Multiclass Segmentation Using U-Net: My training loss is not decreasing after certain epoch (accuracy not...
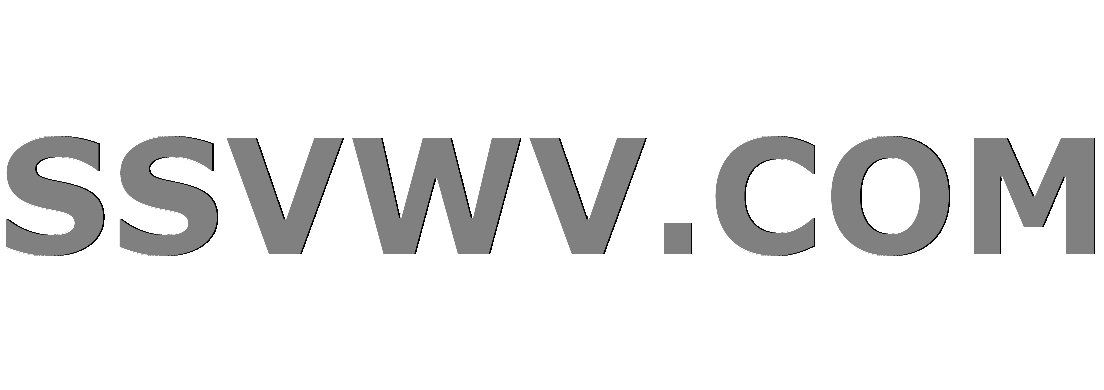
Multi tool use
$begingroup$
So the problem is to perform a multiclass segmentation (255 classes of crops), and I am using a U-Net model for that. The input images are grayscale and the images of dimensions (128,128,1) are grouped into 7 groups (that is 7 channels). The output images are segmented images (128,128,1).
Approach: So I created a dataset for segmentation. Since the input images are to be grouped in 7, I created an input tensor (128,128,7) and the output tensor as (128,128,255) since there are 255 classes and we need this segmentation.
Number of Input:11151 (1593*7) images
Outputs: 1593 images
First I mounted my drive on google colab:
from google.colab import drive
drive.mount('/content/drive')
Go to this URL in a browser: https://accounts.google.com/o/oauth2/auth?client_id=947318989803-6bn6qk8qdgf4n4g3pfee6491hc0brc4i.apps.googleusercontent.com&redirect_uri=urn%3Aietf%3Awg%3Aoauth%3A2.0%3Aoob&scope=email%20https%3A%2F%2Fwww.googleapis.com%2Fauth%2Fdocs.test%20https%3A%2F%2Fwww.googleapis.com%2Fauth%2Fdrive%20https%3A%2F%2Fwww.googleapis.com%2Fauth%2Fdrive.photos.readonly%20https%3A%2F%2Fwww.googleapis.com%2Fauth%2Fpeopleapi.readonly&response_type=code
Enter your authorization code:
··········
Mounted at /content/drive
Then I loaded the libraries:
import os
import random
import pandas as pd
import numpy as np
import matplotlib.pyplot as plt
plt.style.use("ggplot")
%matplotlib inline
from itertools import chain
from skimage.io import imread, imshow, concatenate_images
from skimage.transform import resize
from skimage.morphology import label
from sklearn.model_selection import train_test_split
import tensorflow as tf
from keras.models import Model, load_model
from keras.layers import Input, BatchNormalization, Activation, Dense, Dropout
from keras.layers.core import Lambda, RepeatVector, Reshape
from keras.layers.convolutional import Conv2D, Conv2DTranspose
from keras.layers.pooling import MaxPooling2D, GlobalMaxPool2D
from keras.layers.merge import concatenate, add
from keras.callbacks import EarlyStopping, ModelCheckpoint, ReduceLROnPlateau
from keras.optimizers import Adam,SGD
from keras.preprocessing.image import ImageDataGenerator, array_to_img, img_to_array, load_img
from keras.layers import LeakyReLU
Then I divieded the dataset into training and validation:
im_width = 128
im_height = 128
border = 5
path_train_input = '/content/drive/path....'
path_train_output = '/content/drive/path..'
ids = next(os.walk(path_train_output))[2]
print(len(ids))
from random import shuffle
shuffle(ids)
train_ids = ids[0:1350]
valid_ids = ids[1350:]
print(len(train_ids))
I created this function to load the data in batches to avoid memory crashes in colab:
def get_data(ids,batch_size):
while True:
ids_batches = [ids[i:min(i+batch_size,len(ids))] for i in range(0, len(ids), batch_size)]
#ids_out_batches = [ids_out[j:min(j+batch_size,len(ids_out))] for j in range(0, len(ids_out), batch_size)]
# Load images
for b in range(len(ids_batches)):
k=-1
X = np.zeros((len(ids_batches[b]), im_height, im_width, 7), dtype=np.float32)
y = np.zeros((len(ids_batches[b]), im_height, im_width, 255), dtype=np.float32)
for c in range(len(ids_batches[b])):
k=k+1
#temp1=np.zeros((1,7),dtype=np.float32)
for r in range(1,8):
img = load_img(path_train_input + 'lc8' + ids_batches[b][c][3:-4] + '_' + str(r) + '.tif', color_mode="grayscale")
x_img = img_to_array(img)
x_img = resize(x_img, (128, 128), mode='constant', preserve_range=True)
for p in range(128):
for q in range(128):
X[k][p][q][r-1]=x_img[p][q]/255
#k=k+1
# Save images
#X[k, ..., 0] = temp1 / 255
# Load masks
mask = img_to_array(load_img(path_train_output+ids_batches[b][c], color_mode="grayscale"))
mask = resize(mask, (128, 128), mode='constant', preserve_range=True)
for p in range(128):
for q in range(128):
num=int(mask[p][q])
temp=np.zeros((255), dtype=np.float32)
temp[num]=1
y[k][p][q]=temp
yield X,y
Then I started creating my model:
def conv2d_block(input_tensor, n_filters, kernel_size=3, batchnorm=True):
# first layer
x = Conv2D(filters=n_filters, kernel_size=(kernel_size, kernel_size), kernel_initializer="he_normal",
padding="same")(input_tensor)
if batchnorm:
x = BatchNormalization()(x)
x = LeakyReLU(alpha=0.3)(x)
# second layer
x = Conv2D(filters=n_filters, kernel_size=(kernel_size, kernel_size), kernel_initializer="he_normal",
padding="same")(x)
if batchnorm:
x = BatchNormalization()(x)
x = LeakyReLU(alpha=0.3)(x)
return x
Then the U-Net:
def get_unet(input_img, n_filters=16, dropout=0.15, batchnorm=True):
# contracting path
c1 = conv2d_block(input_img, n_filters=n_filters*1, kernel_size=3, batchnorm=batchnorm)
p1 = MaxPooling2D((2, 2)) (c1)
p1 = Dropout(rate=dropout*0.5)(p1)
c2 = conv2d_block(p1, n_filters=n_filters*2, kernel_size=3, batchnorm=batchnorm)
p2 = MaxPooling2D((2, 2)) (c2)
p2 = Dropout(rate=dropout)(p2)
c3 = conv2d_block(p2, n_filters=n_filters*4, kernel_size=3, batchnorm=batchnorm)
p3 = MaxPooling2D((2, 2)) (c3)
p3 = Dropout(rate=dropout)(p3)
c4 = conv2d_block(p3, n_filters=n_filters*8, kernel_size=3, batchnorm=batchnorm)
p4 = MaxPooling2D(pool_size=(2, 2)) (c4)
p4 = Dropout(rate=dropout)(p4)
c5 = conv2d_block(p4, n_filters=n_filters*16, kernel_size=3, batchnorm=batchnorm)
# expansive path
u6 = Conv2DTranspose(n_filters*8, (3, 3), strides=(2, 2), padding='same') (c5)
u6 = concatenate([u6, c4])
u6 = Dropout(rate=dropout)(u6)
c6 = conv2d_block(u6, n_filters=n_filters*8, kernel_size=3, batchnorm=batchnorm)
u7 = Conv2DTranspose(n_filters*4, (3, 3), strides=(2, 2), padding='same') (c6)
u7 = concatenate([u7, c3])
u7 = Dropout(rate=dropout)(u7)
c7 = conv2d_block(u7, n_filters=n_filters*4, kernel_size=3, batchnorm=batchnorm)
u8 = Conv2DTranspose(n_filters*2, (3, 3), strides=(2, 2), padding='same') (c7)
u8 = concatenate([u8, c2])
u8 = Dropout(rate=dropout)(u8)
c8 = conv2d_block(u8, n_filters=n_filters*2, kernel_size=3, batchnorm=batchnorm)
u9 = Conv2DTranspose(n_filters*1, (3, 3), strides=(2, 2), padding='same') (c8)
u9 = concatenate([u9, c1], axis=3)
u9 = Dropout(rate=dropout)(u9)
c9 = conv2d_block(u9, n_filters=n_filters*1, kernel_size=3, batchnorm=batchnorm)
c10 = conv2d_block(c9, n_filters=255, kernel_size=1, batchnorm=batchnorm)
#conv6 = core.Reshape((1,128,128))(c9)
#conv6 = core.Permute((2,1))(conv6)
outputs = Conv2D(255, (1, 1), activation='softmax') (c10)
model = Model(inputs=[input_img], outputs=[outputs])
return model
input_img = Input((im_height, im_width, 7), name='img')
model = get_unet(input_img, n_filters=16, dropout=0.15, batchnorm=True)
#model = get_unet()
model.compile(optimizer=Adam(lr=0.01), loss="categorical_crossentropy", metrics=["accuracy"])
model.summary()
As you can see in the model summary input is (128,128,7) and output (128,128,255):
__________________________________________________________________________________________________
Layer (type) Output Shape Param # Connected to
==================================================================================================
img (InputLayer) (None, 128, 128, 7) 0
__________________________________________________________________________________________________
conv2d_51 (Conv2D) (None, 128, 128, 16) 1024 img[0][0]
__________________________________________________________________________________________________
batch_normalization_49 (BatchNo (None, 128, 128, 16) 64 conv2d_51[0][0]
__________________________________________________________________________________________________
leaky_re_lu_49 (LeakyReLU) (None, 128, 128, 16) 0 batch_normalization_49[0][0]
__________________________________________________________________________________________________
conv2d_52 (Conv2D) (None, 128, 128, 16) 2320 leaky_re_lu_49[0][0]
__________________________________________________________________________________________________
batch_normalization_50 (BatchNo (None, 128, 128, 16) 64 conv2d_52[0][0]
__________________________________________________________________________________________________
leaky_re_lu_50 (LeakyReLU) (None, 128, 128, 16) 0 batch_normalization_50[0][0]
__________________________________________________________________________________________________
max_pooling2d_11 (MaxPooling2D) (None, 64, 64, 16) 0 leaky_re_lu_50[0][0]
__________________________________________________________________________________________________
dropout_21 (Dropout) (None, 64, 64, 16) 0 max_pooling2d_11[0][0]
__________________________________________________________________________________________________
conv2d_53 (Conv2D) (None, 64, 64, 32) 4640 dropout_21[0][0]
__________________________________________________________________________________________________
batch_normalization_51 (BatchNo (None, 64, 64, 32) 128 conv2d_53[0][0]
__________________________________________________________________________________________________
leaky_re_lu_51 (LeakyReLU) (None, 64, 64, 32) 0 batch_normalization_51[0][0]
__________________________________________________________________________________________________
conv2d_54 (Conv2D) (None, 64, 64, 32) 9248 leaky_re_lu_51[0][0]
__________________________________________________________________________________________________
batch_normalization_52 (BatchNo (None, 64, 64, 32) 128 conv2d_54[0][0]
__________________________________________________________________________________________________
leaky_re_lu_52 (LeakyReLU) (None, 64, 64, 32) 0 batch_normalization_52[0][0]
__________________________________________________________________________________________________
max_pooling2d_12 (MaxPooling2D) (None, 32, 32, 32) 0 leaky_re_lu_52[0][0]
__________________________________________________________________________________________________
dropout_22 (Dropout) (None, 32, 32, 32) 0 max_pooling2d_12[0][0]
__________________________________________________________________________________________________
conv2d_55 (Conv2D) (None, 32, 32, 64) 18496 dropout_22[0][0]
__________________________________________________________________________________________________
batch_normalization_53 (BatchNo (None, 32, 32, 64) 256 conv2d_55[0][0]
__________________________________________________________________________________________________
leaky_re_lu_53 (LeakyReLU) (None, 32, 32, 64) 0 batch_normalization_53[0][0]
__________________________________________________________________________________________________
conv2d_56 (Conv2D) (None, 32, 32, 64) 36928 leaky_re_lu_53[0][0]
__________________________________________________________________________________________________
batch_normalization_54 (BatchNo (None, 32, 32, 64) 256 conv2d_56[0][0]
__________________________________________________________________________________________________
leaky_re_lu_54 (LeakyReLU) (None, 32, 32, 64) 0 batch_normalization_54[0][0]
__________________________________________________________________________________________________
max_pooling2d_13 (MaxPooling2D) (None, 16, 16, 64) 0 leaky_re_lu_54[0][0]
__________________________________________________________________________________________________
dropout_23 (Dropout) (None, 16, 16, 64) 0 max_pooling2d_13[0][0]
