Is reward accumulated during a play iteration when performing SARSA?
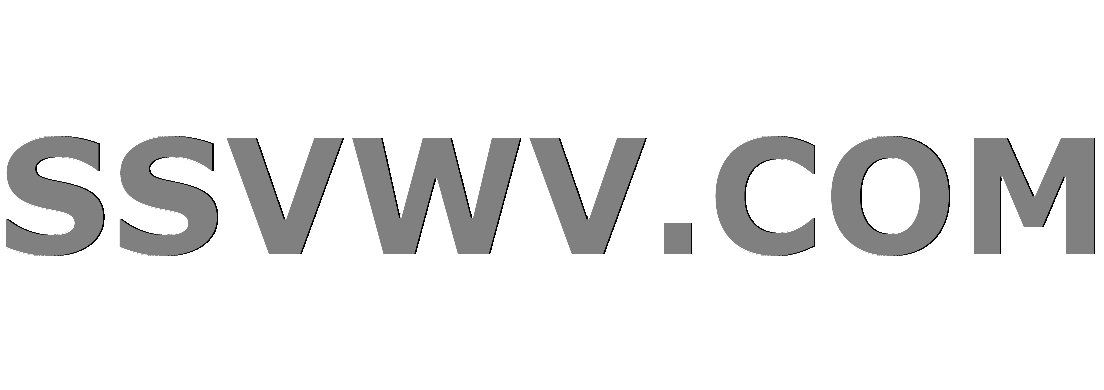
Multi tool use
$begingroup$
I've been having issue with getting my DQN to converge to a good solution for snake. Regardless of the different types of reward functions I've tried, it seems that the snake is indefinitely going around in circles. I have not tried exploring more states yet because I am confused about how to properly assign reward.
Currently, I am using a 2D-Gaussian distribution to assign reward where $f(x=x_{food},y=y_{food}) = 1$. Terminal states like hitting the wall or itself result in a reward value of -1.
My reason for using the Gaussian was because of the relatively sparse rewards in this game and the ability to easily clip rewards between [1,-1] in meaning full way.
I have two questions.
- Is this an appropriate way to define the reward function?
- Currently during training, I do no accumulate the reward during each play iteration. So each reward for transitions are independent of the reward values before it. Right now I am doing $big[S_1,A_1,R_1,S_2,A_2,R_2,S_3big]$. I've looked at other code where people have accumulate the reward like $big[S_1,A_1,R_1,S_2,A_2,R_2 = R_1+r,S_3big]$. Where $r$ is given by the reward function. The thing is, I can't find a paper that defines why you should do this. So my question is, which way is the appropriate way to assign reward?
machine-learning deep-learning reinforcement-learning q-learning dqn
$endgroup$
add a comment |
$begingroup$
I've been having issue with getting my DQN to converge to a good solution for snake. Regardless of the different types of reward functions I've tried, it seems that the snake is indefinitely going around in circles. I have not tried exploring more states yet because I am confused about how to properly assign reward.
Currently, I am using a 2D-Gaussian distribution to assign reward where $f(x=x_{food},y=y_{food}) = 1$. Terminal states like hitting the wall or itself result in a reward value of -1.
My reason for using the Gaussian was because of the relatively sparse rewards in this game and the ability to easily clip rewards between [1,-1] in meaning full way.
I have two questions.
- Is this an appropriate way to define the reward function?
- Currently during training, I do no accumulate the reward during each play iteration. So each reward for transitions are independent of the reward values before it. Right now I am doing $big[S_1,A_1,R_1,S_2,A_2,R_2,S_3big]$. I've looked at other code where people have accumulate the reward like $big[S_1,A_1,R_1,S_2,A_2,R_2 = R_1+r,S_3big]$. Where $r$ is given by the reward function. The thing is, I can't find a paper that defines why you should do this. So my question is, which way is the appropriate way to assign reward?
machine-learning deep-learning reinforcement-learning q-learning dqn
$endgroup$
add a comment |
$begingroup$
I've been having issue with getting my DQN to converge to a good solution for snake. Regardless of the different types of reward functions I've tried, it seems that the snake is indefinitely going around in circles. I have not tried exploring more states yet because I am confused about how to properly assign reward.
Currently, I am using a 2D-Gaussian distribution to assign reward where $f(x=x_{food},y=y_{food}) = 1$. Terminal states like hitting the wall or itself result in a reward value of -1.
My reason for using the Gaussian was because of the relatively sparse rewards in this game and the ability to easily clip rewards between [1,-1] in meaning full way.
I have two questions.
- Is this an appropriate way to define the reward function?
- Currently during training, I do no accumulate the reward during each play iteration. So each reward for transitions are independent of the reward values before it. Right now I am doing $big[S_1,A_1,R_1,S_2,A_2,R_2,S_3big]$. I've looked at other code where people have accumulate the reward like $big[S_1,A_1,R_1,S_2,A_2,R_2 = R_1+r,S_3big]$. Where $r$ is given by the reward function. The thing is, I can't find a paper that defines why you should do this. So my question is, which way is the appropriate way to assign reward?
machine-learning deep-learning reinforcement-learning q-learning dqn
$endgroup$
I've been having issue with getting my DQN to converge to a good solution for snake. Regardless of the different types of reward functions I've tried, it seems that the snake is indefinitely going around in circles. I have not tried exploring more states yet because I am confused about how to properly assign reward.
