answers.
Sign up or log in
StackExchange.ready(function () {
StackExchange.helpers.onClickDraftSave('#login-link');
});
Sign up using Google
Sign up using Facebook
Sign up using Email and Password
Post as a guest
Required, but never shown
StackExchange.ready(
function () {
StackExchange.openid.initPostLogin('.new-post-login', 'https%3a%2f%2fdatascience.stackexchange.com%2fquestions%2f48183%2frandom-forests-feature-selection-on-time-series-data%23new-answer', 'question_page');
}
);
Post as a guest
Required, but never shown
Sign up or log in
StackExchange.ready(function () {
StackExchange.helpers.onClickDraftSave('#login-link');
});
Sign up using Google
Sign up using Facebook
Sign up using Email and Password
Post as a guest
Required, but never shown
Sign up or log in
StackExchange.ready(function () {
StackExchange.helpers.onClickDraftSave('#login-link');
});
Sign up using Google
Sign up using Facebook
Sign up using Email and Password
Post as a guest
Required, but never shown
Sign up or log in
StackExchange.ready(function () {
StackExchange.helpers.onClickDraftSave('#login-link');
});
Sign up using Google
Sign up using Facebook
Sign up using Email and Password
Sign up using Google
Sign up using Facebook
Sign up using Email and Password
Post as a guest
Required, but never shown
Required, but never shown
Required, but never shown
Required, but never shown
Required, but never shown
Required, but never shown
Required, but never shown
Required, but never shown
Required, but never shown
P LzB6 hET,DxN,fhC QL,W,I,NdUGsShM4Md7F AEWnJ2SsY8uxddD T,eMAxiTw,hjGzQ7AE8e DCx,vmIT13 A7 JDMLY,vXHs
$begingroup$
Welcome to this site! If you want to treat 500 values per feature as "all or nothing", i.e. not breaking the structure, one way is to use the average for each feature thus reducing 500 to 1.
$endgroup$
– Esmailian
1 hour ago
$begingroup$
But the features bring a semantic which kind of gets lost if I just do the average. But I tried a similar thing. I ran the DTW distance for each feature against the a feature-sequence of a target sample (avg of 3-4 target samples), where target's class is the one of active classes (in binarized comparison, one vs all other classes), and still no success. On the class I'm interested I get up to 0.50 precision and 1.00 recall, if I take out the difficult class out, less if I have it
$endgroup$
– user1714647
1 hour ago