__________________________________________________________________________________________________
conv2d_57 (Conv2D) (None, 16, 16, 128) 73856 dropout_23[0][0]
__________________________________________________________________________________________________
batch_normalization_55 (BatchNo (None, 16, 16, 128) 512 conv2d_57[0][0]
__________________________________________________________________________________________________
leaky_re_lu_55 (LeakyReLU) (None, 16, 16, 128) 0 batch_normalization_55[0][0]
__________________________________________________________________________________________________
conv2d_58 (Conv2D) (None, 16, 16, 128) 147584 leaky_re_lu_55[0][0]
__________________________________________________________________________________________________
batch_normalization_56 (BatchNo (None, 16, 16, 128) 512 conv2d_58[0][0]
__________________________________________________________________________________________________
leaky_re_lu_56 (LeakyReLU) (None, 16, 16, 128) 0 batch_normalization_56[0][0]
__________________________________________________________________________________________________
max_pooling2d_14 (MaxPooling2D) (None, 8, 8, 128) 0 leaky_re_lu_56[0][0]
__________________________________________________________________________________________________
dropout_24 (Dropout) (None, 8, 8, 128) 0 max_pooling2d_14[0][0]
__________________________________________________________________________________________________
conv2d_59 (Conv2D) (None, 8, 8, 256) 295168 dropout_24[0][0]
__________________________________________________________________________________________________
batch_normalization_57 (BatchNo (None, 8, 8, 256) 1024 conv2d_59[0][0]
__________________________________________________________________________________________________
leaky_re_lu_57 (LeakyReLU) (None, 8, 8, 256) 0 batch_normalization_57[0][0]
__________________________________________________________________________________________________
conv2d_60 (Conv2D) (None, 8, 8, 256) 590080 leaky_re_lu_57[0][0]
__________________________________________________________________________________________________
batch_normalization_58 (BatchNo (None, 8, 8, 256) 1024 conv2d_60[0][0]
__________________________________________________________________________________________________
leaky_re_lu_58 (LeakyReLU) (None, 8, 8, 256) 0 batch_normalization_58[0][0]
__________________________________________________________________________________________________
conv2d_transpose_11 (Conv2DTran (None, 16, 16, 128) 295040 leaky_re_lu_58[0][0]
__________________________________________________________________________________________________
concatenate_11 (Concatenate) (None, 16, 16, 256) 0 conv2d_transpose_11[0][0]
leaky_re_lu_56[0][0]
__________________________________________________________________________________________________
dropout_25 (Dropout) (None, 16, 16, 256) 0 concatenate_11[0][0]
__________________________________________________________________________________________________
conv2d_61 (Conv2D) (None, 16, 16, 128) 295040 dropout_25[0][0]
__________________________________________________________________________________________________
batch_normalization_59 (BatchNo (None, 16, 16, 128) 512 conv2d_61[0][0]
__________________________________________________________________________________________________
leaky_re_lu_59 (LeakyReLU) (None, 16, 16, 128) 0 batch_normalization_59[0][0]
__________________________________________________________________________________________________
conv2d_62 (Conv2D) (None, 16, 16, 128) 147584 leaky_re_lu_59[0][0]
__________________________________________________________________________________________________
batch_normalization_60 (BatchNo (None, 16, 16, 128) 512 conv2d_62[0][0]
__________________________________________________________________________________________________
leaky_re_lu_60 (LeakyReLU) (None, 16, 16, 128) 0 batch_normalization_60[0][0]
__________________________________________________________________________________________________
conv2d_transpose_12 (Conv2DTran (None, 32, 32, 64) 73792 leaky_re_lu_60[0][0]
__________________________________________________________________________________________________
concatenate_12 (Concatenate) (None, 32, 32, 128) 0 conv2d_transpose_12[0][0]
leaky_re_lu_54[0][0]
__________________________________________________________________________________________________
dropout_26 (Dropout) (None, 32, 32, 128) 0 concatenate_12[0][0]
__________________________________________________________________________________________________
conv2d_63 (Conv2D) (None, 32, 32, 64) 73792 dropout_26[0][0]
__________________________________________________________________________________________________
batch_normalization_61 (BatchNo (None, 32, 32, 64) 256 conv2d_63[0][0]
__________________________________________________________________________________________________
leaky_re_lu_61 (LeakyReLU) (None, 32, 32, 64) 0 batch_normalization_61[0][0]
__________________________________________________________________________________________________
conv2d_64 (Conv2D) (None, 32, 32, 64) 36928 leaky_re_lu_61[0][0]
__________________________________________________________________________________________________
batch_normalization_62 (BatchNo (None, 32, 32, 64) 256 conv2d_64[0][0]
__________________________________________________________________________________________________
leaky_re_lu_62 (LeakyReLU) (None, 32, 32, 64) 0 batch_normalization_62[0][0]
__________________________________________________________________________________________________
conv2d_transpose_13 (Conv2DTran (None, 64, 64, 32) 18464 leaky_re_lu_62[0][0]
__________________________________________________________________________________________________
concatenate_13 (Concatenate) (None, 64, 64, 64) 0 conv2d_transpose_13[0][0]
leaky_re_lu_52[0][0]
__________________________________________________________________________________________________
dropout_27 (Dropout) (None, 64, 64, 64) 0 concatenate_13[0][0]
__________________________________________________________________________________________________
conv2d_65 (Conv2D) (None, 64, 64, 32) 18464 dropout_27[0][0]
__________________________________________________________________________________________________
batch_normalization_63 (BatchNo (None, 64, 64, 32) 128 conv2d_65[0][0]
__________________________________________________________________________________________________
leaky_re_lu_63 (LeakyReLU) (None, 64, 64, 32) 0 batch_normalization_63[0][0]
__________________________________________________________________________________________________
conv2d_66 (Conv2D) (None, 64, 64, 32) 9248 leaky_re_lu_63[0][0]
__________________________________________________________________________________________________
batch_normalization_64 (BatchNo (None, 64, 64, 32) 128 conv2d_66[0][0]
__________________________________________________________________________________________________
leaky_re_lu_64 (LeakyReLU) (None, 64, 64, 32) 0 batch_normalization_64[0][0]
__________________________________________________________________________________________________
conv2d_transpose_14 (Conv2DTran (None, 128, 128, 16) 4624 leaky_re_lu_64[0][0]
__________________________________________________________________________________________________
concatenate_14 (Concatenate) (None, 128, 128, 32) 0 conv2d_transpose_14[0][0]
leaky_re_lu_50[0][0]
__________________________________________________________________________________________________
dropout_28 (Dropout) (None, 128, 128, 32) 0 concatenate_14[0][0]
__________________________________________________________________________________________________
conv2d_67 (Conv2D) (None, 128, 128, 16) 4624 dropout_28[0][0]
__________________________________________________________________________________________________
batch_normalization_65 (BatchNo (None, 128, 128, 16) 64 conv2d_67[0][0]
__________________________________________________________________________________________________
leaky_re_lu_65 (LeakyReLU) (None, 128, 128, 16) 0 batch_normalization_65[0][0]
__________________________________________________________________________________________________
conv2d_68 (Conv2D) (None, 128, 128, 16) 2320 leaky_re_lu_65[0][0]
__________________________________________________________________________________________________
batch_normalization_66 (BatchNo (None, 128, 128, 16) 64 conv2d_68[0][0]
__________________________________________________________________________________________________
leaky_re_lu_66 (LeakyReLU) (None, 128, 128, 16) 0 batch_normalization_66[0][0]
__________________________________________________________________________________________________
conv2d_69 (Conv2D) (None, 128, 128, 255 4335 leaky_re_lu_66[0][0]
__________________________________________________________________________________________________
batch_normalization_67 (BatchNo (None, 128, 128, 255 1020 conv2d_69[0][0]
__________________________________________________________________________________________________
leaky_re_lu_67 (LeakyReLU) (None, 128, 128, 255 0 batch_normalization_67[0][0]
__________________________________________________________________________________________________
conv2d_70 (Conv2D) (None, 128, 128, 255 65280 leaky_re_lu_67[0][0]
__________________________________________________________________________________________________
batch_normalization_68 (BatchNo (None, 128, 128, 255 1020 conv2d_70[0][0]
__________________________________________________________________________________________________
leaky_re_lu_68 (LeakyReLU) (None, 128, 128, 255 0 batch_normalization_68[0][0]
__________________________________________________________________________________________________
conv2d_71 (Conv2D) (None, 128, 128, 255 65280 leaky_re_lu_68[0][0]
==================================================================================================
Total params: 2,302,087
Trainable params: 2,298,123
Non-trainable params: 3,964
Then I created callbacks on validation loss:
callbacks = [
EarlyStopping(patience=10, verbose=1),
ReduceLROnPlateau(factor=0.1, patience=2, min_lr=0.0000001, verbose=1),
ModelCheckpoint('model.h5', verbose=1, save_best_only=True, save_weights_only=True)
]
results = model.fit_generator(get_data(train_ids, batch_size=2), steps_per_epoch=675 , epochs=100, verbose=1 , callbacks=callbacks, validation_data = get_data(valid_ids,batch_size=1) , validation_steps = 243)
The results came like this:
Epoch 1/100
675/675 [==============================] - 1087s 2s/step - loss: 1.8353 - acc: 0.4686 - val_loss: 3.2239 - val_acc: 0.3724
Epoch 00001: val_loss improved from inf to 3.22391, saving model to model.h5
Epoch 2/100
675/675 [==============================] - 1049s 2s/step - loss: 1.8136 - acc: 0.4762 - val_loss: 1.9655 - val_acc: 0.4165
Epoch 00002: val_loss improved from 3.22391 to 1.96552, saving model to model.h5
Epoch 3/100
675/675 [==============================] - 1041s 2s/step - loss: 1.8003 - acc: 0.4810 - val_loss: 6.6811 - val_acc: 0.2085
Epoch 00003: val_loss did not improve from 1.96552
Epoch 4/100
675/675 [==============================] - 1053s 2s/step - loss: 1.7834 - acc: 0.4863 - val_loss: 10.5597 - val_acc: 0.1563
Epoch 00004: ReduceLROnPlateau reducing learning rate to 0.0009999999776482583.