Currently, I am using a 2D-Gaussian distribution to assign reward where $f(x=x_{food},y=y_{food}) = 1$. Terminal states like hitting the wall or itself result in a reward value of -1.
My reason for using the Gaussian was because of the relatively sparse rewards in this game and the ability to easily clip rewards between [1,-1] in meaning full way.
I have two questions.
- Is this an appropriate way to define the reward function?
- Currently during training, I do no accumulate the reward during each play iteration. So each reward for transitions are independent of the reward values before it. Right now I am doing $big[S_1,A_1,R_1,S_2,A_2,R_2,S_3big]$. I've looked at other code where people have accumulate the reward like $big[S_1,A_1,R_1,S_2,A_2,R_2 = R_1+r,S_3big]$. Where $r$ is given by the reward function. The thing is, I can't find a paper that defines why you should do this. So my question is, which way is the appropriate way to assign reward?
machine-learning deep-learning reinforcement-learning q-learning dqn
machine-learning deep-learning reinforcement-learning q-learning dqn
asked 18 mins ago
DevarakondaVDevarakondaV
163
163
add a comment |
add a comment |
0
active
oldest
votes
Your Answer
StackExchange.ifUsing("editor", function () {
return StackExchange.using("mathjaxEditing", function () {
StackExchange.MarkdownEditor.creationCallbacks.add(function (editor, postfix) {
StackExchange.mathjaxEditing.prepareWmdForMathJax(editor, postfix, [["$", "$"], ["\\(","\\)"]]);
});
});
}, "mathjax-editing");
StackExchange.ready(function() {
var channelOptions = {
tags: "".split(" "),
id: "557"
};
initTagRenderer("".split(" "), "".split(" "), channelOptions);
StackExchange.using("externalEditor", function() {
// Have to fire editor after snippets, if snippets enabled
if (StackExchange.settings.snippets.snippetsEnabled) {
StackExchange.using("snippets", function() {
createEditor();
});
}
else {
createEditor();
}
});
function createEditor() {
StackExchange.prepareEditor({
heartbeatType: 'answer',
autoActivateHeartbeat: false,
convertImagesToLinks: false,
noModals: true,
showLowRepImageUploadWarning: true,
reputationToPostImages: null,
bindNavPrevention: true,
postfix: "",
imageUploader: {
brandingHtml: "Powered by u003ca class="icon-imgur-white" href="https://imgur.com/"u003eu003c/au003e",
contentPolicyHtml: "User contributions licensed under u003ca href="https://creativecommons.org/licenses/by-sa/3.0/"u003ecc by-sa 3.0 with attribution requiredu003c/au003e u003ca href="https://stackoverflow.com/legal/content-policy"u003e(content policy)u003c/au003e",
allowUrls: true
},
onDemand: true,
discardSelector: ".discard-answer"
,immediatelyShowMarkdownHelp:true
});
}
});
Sign up or log in
StackExchange.ready(function () {
StackExchange.helpers.onClickDraftSave('#login-link');
});
Sign up using Google
Sign up using Facebook
Sign up using Email and Password
Post as a guest
Required, but never shown
StackExchange.ready(
function () {
StackExchange.openid.initPostLogin('.new-post-login', 'https%3a%2f%2fdatascience.stackexchange.com%2fquestions%2f48186%2fis-reward-accumulated-during-a-play-iteration-when-performing-sarsa%23new-answer', 'question_page');
}
);
Post as a guest
Required, but never shown
0
active
oldest
votes
0
active
oldest
votes
active
oldest
votes
active
oldest
votes
Thanks for contributing an answer to Data Science Stack Exchange!
- Please be sure to answer the question. Provide details and share your research!
But avoid …
- Asking for help, clarification, or responding to other answers.
- Making statements based on opinion; back them up with references or personal experience.
Use MathJax to format equations. MathJax reference.
To learn more, see our tips on writing great answers.
Sign up or log in
StackExchange.ready(function () {
StackExchange.helpers.onClickDraftSave('#login-link');
});
Sign up using Google
Sign up using Facebook
Sign up using Email and Password
Post as a guest
Required, but never shown
StackExchange.ready(
function () {
StackExchange.openid.initPostLogin('.new-post-login', 'https%3a%2f%2fdatascience.stackexchange.com%2fquestions%2f48186%2fis-reward-accumulated-during-a-play-iteration-when-performing-sarsa%23new-answer', 'question_page');
}
);
Post as a guest
Required, but never shown
Sign up or log in
StackExchange.ready(function () {
StackExchange.helpers.onClickDraftSave('#login-link');
});
Sign up using Google
Sign up using Facebook
Sign up using Email and Password
Post as a guest
Required, but never shown
Sign up or log in
StackExchange.ready(function () {
StackExchange.helpers.onClickDraftSave('#login-link');
});
Sign up using Google
Sign up using Facebook
Sign up using Email and Password
Post as a guest
Required, but never shown
Sign up or log in
StackExchange.ready(function () {
StackExchange.helpers.onClickDraftSave('#login-link');
});
Sign up using Google
Sign up using Facebook
Sign up using Email and Password
Sign up using Google
Sign up using Facebook
Sign up using Email and Password
Post as a guest
Required, but never shown
Required, but never shown
Required, but never shown
Required, but never shown
Required, but never shown
Required, but never shown
Required, but never shown
Required, but never shown
Required, but never shown
1aZfDCUJtJsZyvJf vnGUOa,N7,VUISoN oPP