Epoch 00004: val_loss did not improve from 1.96552
Epoch 5/100
675/675 [==============================] - 1057s 2s/step - loss: 1.7254 - acc: 0.5030 - val_loss: 2.1023 - val_acc: 0.4267
Epoch 00005: val_loss did not improve from 1.96552
Epoch 6/100
675/675 [==============================] - 1052s 2s/step - loss: 1.7088 - acc: 0.5083 - val_loss: 2.1336 - val_acc: 0.4183
Epoch 00006: ReduceLROnPlateau reducing learning rate to 9.999999310821295e-05.
Epoch 00006: val_loss did not improve from 1.96552
Epoch 7/100
675/675 [==============================] - 1052s 2s/step - loss: 1.6963 - acc: 0.5126 - val_loss: 1.6861 - val_acc: 0.5166
Epoch 00007: val_loss improved from 1.96552 to 1.68613, saving model to model.h5
Epoch 8/100
675/675 [==============================] - 1040s 2s/step - loss: 1.6937 - acc: 0.5137 - val_loss: 1.6918 - val_acc: 0.5150
Epoch 00008: val_loss did not improve from 1.68613
Epoch 9/100
675/675 [==============================] - 1019s 2s/step - loss: 1.6928 - acc: 0.5137 - val_loss: 1.6943 - val_acc: 0.5139
Epoch 00009: ReduceLROnPlateau reducing learning rate to 9.999999019782991e-06.
Epoch 00009: val_loss did not improve from 1.68613
Epoch 10/100
454/675 [===================>..........] - ETA: 4:39 - loss: 1.6907 - acc: 0.5154
As you can see the accuracy is not at all improving after later epochs. Loss is decreasing very very slowly. What's wrong with my model? How to get rid of this problem?
Help is appreciated. Thanks in advance.
neural-network deep-learning keras
New contributor
Sank_BE is a new contributor to this site. Take care in asking for clarification, commenting, and answering.
Check out our Code of Conduct.
$endgroup$
add a comment |
$begingroup$
So the problem is to perform a multiclass segmentation (255 classes of crops), and I am using a U-Net model for that. The input images are grayscale and the images of dimensions (128,128,1) are grouped into 7 groups (that is 7 channels). The output images are segmented images (128,128,1).
Approach: So I created a dataset for segmentation. Since the input images are to be grouped in 7, I created an input tensor (128,128,7) and the output tensor as (128,128,255) since there are 255 classes and we need this segmentation.
Number of Input:11151 (1593*7) images
Outputs: 1593 images
First I mounted my drive on google colab:
from google.colab import drive
drive.mount('/content/drive')
Go to this URL in a browser: https://accounts.google.com/o/oauth2/auth?client_id=947318989803-6bn6qk8qdgf4n4g3pfee6491hc0brc4i.apps.googleusercontent.com&redirect_uri=urn%3Aietf%3Awg%3Aoauth%3A2.0%3Aoob&scope=email%20https%3A%2F%2Fwww.googleapis.com%2Fauth%2Fdocs.test%20https%3A%2F%2Fwww.googleapis.com%2Fauth%2Fdrive%20https%3A%2F%2Fwww.googleapis.com%2Fauth%2Fdrive.photos.readonly%20https%3A%2F%2Fwww.googleapis.com%2Fauth%2Fpeopleapi.readonly&response_type=code
Enter your authorization code:
··········
Mounted at /content/drive
Then I loaded the libraries:
import os
import random
import pandas as pd
import numpy as np
import matplotlib.pyplot as plt
plt.style.use("ggplot")
%matplotlib inline
from itertools import chain
from skimage.io import imread, imshow, concatenate_images
from skimage.transform import resize
from skimage.morphology import label
from sklearn.model_selection import train_test_split
import tensorflow as tf
from keras.models import Model, load_model
from keras.layers import Input, BatchNormalization, Activation, Dense, Dropout
from keras.layers.core import Lambda, RepeatVector, Reshape
from keras.layers.convolutional import Conv2D, Conv2DTranspose
from keras.layers.pooling import MaxPooling2D, GlobalMaxPool2D
from keras.layers.merge import concatenate, add
from keras.callbacks import EarlyStopping, ModelCheckpoint, ReduceLROnPlateau
from keras.optimizers import Adam,SGD
from keras.preprocessing.image import ImageDataGenerator, array_to_img, img_to_array, load_img
from keras.layers import LeakyReLU
Then I divieded the dataset into training and validation:
im_width = 128
im_height = 128
border = 5
path_train_input = '/content/drive/path....'
path_train_output = '/content/drive/path..'
ids = next(os.walk(path_train_output))[2]
print(len(ids))
from random import shuffle
shuffle(ids)
train_ids = ids[0:1350]
valid_ids = ids[1350:]
print(len(train_ids))
I created this function to load the data in batches to avoid memory crashes in colab:
def get_data(ids,batch_size):
while True:
ids_batches = [ids[i:min(i+batch_size,len(ids))] for i in range(0, len(ids), batch_size)]
#ids_out_batches = [ids_out[j:min(j+batch_size,len(ids_out))] for j in range(0, len(ids_out), batch_size)]
# Load images
for b in range(len(ids_batches)):
k=-1
X = np.zeros((len(ids_batches[b]), im_height, im_width, 7), dtype=np.float32)
y = np.zeros((len(ids_batches[b]), im_height, im_width, 255), dtype=np.float32)
for c in range(len(ids_batches[b])):
k=k+1
#temp1=np.zeros((1,7),dtype=np.float32)
for r in range(1,8):
img = load_img(path_train_input + 'lc8' + ids_batches[b][c][3:-4] + '_' + str(r) + '.tif', color_mode="grayscale")
x_img = img_to_array(img)
x_img = resize(x_img, (128, 128), mode='constant', preserve_range=True)
for p in range(128):
for q in range(128):
X[k][p][q][r-1]=x_img[p][q]/255
#k=k+1
# Save images
#X[k, ..., 0] = temp1 / 255
# Load masks
mask = img_to_array(load_img(path_train_output+ids_batches[b][c], color_mode="grayscale"))
mask = resize(mask, (128, 128), mode='constant', preserve_range=True)
for p in range(128):
for q in range(128):
num=int(mask[p][q])
temp=np.zeros((255), dtype=np.float32)
temp[num]=1
y[k][p][q]=temp
yield X,y
Then I started creating my model:
def conv2d_block(input_tensor, n_filters, kernel_size=3, batchnorm=True):
# first layer
x = Conv2D(filters=n_filters, kernel_size=(kernel_size, kernel_size), kernel_initializer="he_normal",
padding="same")(input_tensor)
if batchnorm:
x = BatchNormalization()(x)
x = LeakyReLU(alpha=0.3)(x)
# second layer
x = Conv2D(filters=n_filters, kernel_size=(kernel_size, kernel_size), kernel_initializer="he_normal",
padding="same")(x)
if batchnorm:
x = BatchNormalization()(x)
x = LeakyReLU(alpha=0.3)(x)
return x
Then the U-Net:
def get_unet(input_img, n_filters=16, dropout=0.15, batchnorm=True):
# contracting path
c1 = conv2d_block(input_img, n_filters=n_filters*1, kernel_size=3, batchnorm=batchnorm)
p1 = MaxPooling2D((2, 2)) (c1)
p1 = Dropout(rate=dropout*0.5)(p1)
c2 = conv2d_block(p1, n_filters=n_filters*2, kernel_size=3, batchnorm=batchnorm)
p2 = MaxPooling2D((2, 2)) (c2)
p2 = Dropout(rate=dropout)(p2)
c3 = conv2d_block(p2, n_filters=n_filters*4, kernel_size=3, batchnorm=batchnorm)
p3 = MaxPooling2D((2, 2)) (c3)
p3 = Dropout(rate=dropout)(p3)
c4 = conv2d_block(p3, n_filters=n_filters*8, kernel_size=3, batchnorm=batchnorm)
p4 = MaxPooling2D(pool_size=(2, 2)) (c4)
p4 = Dropout(rate=dropout)(p4)
c5 = conv2d_block(p4, n_filters=n_filters*16, kernel_size=3, batchnorm=batchnorm)
# expansive path
u6 = Conv2DTranspose(n_filters*8, (3, 3), strides=(2, 2), padding='same') (c5)
u6 = concatenate([u6, c4])
u6 = Dropout(rate=dropout)(u6)
c6 = conv2d_block(u6, n_filters=n_filters*8, kernel_size=3, batchnorm=batchnorm)
u7 = Conv2DTranspose(n_filters*4, (3, 3), strides=(2, 2), padding='same') (c6)
u7 = concatenate([u7, c3])
u7 = Dropout(rate=dropout)(u7)
c7 = conv2d_block(u7, n_filters=n_filters*4, kernel_size=3, batchnorm=batchnorm)
u8 = Conv2DTranspose(n_filters*2, (3, 3), strides=(2, 2), padding='same') (c7)
u8 = concatenate([u8, c2])
u8 = Dropout(rate=dropout)(u8)
c8 = conv2d_block(u8, n_filters=n_filters*2, kernel_size=3, batchnorm=batchnorm)
u9 = Conv2DTranspose(n_filters*1, (3, 3), strides=(2, 2), padding='same') (c8)
u9 = concatenate([u9, c1], axis=3)
u9 = Dropout(rate=dropout)(u9)
c9 = conv2d_block(u9, n_filters=n_filters*1, kernel_size=3, batchnorm=batchnorm)
c10 = conv2d_block(c9, n_filters=255, kernel_size=1, batchnorm=batchnorm)
#conv6 = core.Reshape((1,128,128))(c9)
#conv6 = core.Permute((2,1))(conv6)
outputs = Conv2D(255, (1, 1), activation='softmax') (c10)
model = Model(inputs=[input_img], outputs=[outputs])
return model
input_img = Input((im_height, im_width, 7), name='img')
model = get_unet(input_img, n_filters=16, dropout=0.15, batchnorm=True)
#model = get_unet()
model.compile(optimizer=Adam(lr=0.01), loss="categorical_crossentropy", metrics=["accuracy"])
model.summary()
As you can see in the model summary input is (128,128,7) and output (128,128,255):
__________________________________________________________________________________________________
Layer (type) Output Shape Param # Connected to
==================================================================================================
img (InputLayer) (None, 128, 128, 7) 0
__________________________________________________________________________________________________
conv2d_51 (Conv2D) (None, 128, 128, 16) 1024 img[0][0]
__________________________________________________________________________________________________
batch_normalization_49 (BatchNo (None, 128, 128, 16) 64 conv2d_51[0][0]
__________________________________________________________________________________________________
leaky_re_lu_49 (LeakyReLU) (None, 128, 128, 16) 0 batch_normalization_49[0][0]
__________________________________________________________________________________________________
conv2d_52 (Conv2D) (None, 128, 128, 16) 2320 leaky_re_lu_49[0][0]
__________________________________________________________________________________________________
batch_normalization_50 (BatchNo (None, 128, 128, 16) 64 conv2d_52[0][0]
__________________________________________________________________________________________________
leaky_re_lu_50 (LeakyReLU) (None, 128, 128, 16) 0 batch_normalization_50[0][0]
__________________________________________________________________________________________________
max_pooling2d_11 (MaxPooling2D) (None, 64, 64, 16) 0 leaky_re_lu_50[0][0]
__________________________________________________________________________________________________
dropout_21 (Dropout) (None, 64, 64, 16) 0 max_pooling2d_11[0][0]
__________________________________________________________________________________________________
conv2d_53 (Conv2D) (None, 64, 64, 32) 4640 dropout_21[0][0]
__________________________________________________________________________________________________
batch_normalization_51 (BatchNo (None, 64, 64, 32) 128 conv2d_53[0][0]
__________________________________________________________________________________________________
leaky_re_lu_51 (LeakyReLU) (None, 64, 64, 32) 0 batch_normalization_51[0][0]
__________________________________________________________________________________________________
conv2d_54 (Conv2D) (None, 64, 64, 32) 9248 leaky_re_lu_51[0][0]
__________________________________________________________________________________________________
batch_normalization_52 (BatchNo (None, 64, 64, 32) 128 conv2d_54[0][0]
__________________________________________________________________________________________________
leaky_re_lu_52 (LeakyReLU) (None, 64, 64, 32) 0 batch_normalization_52[0][0]
__________________________________________________________________________________________________
max_pooling2d_12 (MaxPooling2D) (None, 32, 32, 32) 0 leaky_re_lu_52[0][0]
__________________________________________________________________________________________________
dropout_22 (Dropout) (None, 32, 32, 32) 0 max_pooling2d_12[0][0]
__________________________________________________________________________________________________
conv2d_55 (Conv2D) (None, 32, 32, 64) 18496 dropout_22[0][0]
__________________________________________________________________________________________________
batch_normalization_53 (BatchNo (None, 32, 32, 64) 256 conv2d_55[0][0]
__________________________________________________________________________________________________
leaky_re_lu_53 (LeakyReLU) (None, 32, 32, 64) 0 batch_normalization_53[0][0]
__________________________________________________________________________________________________
conv2d_56 (Conv2D) (None, 32, 32, 64) 36928 leaky_re_lu_53[0][0]
__________________________________________________________________________________________________
batch_normalization_54 (BatchNo (None, 32, 32, 64) 256 conv2d_56[0][0]
__________________________________________________________________________________________________
leaky_re_lu_54 (LeakyReLU) (None, 32, 32, 64) 0 batch_normalization_54[0][0]
__________________________________________________________________________________________________
max_pooling2d_13 (MaxPooling2D) (None, 16, 16, 64) 0 leaky_re_lu_54[0][0]
__________________________________________________________________________________________________
dropout_23 (Dropout) (None, 16, 16, 64) 0 max_pooling2d_13[0][0]
__________________________________________________________________________________________________
conv2d_57 (Conv2D) (None, 16, 16, 128) 73856 dropout_23[0][0]
__________________________________________________________________________________________________
batch_normalization_55 (BatchNo (None, 16, 16, 128) 512 conv2d_57[0][0]
__________________________________________________________________________________________________
leaky_re_lu_55 (LeakyReLU) (None, 16, 16, 128) 0 batch_normalization_55[0][0]
__________________________________________________________________________________________________
conv2d_58 (Conv2D) (None, 16, 16, 128) 147584 leaky_re_lu_55[0][0]
__________________________________________________________________________________________________
batch_normalization_56 (BatchNo (None, 16, 16, 128) 512 conv2d_58[0][0]
__________________________________________________________________________________________________
leaky_re_lu_56 (LeakyReLU) (None, 16, 16, 128) 0 batch_normalization_56[0][0]
__________________________________________________________________________________________________
max_pooling2d_14 (MaxPooling2D) (None, 8, 8, 128) 0 leaky_re_lu_56[0][0]
__________________________________________________________________________________________________
dropout_24 (Dropout) (None, 8, 8, 128) 0 max_pooling2d_14[0][0]
__________________________________________________________________________________________________
conv2d_59 (Conv2D) (None, 8, 8, 256) 295168 dropout_24[0][0]
__________________________________________________________________________________________________
batch_normalization_57 (BatchNo (None, 8, 8, 256) 1024 conv2d_59[0][0]
__________________________________________________________________________________________________
leaky_re_lu_57 (LeakyReLU) (None, 8, 8, 256) 0 batch_normalization_57[0][0]
__________________________________________________________________________________________________
conv2d_60 (Conv2D) (None, 8, 8, 256) 590080 leaky_re_lu_57[0][0]
__________________________________________________________________________________________________
batch_normalization_58 (BatchNo (None, 8, 8, 256) 1024 conv2d_60[0][0]
__________________________________________________________________________________________________
leaky_re_lu_58 (LeakyReLU) (None, 8, 8, 256) 0 batch_normalization_58[0][0]
__________________________________________________________________________________________________
conv2d_transpose_11 (Conv2DTran (None, 16, 16, 128) 295040 leaky_re_lu_58[0][0]
__________________________________________________________________________________________________
concatenate_11 (Concatenate) (None, 16, 16, 256) 0 conv2d_transpose_11[0][0]
leaky_re_lu_56[0][0]
__________________________________________________________________________________________________
dropout_25 (Dropout) (None, 16, 16, 256) 0 concatenate_11[0][0]
__________________________________________________________________________________________________
conv2d_61 (Conv2D) (None, 16, 16, 128) 295040 dropout_25[0][0]
__________________________________________________________________________________________________
batch_normalization_59 (BatchNo (None, 16, 16, 128) 512 conv2d_61[0][0]
__________________________________________________________________________________________________
leaky_re_lu_59 (LeakyReLU) (None, 16, 16, 128) 0 batch_normalization_59[0][0]
__________________________________________________________________________________________________
conv2d_62 (Conv2D) (None, 16, 16, 128) 147584 leaky_re_lu_59[0][0]
__________________________________________________________________________________________________
batch_normalization_60 (BatchNo (None, 16, 16, 128) 512 conv2d_62[0][0]
__________________________________________________________________________________________________
leaky_re_lu_60 (LeakyReLU) (None, 16, 16, 128) 0 batch_normalization_60[0][0]
__________________________________________________________________________________________________
conv2d_transpose_12 (Conv2DTran (None, 32, 32, 64) 73792 leaky_re_lu_60[0][0]
__________________________________________________________________________________________________
concatenate_12 (Concatenate) (None, 32, 32, 128) 0 conv2d_transpose_12[0][0]
leaky_re_lu_54[0][0]
__________________________________________________________________________________________________
dropout_26 (Dropout) (None, 32, 32, 128) 0 concatenate_12[0][0]
__________________________________________________________________________________________________
conv2d_63 (Conv2D) (None, 32, 32, 64) 73792 dropout_26[0][0]
__________________________________________________________________________________________________
batch_normalization_61 (BatchNo (None, 32, 32, 64) 256 conv2d_63[0][0]
__________________________________________________________________________________________________
leaky_re_lu_61 (LeakyReLU) (None, 32, 32, 64) 0 batch_normalization_61[0][0]
__________________________________________________________________________________________________
conv2d_64 (Conv2D) (None, 32, 32, 64) 36928 leaky_re_lu_61[0][0]
__________________________________________________________________________________________________
batch_normalization_62 (BatchNo (None, 32, 32, 64) 256 conv2d_64[0][0]
__________________________________________________________________________________________________
leaky_re_lu_62 (LeakyReLU) (None, 32, 32, 64) 0 batch_normalization_62[0][0]
__________________________________________________________________________________________________
conv2d_transpose_13 (Conv2DTran (None, 64, 64, 32) 18464 leaky_re_lu_62[0][0]
__________________________________________________________________________________________________
concatenate_13 (Concatenate) (None, 64, 64, 64) 0 conv2d_transpose_13[0][0]
leaky_re_lu_52[0][0]
__________________________________________________________________________________________________
dropout_27 (Dropout) (None, 64, 64, 64) 0 concatenate_13[0][0]
__________________________________________________________________________________________________
conv2d_65 (Conv2D) (None, 64, 64, 32) 18464 dropout_27[0][0]
__________________________________________________________________________________________________
batch_normalization_63 (BatchNo (None, 64, 64, 32) 128 conv2d_65[0][0]
__________________________________________________________________________________________________
leaky_re_lu_63 (LeakyReLU) (None, 64, 64, 32) 0 batch_normalization_63[0][0]
__________________________________________________________________________________________________
conv2d_66 (Conv2D) (None, 64, 64, 32) 9248 leaky_re_lu_63[0][0]
__________________________________________________________________________________________________
batch_normalization_64 (BatchNo (None, 64, 64, 32) 128 conv2d_66[0][0]
__________________________________________________________________________________________________
leaky_re_lu_64 (LeakyReLU) (None, 64, 64, 32) 0 batch_normalization_64[0][0]
__________________________________________________________________________________________________
conv2d_transpose_14 (Conv2DTran (None, 128, 128, 16) 4624 leaky_re_lu_64[0][0]
__________________________________________________________________________________________________
concatenate_14 (Concatenate) (None, 128, 128, 32) 0 conv2d_transpose_14[0][0]
leaky_re_lu_50[0][0]
__________________________________________________________________________________________________
dropout_28 (Dropout) (None, 128, 128, 32) 0 concatenate_14[0][0]
__________________________________________________________________________________________________
conv2d_67 (Conv2D) (None, 128, 128, 16) 4624 dropout_28[0][0]
__________________________________________________________________________________________________
batch_normalization_65 (BatchNo (None, 128, 128, 16) 64 conv2d_67[0][0]
__________________________________________________________________________________________________
leaky_re_lu_65 (LeakyReLU) (None, 128, 128, 16) 0 batch_normalization_65[0][0]
__________________________________________________________________________________________________
conv2d_68 (Conv2D) (None, 128, 128, 16) 2320 leaky_re_lu_65[0][0]
__________________________________________________________________________________________________
batch_normalization_66 (BatchNo (None, 128, 128, 16) 64 conv2d_68[0][0]
__________________________________________________________________________________________________
leaky_re_lu_66 (LeakyReLU) (None, 128, 128, 16) 0 batch_normalization_66[0][0]
__________________________________________________________________________________________________
conv2d_69 (Conv2D) (None, 128, 128, 255 4335 leaky_re_lu_66[0][0]
__________________________________________________________________________________________________
batch_normalization_67 (BatchNo (None, 128, 128, 255 1020 conv2d_69[0][0]
__________________________________________________________________________________________________
leaky_re_lu_67 (LeakyReLU) (None, 128, 128, 255 0 batch_normalization_67[0][0]
__________________________________________________________________________________________________
conv2d_70 (Conv2D) (None, 128, 128, 255 65280 leaky_re_lu_67[0][0]
__________________________________________________________________________________________________
batch_normalization_68 (BatchNo (None, 128, 128, 255 1020 conv2d_70[0][0]
__________________________________________________________________________________________________
leaky_re_lu_68 (LeakyReLU) (None, 128, 128, 255 0 batch_normalization_68[0][0]
__________________________________________________________________________________________________
conv2d_71 (Conv2D) (None, 128, 128, 255 65280 leaky_re_lu_68[0][0]
==================================================================================================
Total params: 2,302,087
Trainable params: 2,298,123
Non-trainable params: 3,964
Then I created callbacks on validation loss:
callbacks = [
EarlyStopping(patience=10, verbose=1),
ReduceLROnPlateau(factor=0.1, patience=2, min_lr=0.0000001, verbose=1),
ModelCheckpoint('model.h5', verbose=1, save_best_only=True, save_weights_only=True)
]
results = model.fit_generator(get_data(train_ids, batch_size=2), steps_per_epoch=675 , epochs=100, verbose=1 , callbacks=callbacks, validation_data = get_data(valid_ids,batch_size=1) , validation_steps = 243)
The results came like this:
Epoch 1/100
675/675 [==============================] - 1087s 2s/step - loss: 1.8353 - acc: 0.4686 - val_loss: 3.2239 - val_acc: 0.3724
Epoch 00001: val_loss improved from inf to 3.22391, saving model to model.h5
Epoch 2/100
675/675 [==============================] - 1049s 2s/step - loss: 1.8136 - acc: 0.4762 - val_loss: 1.9655 - val_acc: 0.4165
Epoch 00002: val_loss improved from 3.22391 to 1.96552, saving model to model.h5
Epoch 3/100
675/675 [==============================] - 1041s 2s/step - loss: 1.8003 - acc: 0.4810 - val_loss: 6.6811 - val_acc: 0.2085
Epoch 00003: val_loss did not improve from 1.96552
Epoch 4/100
675/675 [==============================] - 1053s 2s/step - loss: 1.7834 - acc: 0.4863 - val_loss: 10.5597 - val_acc: 0.1563
Epoch 00004: ReduceLROnPlateau reducing learning rate to 0.0009999999776482583.
Epoch 00004: val_loss did not improve from 1.96552
Epoch 5/100
675/675 [==============================] - 1057s 2s/step - loss: 1.7254 - acc: 0.5030 - val_loss: 2.1023 - val_acc: 0.4267
Epoch 00005: val_loss did not improve from 1.96552
Epoch 6/100
675/675 [==============================] - 1052s 2s/step - loss: 1.7088 - acc: 0.5083 - val_loss: 2.1336 - val_acc: 0.4183
Epoch 00006: ReduceLROnPlateau reducing learning rate to 9.999999310821295e-05.
Epoch 00006: val_loss did not improve from 1.96552
Epoch 7/100
675/675 [==============================] - 1052s 2s/step - loss: 1.6963 - acc: 0.5126 - val_loss: 1.6861 - val_acc: 0.5166
Epoch 00007: val_loss improved from 1.96552 to 1.68613, saving model to model.h5
Epoch 8/100
675/675 [==============================] - 1040s 2s/step - loss: 1.6937 - acc: 0.5137 - val_loss: 1.6918 - val_acc: 0.5150
Epoch 00008: val_loss did not improve from 1.68613
Epoch 9/100
675/675 [==============================] - 1019s 2s/step - loss: 1.6928 - acc: 0.5137 - val_loss: 1.6943 - val_acc: 0.5139
Epoch 00009: ReduceLROnPlateau reducing learning rate to 9.999999019782991e-06.
Epoch 00009: val_loss did not improve from 1.68613
Epoch 10/100
454/675 [===================>..........] - ETA: 4:39 - loss: 1.6907 - acc: 0.5154
As you can see the accuracy is not at all improving after later epochs. Loss is decreasing very very slowly. What's wrong with my model? How to get rid of this problem?
Help is appreciated. Thanks in advance.
neural-network deep-learning keras
New contributor
Sank_BE is a new contributor to this site. Take care in asking for clarification, commenting, and answering.
Check out our Code of Conduct.
$endgroup$
$begingroup$
Anyone please help me? How can I set bounty on this question? I see no such option below.
$endgroup$
– Sank_BE
12 hours ago
add a comment |
$begingroup$
So the problem is to perform a multiclass segmentation (255 classes of crops), and I am using a U-Net model for that. The input images are grayscale and the images of dimensions (128,128,1) are grouped into 7 groups (that is 7 channels). The output images are segmented images (128,128,1).
Approach: So I created a dataset for segmentation. Since the input images are to be grouped in 7, I created an input tensor (128,128,7) and the output tensor as (128,128,255) since there are 255 classes and we need this segmentation.
Number of Input:11151 (1593*7) images
Outputs: 1593 images
First I mounted my drive on google colab:
from google.colab import drive
drive.mount('/content/drive')
Go to this URL in a browser: https://accounts.google.com/o/oauth2/auth?client_id=947318989803-6bn6qk8qdgf4n4g3pfee6491hc0brc4i.apps.googleusercontent.com&redirect_uri=urn%3Aietf%3Awg%3Aoauth%3A2.0%3Aoob&scope=email%20https%3A%2F%2Fwww.googleapis.com%2Fauth%2Fdocs.test%20https%3A%2F%2Fwww.googleapis.com%2Fauth%2Fdrive%20https%3A%2F%2Fwww.googleapis.com%2Fauth%2Fdrive.photos.readonly%20https%3A%2F%2Fwww.googleapis.com%2Fauth%2Fpeopleapi.readonly&response_type=code
Enter your authorization code:
··········
Mounted at /content/drive
Then I loaded the libraries:
import os
import random
import pandas as pd
import numpy as np
import matplotlib.pyplot as plt
plt.style.use("ggplot")
%matplotlib inline
from itertools import chain
from skimage.io import imread, imshow, concatenate_images
from skimage.transform import resize
from skimage.morphology import label
from sklearn.model_selection import train_test_split
import tensorflow as tf
from keras.models import Model, load_model
from keras.layers import Input, BatchNormalization, Activation, Dense, Dropout
from keras.layers.core import Lambda, RepeatVector, Reshape
from keras.layers.convolutional import Conv2D, Conv2DTranspose
from keras.layers.pooling import MaxPooling2D, GlobalMaxPool2D
from keras.layers.merge import concatenate, add
from keras.callbacks import EarlyStopping, ModelCheckpoint, ReduceLROnPlateau
from keras.optimizers import Adam,SGD
from keras.preprocessing.image import ImageDataGenerator, array_to_img, img_to_array, load_img
from keras.layers import LeakyReLU
Then I divieded the dataset into training and validation:
im_width = 128
im_height = 128
border = 5
path_train_input = '/content/drive/path....'
path_train_output = '/content/drive/path..'
ids = next(os.walk(path_train_output))[2]
print(len(ids))
from random import shuffle
shuffle(ids)
train_ids = ids[0:1350]
valid_ids = ids[1350:]
print(len(train_ids))
I created this function to load the data in batches to avoid memory crashes in colab:
def get_data(ids,batch_size):
while True:
ids_batches = [ids[i:min(i+batch_size,len(ids))] for i in range(0, len(ids), batch_size)]
#ids_out_batches = [ids_out[j:min(j+batch_size,len(ids_out))] for j in range(0, len(ids_out), batch_size)]
# Load images
for b in range(len(ids_batches)):
k=-1
X = np.zeros((len(ids_batches[b]), im_height, im_width, 7), dtype=np.float32)
y = np.zeros((len(ids_batches[b]), im_height, im_width, 255), dtype=np.float32)
for c in range(len(ids_batches[b])):
k=k+1
#temp1=np.zeros((1,7),dtype=np.float32)
for r in range(1,8):
img = load_img(path_train_input + 'lc8' + ids_batches[b][c][3:-4] + '_' + str(r) + '.tif', color_mode="grayscale")
x_img = img_to_array(img)
x_img = resize(x_img, (128, 128), mode='constant', preserve_range=True)
for p in range(128):
for q in range(128):
X[k][p][q][r-1]=x_img[p][q]/255
#k=k+1
# Save images
#X[k, ..., 0] = temp1 / 255
# Load masks
mask = img_to_array(load_img(path_train_output+ids_batches[b][c], color_mode="grayscale"))
mask = resize(mask, (128, 128), mode='constant', preserve_range=True)
for p in range(128):
for q in range(128):
num=int(mask[p][q])
temp=np.zeros((255), dtype=np.float32)
temp[num]=1
y[k][p][q]=temp
yield X,y
Then I started creating my model:
def conv2d_block(input_tensor, n_filters, kernel_size=3, batchnorm=True):
# first layer
x = Conv2D(filters=n_filters, kernel_size=(kernel_size, kernel_size), kernel_initializer="he_normal",
padding="same")(input_tensor)
if batchnorm:
x = BatchNormalization()(x)
x = LeakyReLU(alpha=0.3)(x)
# second layer
x = Conv2D(filters=n_filters, kernel_size=(kernel_size, kernel_size), kernel_initializer="he_normal",
padding="same")(x)
if batchnorm:
x = BatchNormalization()(x)
x = LeakyReLU(alpha=0.3)(x)
return x
Then the U-Net:
def get_unet(input_img, n_filters=16, dropout=0.15, batchnorm=True):
# contracting path
c1 = conv2d_block(input_img, n_filters=n_filters*1, kernel_size=3, batchnorm=batchnorm)
p1 = MaxPooling2D((2, 2)) (c1)
p1 = Dropout(rate=dropout*0.5)(p1)
c2 = conv2d_block(p1, n_filters=n_filters*2, kernel_size=3, batchnorm=batchnorm)
p2 = MaxPooling2D((2, 2)) (c2)
p2 = Dropout(rate=dropout)(p2)
c3 = conv2d_block(p2, n_filters=n_filters*4, kernel_size=3, batchnorm=batchnorm)
p3 = MaxPooling2D((2, 2)) (c3)
p3 = Dropout(rate=dropout)(p3)
c4 = conv2d_block(p3, n_filters=n_filters*8, kernel_size=3, batchnorm=batchnorm)
p4 = MaxPooling2D(pool_size=(2, 2)) (c4)
p4 = Dropout(rate=dropout)(p4)
c5 = conv2d_block(p4, n_filters=n_filters*16, kernel_size=3, batchnorm=batchnorm)
# expansive path
u6 = Conv2DTranspose(n_filters*8, (3, 3), strides=(2, 2), padding='same') (c5)
u6 = concatenate([u6, c4])
u6 = Dropout(rate=dropout)(u6)
c6 = conv2d_block(u6, n_filters=n_filters*8, kernel_size=3, batchnorm=batchnorm)
u7 = Conv2DTranspose(n_filters*4, (3, 3), strides=(2, 2), padding='same') (c6)
u7 = concatenate([u7, c3])
u7 = Dropout(rate=dropout)(u7)
c7 = conv2d_block(u7, n_filters=n_filters*4, kernel_size=3, batchnorm=batchnorm)
u8 = Conv2DTranspose(n_filters*2, (3, 3), strides=(2, 2), padding='same') (c7)
u8 = concatenate([u8, c2])
u8 = Dropout(rate=dropout)(u8)
c8 = conv2d_block(u8, n_filters=n_filters*2, kernel_size=3, batchnorm=batchnorm)
u9 = Conv2DTranspose(n_filters*1, (3, 3), strides=(2, 2), padding='same') (c8)
u9 = concatenate([u9, c1], axis=3)
u9 = Dropout(rate=dropout)(u9)
c9 = conv2d_block(u9, n_filters=n_filters*1, kernel_size=3, batchnorm=batchnorm)
c10 = conv2d_block(c9, n_filters=255, kernel_size=1, batchnorm=batchnorm)
#conv6 = core.Reshape((1,128,128))(c9)
#conv6 = core.Permute((2,1))(conv6)
outputs = Conv2D(255, (1, 1), activation='softmax') (c10)
model = Model(inputs=[input_img], outputs=[outputs])
return model
input_img = Input((im_height, im_width, 7), name='img')
model = get_unet(input_img, n_filters=16, dropout=0.15, batchnorm=True)
#model = get_unet()
model.compile(optimizer=Adam(lr=0.01), loss="categorical_crossentropy", metrics=["accuracy"])
model.summary()
As you can see in the model summary input is (128,128,7) and output (128,128,255):
__________________________________________________________________________________________________
Layer (type) Output Shape Param # Connected to
==================================================================================================
img (InputLayer) (None, 128, 128, 7) 0
__________________________________________________________________________________________________
conv2d_51 (Conv2D) (None, 128, 128, 16) 1024 img[0][0]
__________________________________________________________________________________________________
batch_normalization_49 (BatchNo (None, 128, 128, 16) 64 conv2d_51[0][0]
__________________________________________________________________________________________________
leaky_re_lu_49 (LeakyReLU) (None, 128, 128, 16) 0 batch_normalization_49[0][0]
__________________________________________________________________________________________________
conv2d_52 (Conv2D) (None, 128, 128, 16) 2320 leaky_re_lu_49[0][0]
__________________________________________________________________________________________________
batch_normalization_50 (BatchNo (None, 128, 128, 16) 64 conv2d_52[0][0]
__________________________________________________________________________________________________
leaky_re_lu_50 (LeakyReLU) (None, 128, 128, 16) 0 batch_normalization_50[0][0]
__________________________________________________________________________________________________
max_pooling2d_11 (MaxPooling2D) (None, 64, 64, 16) 0 leaky_re_lu_50[0][0]
__________________________________________________________________________________________________
dropout_21 (Dropout) (None, 64, 64, 16) 0 max_pooling2d_11[0][0]
__________________________________________________________________________________________________
conv2d_53 (Conv2D) (None, 64, 64, 32) 4640 dropout_21[0][0]
__________________________________________________________________________________________________
batch_normalization_51 (BatchNo (None, 64, 64, 32) 128 conv2d_53[0][0]
__________________________________________________________________________________________________
leaky_re_lu_51 (LeakyReLU) (None, 64, 64, 32) 0 batch_normalization_51[0][0]
__________________________________________________________________________________________________
conv2d_54 (Conv2D) (None, 64, 64, 32) 9248 leaky_re_lu_51[0][0]
__________________________________________________________________________________________________
batch_normalization_52 (BatchNo (None, 64, 64, 32) 128 conv2d_54[0][0]
__________________________________________________________________________________________________
leaky_re_lu_52 (LeakyReLU) (None, 64, 64, 32) 0 batch_normalization_52[0][0]
__________________________________________________________________________________________________
max_pooling2d_12 (MaxPooling2D) (None, 32, 32, 32) 0 leaky_re_lu_52[0][0]
__________________________________________________________________________________________________
dropout_22 (Dropout) (None, 32, 32, 32) 0 max_pooling2d_12[0][0]
__________________________________________________________________________________________________
conv2d_55 (Conv2D) (None, 32, 32, 64) 18496 dropout_22[0][0]
__________________________________________________________________________________________________
batch_normalization_53 (BatchNo (None, 32, 32, 64) 256 conv2d_55[0][0]
__________________________________________________________________________________________________
leaky_re_lu_53 (LeakyReLU) (None, 32, 32, 64) 0 batch_normalization_53[0][0]
__________________________________________________________________________________________________
conv2d_56 (Conv2D) (None, 32, 32, 64) 36928 leaky_re_lu_53[0][0]
__________________________________________________________________________________________________
batch_normalization_54 (BatchNo (None, 32, 32, 64) 256 conv2d_56[0][0]
__________________________________________________________________________________________________
leaky_re_lu_54 (LeakyReLU) (None, 32, 32, 64) 0 batch_normalization_54[0][0]
__________________________________________________________________________________________________
max_pooling2d_13 (MaxPooling2D) (None, 16, 16, 64) 0 leaky_re_lu_54[0][0]
__________________________________________________________________________________________________
dropout_23 (Dropout) (None, 16, 16, 64) 0 max_pooling2d_13[0][0]
__________________________________________________________________________________________________
conv2d_57 (Conv2D) (None, 16, 16, 128) 73856 dropout_23[0][0]
__________________________________________________________________________________________________
batch_normalization_55 (BatchNo (None, 16, 16, 128) 512 conv2d_57[0][0]
__________________________________________________________________________________________________
leaky_re_lu_55 (LeakyReLU) (None, 16, 16, 128) 0 batch_normalization_55[0][0]
__________________________________________________________________________________________________
conv2d_58 (Conv2D) (None, 16, 16, 128) 147584 leaky_re_lu_55[0][0]
__________________________________________________________________________________________________
batch_normalization_56 (BatchNo (None, 16, 16, 128) 512 conv2d_58[0][0]
__________________________________________________________________________________________________
leaky_re_lu_56 (LeakyReLU) (None, 16, 16, 128) 0 batch_normalization_56[0][0]
__________________________________________________________________________________________________
max_pooling2d_14 (MaxPooling2D) (None, 8, 8, 128) 0 leaky_re_lu_56[0][0]
__________________________________________________________________________________________________
dropout_24 (Dropout) (None, 8, 8, 128) 0 max_pooling2d_14[0][0]
__________________________________________________________________________________________________
conv2d_59 (Conv2D) (None, 8, 8, 256) 295168 dropout_24[0][0]
__________________________________________________________________________________________________
batch_normalization_57 (BatchNo (None, 8, 8, 256) 1024 conv2d_59[0][0]
__________________________________________________________________________________________________
leaky_re_lu_57 (LeakyReLU) (None, 8, 8, 256) 0 batch_normalization_57[0][0]
__________________________________________________________________________________________________
conv2d_60 (Conv2D) (None, 8, 8, 256) 590080 leaky_re_lu_57[0][0]
__________________________________________________________________________________________________
batch_normalization_58 (BatchNo (None, 8, 8, 256) 1024 conv2d_60[0][0]
__________________________________________________________________________________________________
leaky_re_lu_58 (LeakyReLU) (None, 8, 8, 256) 0 batch_normalization_58[0][0]
__________________________________________________________________________________________________
conv2d_transpose_11 (Conv2DTran (None, 16, 16, 128) 295040 leaky_re_lu_58[0][0]
__________________________________________________________________________________________________
concatenate_11 (Concatenate) (None, 16, 16, 256) 0 conv2d_transpose_11[0][0]
leaky_re_lu_56[0][0]
__________________________________________________________________________________________________
dropout_25 (Dropout) (None, 16, 16, 256) 0 concatenate_11[0][0]
__________________________________________________________________________________________________
conv2d_61 (Conv2D) (None, 16, 16, 128) 295040 dropout_25[0][0]
__________________________________________________________________________________________________
batch_normalization_59 (BatchNo (None, 16, 16, 128) 512 conv2d_61[0][0]
__________________________________________________________________________________________________
leaky_re_lu_59 (LeakyReLU) (None, 16, 16, 128) 0 batch_normalization_59[0][0]
__________________________________________________________________________________________________
conv2d_62 (Conv2D) (None, 16, 16, 128) 147584 leaky_re_lu_59[0][0]
__________________________________________________________________________________________________
batch_normalization_60 (BatchNo (None, 16, 16, 128) 512 conv2d_62[0][0]
__________________________________________________________________________________________________
leaky_re_lu_60 (LeakyReLU) (None, 16, 16, 128) 0 batch_normalization_60[0][0]
__________________________________________________________________________________________________
conv2d_transpose_12 (Conv2DTran (None, 32, 32, 64) 73792 leaky_re_lu_60[0][0]
__________________________________________________________________________________________________
concatenate_12 (Concatenate) (None, 32, 32, 128) 0 conv2d_transpose_12[0][0]
leaky_re_lu_54[0][0]
__________________________________________________________________________________________________
dropout_26 (Dropout) (None, 32, 32, 128) 0 concatenate_12[0][0]
__________________________________________________________________________________________________
conv2d_63 (Conv2D) (None, 32, 32, 64) 73792 dropout_26[0][0]
__________________________________________________________________________________________________
batch_normalization_61 (BatchNo (None, 32, 32, 64) 256 conv2d_63[0][0]
__________________________________________________________________________________________________
leaky_re_lu_61 (LeakyReLU) (None, 32, 32, 64) 0 batch_normalization_61[0][0]
__________________________________________________________________________________________________
conv2d_64 (Conv2D) (None, 32, 32, 64) 36928 leaky_re_lu_61[0][0]
__________________________________________________________________________________________________
batch_normalization_62 (BatchNo (None, 32, 32, 64) 256 conv2d_64[0][0]
__________________________________________________________________________________________________
leaky_re_lu_62 (LeakyReLU) (None, 32, 32, 64) 0 batch_normalization_62[0][0]
__________________________________________________________________________________________________
conv2d_transpose_13 (Conv2DTran (None, 64, 64, 32) 18464 leaky_re_lu_62[0][0]
__________________________________________________________________________________________________
concatenate_13 (Concatenate) (None, 64, 64, 64) 0 conv2d_transpose_13[0][0]
leaky_re_lu_52[0][0]
__________________________________________________________________________________________________
dropout_27 (Dropout) (None, 64, 64, 64) 0 concatenate_13[0][0]
__________________________________________________________________________________________________
conv2d_65 (Conv2D) (None, 64, 64, 32) 18464 dropout_27[0][0]
__________________________________________________________________________________________________
batch_normalization_63 (BatchNo (None, 64, 64, 32) 128 conv2d_65[0][0]
__________________________________________________________________________________________________
leaky_re_lu_63 (LeakyReLU) (None, 64, 64, 32) 0 batch_normalization_63[0][0]
__________________________________________________________________________________________________
conv2d_66 (Conv2D) (None, 64, 64, 32) 9248 leaky_re_lu_63[0][0]
__________________________________________________________________________________________________
batch_normalization_64 (BatchNo (None, 64, 64, 32) 128 conv2d_66[0][0]
__________________________________________________________________________________________________
leaky_re_lu_64 (LeakyReLU) (None, 64, 64, 32) 0 batch_normalization_64[0][0]
__________________________________________________________________________________________________
conv2d_transpose_14 (Conv2DTran (None, 128, 128, 16) 4624 leaky_re_lu_64[0][0]
__________________________________________________________________________________________________
concatenate_14 (Concatenate) (None, 128, 128, 32) 0 conv2d_transpose_14[0][0]
leaky_re_lu_50[0][0]
__________________________________________________________________________________________________
dropout_28 (Dropout) (None, 128, 128, 32) 0 concatenate_14[0][0]
__________________________________________________________________________________________________
conv2d_67 (Conv2D) (None, 128, 128, 16) 4624 dropout_28[0][0]
__________________________________________________________________________________________________
batch_normalization_65 (BatchNo (None, 128, 128, 16) 64 conv2d_67[0][0]
__________________________________________________________________________________________________
leaky_re_lu_65 (LeakyReLU) (None, 128, 128, 16) 0 batch_normalization_65[0][0]
__________________________________________________________________________________________________
conv2d_68 (Conv2D) (None, 128, 128, 16) 2320 leaky_re_lu_65[0][0]
__________________________________________________________________________________________________
batch_normalization_66 (BatchNo (None, 128, 128, 16) 64 conv2d_68[0][0]
__________________________________________________________________________________________________
leaky_re_lu_66 (LeakyReLU) (None, 128, 128, 16) 0 batch_normalization_66[0][0]
__________________________________________________________________________________________________
conv2d_69 (Conv2D) (None, 128, 128, 255 4335 leaky_re_lu_66[0][0]
__________________________________________________________________________________________________
batch_normalization_67 (BatchNo (None, 128, 128, 255 1020 conv2d_69[0][0]
__________________________________________________________________________________________________
leaky_re_lu_67 (LeakyReLU) (None, 128, 128, 255 0 batch_normalization_67[0][0]
__________________________________________________________________________________________________
conv2d_70 (Conv2D) (None, 128, 128, 255 65280 leaky_re_lu_67[0][0]
__________________________________________________________________________________________________
batch_normalization_68 (BatchNo (None, 128, 128, 255 1020 conv2d_70[0][0]
__________________________________________________________________________________________________
leaky_re_lu_68 (LeakyReLU) (None, 128, 128, 255 0 batch_normalization_68[0][0]
__________________________________________________________________________________________________
conv2d_71 (Conv2D) (None, 128, 128, 255 65280 leaky_re_lu_68[0][0]
==================================================================================================
Total params: 2,302,087
Trainable params: 2,298,123
Non-trainable params: 3,964
Then I created callbacks on validation loss:
callbacks = [
EarlyStopping(patience=10, verbose=1),
ReduceLROnPlateau(factor=0.1, patience=2, min_lr=0.0000001, verbose=1),
ModelCheckpoint('model.h5', verbose=1, save_best_only=True, save_weights_only=True)
]
results = model.fit_generator(get_data(train_ids, batch_size=2), steps_per_epoch=675 , epochs=100, verbose=1 , callbacks=callbacks, validation_data = get_data(valid_ids,batch_size=1) , validation_steps = 243)
The results came like this:
Epoch 1/100
675/675 [==============================] - 1087s 2s/step - loss: 1.8353 - acc: 0.4686 - val_loss: 3.2239 - val_acc: 0.3724
Epoch 00001: val_loss improved from inf to 3.22391, saving model to model.h5
Epoch 2/100
675/675 [==============================] - 1049s 2s/step - loss: 1.8136 - acc: 0.4762 - val_loss: 1.9655 - val_acc: 0.4165
Epoch 00002: val_loss improved from 3.22391 to 1.96552, saving model to model.h5
Epoch 3/100
675/675 [==============================] - 1041s 2s/step - loss: 1.8003 - acc: 0.4810 - val_loss: 6.6811 - val_acc: 0.2085
Epoch 00003: val_loss did not improve from 1.96552
Epoch 4/100
675/675 [==============================] - 1053s 2s/step - loss: 1.7834 - acc: 0.4863 - val_loss: 10.5597 - val_acc: 0.1563
Epoch 00004: ReduceLROnPlateau reducing learning rate to 0.0009999999776482583.
Epoch 00004: val_loss did not improve from 1.96552
Epoch 5/100
675/675 [==============================] - 1057s 2s/step - loss: 1.7254 - acc: 0.5030 - val_loss: 2.1023 - val_acc: 0.4267
Epoch 00005: val_loss did not improve from 1.96552
Epoch 6/100
675/675 [==============================] - 1052s 2s/step - loss: 1.7088 - acc: 0.5083 - val_loss: 2.1336 - val_acc: 0.4183
Epoch 00006: ReduceLROnPlateau reducing learning rate to 9.999999310821295e-05.
Epoch 00006: val_loss did not improve from 1.96552
Epoch 7/100
675/675 [==============================] - 1052s 2s/step - loss: 1.6963 - acc: 0.5126 - val_loss: 1.6861 - val_acc: 0.5166
Epoch 00007: val_loss improved from 1.96552 to 1.68613, saving model to model.h5
Epoch 8/100
675/675 [==============================] - 1040s 2s/step - loss: 1.6937 - acc: 0.5137 - val_loss: 1.6918 - val_acc: 0.5150
Epoch 00008: val_loss did not improve from 1.68613
Epoch 9/100
675/675 [==============================] - 1019s 2s/step - loss: 1.6928 - acc: 0.5137 - val_loss: 1.6943 - val_acc: 0.5139
Epoch 00009: ReduceLROnPlateau reducing learning rate to 9.999999019782991e-06.
Epoch 00009: val_loss did not improve from 1.68613
Epoch 10/100
454/675 [===================>..........] - ETA: 4:39 - loss: 1.6907 - acc: 0.5154
As you can see the accuracy is not at all improving after later epochs. Loss is decreasing very very slowly. What's wrong with my model? How to get rid of this problem?
Help is appreciated. Thanks in advance.
neural-network deep-learning keras
New contributor
Sank_BE is a new contributor to this site. Take care in asking for clarification, commenting, and answering.
Check out our Code of Conduct.
$endgroup$
So the problem is to perform a multiclass segmentation (255 classes of crops), and I am using a U-Net model for that. The input images are grayscale and the images of dimensions (128,128,1) are grouped into 7 groups (that is 7 channels). The output images are segmented images (128,128,1).
Approach: So I created a dataset for segmentation. Since the input images are to be grouped in 7, I created an input tensor (128,128,7) and the output tensor as (128,128,255) since there are 255 classes and we need this segmentation.
Number of Input:11151 (1593*7) images
Outputs: 1593 images
First I mounted my drive on google colab:
from google.colab import drive
drive.mount('/content/drive')
Go to this URL in a browser: https://accounts.google.com/o/oauth2/auth?client_id=947318989803-6bn6qk8qdgf4n4g3pfee6491hc0brc4i.apps.googleusercontent.com&redirect_uri=urn%3Aietf%3Awg%3Aoauth%3A2.0%3Aoob&scope=email%20https%3A%2F%2Fwww.googleapis.com%2Fauth%2Fdocs.test%20https%3A%2F%2Fwww.googleapis.com%2Fauth%2Fdrive%20https%3A%2F%2Fwww.googleapis.com%2Fauth%2Fdrive.photos.readonly%20https%3A%2F%2Fwww.googleapis.com%2Fauth%2Fpeopleapi.readonly&response_type=code
Enter your authorization code:
··········
Mounted at /content/drive
Then I loaded the libraries:
import os
import random
import pandas as pd
import numpy as np
import matplotlib.pyplot as plt
plt.style.use("ggplot")
%matplotlib inline
from itertools import chain
from skimage.io import imread, imshow, concatenate_images
from skimage.transform import resize
from skimage.morphology import label
from sklearn.model_selection import train_test_split
import tensorflow as tf
from keras.models import Model, load_model
from keras.layers import Input, BatchNormalization, Activation, Dense, Dropout
from keras.layers.core import Lambda, RepeatVector, Reshape
from keras.layers.convolutional import Conv2D, Conv2DTranspose
from keras.layers.pooling import MaxPooling2D, GlobalMaxPool2D
from keras.layers.merge import concatenate, add
from keras.callbacks import EarlyStopping, ModelCheckpoint, ReduceLROnPlateau
from keras.optimizers import Adam,SGD
from keras.preprocessing.image import ImageDataGenerator, array_to_img, img_to_array, load_img
from keras.layers import LeakyReLU
Then I divieded the dataset into training and validation:
im_width = 128
im_height = 128
border = 5
path_train_input = '/content/drive/path....'
path_train_output = '/content/drive/path..'
ids = next(os.walk(path_train_output))[2]
print(len(ids))
from random import shuffle
shuffle(ids)
train_ids = ids[0:1350]
valid_ids = ids[1350:]
print(len(train_ids))
I created this function to load the data in batches to avoid memory crashes in colab:
def get_data(ids,batch_size):
while True:
ids_batches = [ids[i:min(i+batch_size,len(ids))] for i in range(0, len(ids), batch_size)]
#ids_out_batches = [ids_out[j:min(j+batch_size,len(ids_out))] for j in range(0, len(ids_out), batch_size)]
# Load images
for b in range(len(ids_batches)):
k=-1
X = np.zeros((len(ids_batches[b]), im_height, im_width, 7), dtype=np.float32)
y = np.zeros((len(ids_batches[b]), im_height, im_width, 255), dtype=np.float32)
for c in range(len(ids_batches[b])):
k=k+1
#temp1=np.zeros((1,7),dtype=np.float32)
for r in range(1,8):
img = load_img(path_train_input + 'lc8' + ids_batches[b][c][3:-4] + '_' + str(r) + '.tif', color_mode="grayscale")
x_img = img_to_array(img)
x_img = resize(x_img, (128, 128), mode='constant', preserve_range=True)
for p in range(128):
for q in range(128):
X[k][p][q][r-1]=x_img[p][q]/255
#k=k+1
# Save images
#X[k, ..., 0] = temp1 / 255
# Load masks
mask = img_to_array(load_img(path_train_output+ids_batches[b][c], color_mode="grayscale"))
mask = resize(mask, (128, 128), mode='constant', preserve_range=True)
for p in range(128):
for q in range(128):
num=int(mask[p][q])
temp=np.zeros((255), dtype=np.float32)
temp[num]=1
y[k][p][q]=temp
yield X,y
Then I started creating my model:
def conv2d_block(input_tensor, n_filters, kernel_size=3, batchnorm=True):
# first layer
x = Conv2D(filters=n_filters, kernel_size=(kernel_size, kernel_size), kernel_initializer="he_normal",
padding="same")(input_tensor)
if batchnorm:
x = BatchNormalization()(x)
x = LeakyReLU(alpha=0.3)(x)
# second layer
x = Conv2D(filters=n_filters, kernel_size=(kernel_size, kernel_size), kernel_initializer="he_normal",
padding="same")(x)
if batchnorm:
x = BatchNormalization()(x)
x = LeakyReLU(alpha=0.3)(x)
return x
Then the U-Net:
def get_unet(input_img, n_filters=16, dropout=0.15, batchnorm=True):
# contracting path
c1 = conv2d_block(input_img, n_filters=n_filters*1, kernel_size=3, batchnorm=batchnorm)
p1 = MaxPooling2D((2, 2)) (c1)
p1 = Dropout(rate=dropout*0.5)(p1)
c2 = conv2d_block(p1, n_filters=n_filters*2, kernel_size=3, batchnorm=batchnorm)
p2 = MaxPooling2D((2, 2)) (c2)
p2 = Dropout(rate=dropout)(p2)
c3 = conv2d_block(p2, n_filters=n_filters*4, kernel_size=3, batchnorm=batchnorm)
p3 = MaxPooling2D((2, 2)) (c3)
p3 = Dropout(rate=dropout)(p3)
c4 = conv2d_block(p3, n_filters=n_filters*8, kernel_size=3, batchnorm=batchnorm)
p4 = MaxPooling2D(pool_size=(2, 2)) (c4)
p4 = Dropout(rate=dropout)(p4)
c5 = conv2d_block(p4, n_filters=n_filters*16, kernel_size=3, batchnorm=batchnorm)
# expansive path
u6 = Conv2DTranspose(n_filters*8, (3, 3), strides=(2, 2), padding='same') (c5)
u6 = concatenate([u6, c4])
u6 = Dropout(rate=dropout)(u6)
c6 = conv2d_block(u6, n_filters=n_filters*8, kernel_size=3, batchnorm=batchnorm)
u7 = Conv2DTranspose(n_filters*4, (3, 3), strides=(2, 2), padding='same') (c6)
u7 = concatenate([u7, c3])
u7 = Dropout(rate=dropout)(u7)
c7 = conv2d_block(u7, n_filters=n_filters*4, kernel_size=3, batchnorm=batchnorm)
u8 = Conv2DTranspose(n_filters*2, (3, 3), strides=(2, 2), padding='same') (c7)
u8 = concatenate([u8, c2])
u8 = Dropout(rate=dropout)(u8)
c8 = conv2d_block(u8, n_filters=n_filters*2, kernel_size=3, batchnorm=batchnorm)
u9 = Conv2DTranspose(n_filters*1, (3, 3), strides=(2, 2), padding='same') (c8)
u9 = concatenate([u9, c1], axis=3)
u9 = Dropout(rate=dropout)(u9)
c9 = conv2d_block(u9, n_filters=n_filters*1, kernel_size=3, batchnorm=batchnorm)
c10 = conv2d_block(c9, n_filters=255, kernel_size=1, batchnorm=batchnorm)
#conv6 = core.Reshape((1,128,128))(c9)
#conv6 = core.Permute((2,1))(conv6)
outputs = Conv2D(255, (1, 1), activation='softmax') (c10)
model = Model(inputs=[input_img], outputs=[outputs])
return model
input_img = Input((im_height, im_width, 7), name='img')
model = get_unet(input_img, n_filters=16, dropout=0.15, batchnorm=True)
#model = get_unet()
model.compile(optimizer=Adam(lr=0.01), loss="categorical_crossentropy", metrics=["accuracy"])
model.summary()
As you can see in the model summary input is (128,128,7) and output (128,128,255):
__________________________________________________________________________________________________
Layer (type) Output Shape Param # Connected to
==================================================================================================
img (InputLayer) (None, 128, 128, 7) 0
__________________________________________________________________________________________________
conv2d_51 (Conv2D) (None, 128, 128, 16) 1024 img[0][0]
__________________________________________________________________________________________________
batch_normalization_49 (BatchNo (None, 128, 128, 16) 64 conv2d_51[0][0]
__________________________________________________________________________________________________
leaky_re_lu_49 (LeakyReLU) (None, 128, 128, 16) 0 batch_normalization_49[0][0]
__________________________________________________________________________________________________
conv2d_52 (Conv2D) (None, 128, 128, 16) 2320 leaky_re_lu_49[0][0]
__________________________________________________________________________________________________
batch_normalization_50 (BatchNo (None, 128, 128, 16) 64 conv2d_52[0][0]
__________________________________________________________________________________________________
leaky_re_lu_50 (LeakyReLU) (None, 128, 128, 16) 0 batch_normalization_50[0][0]
__________________________________________________________________________________________________
max_pooling2d_11 (MaxPooling2D) (None, 64, 64, 16) 0 leaky_re_lu_50[0][0]
__________________________________________________________________________________________________
dropout_21 (Dropout) (None, 64, 64, 16) 0 max_pooling2d_11[0][0]
__________________________________________________________________________________________________
conv2d_53 (Conv2D) (None, 64, 64, 32) 4640 dropout_21[0][0]
__________________________________________________________________________________________________
batch_normalization_51 (BatchNo (None, 64, 64, 32) 128 conv2d_53[0][0]
__________________________________________________________________________________________________
leaky_re_lu_51 (LeakyReLU) (None, 64, 64, 32) 0 batch_normalization_51[0][0]
__________________________________________________________________________________________________
conv2d_54 (Conv2D) (None, 64, 64, 32) 9248 leaky_re_lu_51[0][0]
__________________________________________________________________________________________________
batch_normalization_52 (BatchNo (None, 64, 64, 32) 128 conv2d_54[0][0]
__________________________________________________________________________________________________
leaky_re_lu_52 (LeakyReLU) (None, 64, 64, 32) 0 batch_normalization_52[0][0]
__________________________________________________________________________________________________
max_pooling2d_12 (MaxPooling2D) (None, 32, 32, 32) 0 leaky_re_lu_52[0][0]
__________________________________________________________________________________________________
dropout_22 (Dropout) (None, 32, 32, 32) 0 max_pooling2d_12[0][0]
__________________________________________________________________________________________________
conv2d_55 (Conv2D) (None, 32, 32, 64) 18496 dropout_22[0][0]
__________________________________________________________________________________________________
batch_normalization_53 (BatchNo (None, 32, 32, 64) 256 conv2d_55[0][0]
__________________________________________________________________________________________________
leaky_re_lu_53 (LeakyReLU) (None, 32, 32, 64) 0 batch_normalization_53[0][0]
__________________________________________________________________________________________________
conv2d_56 (Conv2D) (None, 32, 32, 64) 36928 leaky_re_lu_53[0][0]
__________________________________________________________________________________________________
batch_normalization_54 (BatchNo (None, 32, 32, 64) 256 conv2d_56[0][0]
__________________________________________________________________________________________________
leaky_re_lu_54 (LeakyReLU) (None, 32, 32, 64) 0 batch_normalization_54[0][0]
__________________________________________________________________________________________________
max_pooling2d_13 (MaxPooling2D) (None, 16, 16, 64) 0 leaky_re_lu_54[0][0]
__________________________________________________________________________________________________
dropout_23 (Dropout) (None, 16, 16, 64) 0 max_pooling2d_13[0][0]
__________________________________________________________________________________________________
conv2d_57 (Conv2D) (None, 16, 16, 128) 73856 dropout_23[0][0]
__________________________________________________________________________________________________
batch_normalization_55 (BatchNo (None, 16, 16, 128) 512 conv2d_57[0][0]
__________________________________________________________________________________________________
leaky_re_lu_55 (LeakyReLU) (None, 16, 16, 128) 0 batch_normalization_55[0][0]
__________________________________________________________________________________________________
conv2d_58 (Conv2D) (None, 16, 16, 128) 147584 leaky_re_lu_55[0][0]
__________________________________________________________________________________________________
batch_normalization_56 (BatchNo (None, 16, 16, 128) 512 conv2d_58[0][0]
__________________________________________________________________________________________________
leaky_re_lu_56 (LeakyReLU) (None, 16, 16, 128) 0 batch_normalization_56[0][0]
__________________________________________________________________________________________________
max_pooling2d_14 (MaxPooling2D) (None, 8, 8, 128) 0 leaky_re_lu_56[0][0]
__________________________________________________________________________________________________
dropout_24 (Dropout) (None, 8, 8, 128) 0 max_pooling2d_14[0][0]
__________________________________________________________________________________________________
conv2d_59 (Conv2D) (None, 8, 8, 256) 295168 dropout_24[0][0]
__________________________________________________________________________________________________
batch_normalization_57 (BatchNo (None, 8, 8, 256) 1024 conv2d_59[0][0]
__________________________________________________________________________________________________
leaky_re_lu_57 (LeakyReLU) (None, 8, 8, 256) 0 batch_normalization_57[0][0]
__________________________________________________________________________________________________
conv2d_60 (Conv2D) (None, 8, 8, 256) 590080 leaky_re_lu_57[0][0]
__________________________________________________________________________________________________
batch_normalization_58 (BatchNo (None, 8, 8, 256) 1024 conv2d_60[0][0]
__________________________________________________________________________________________________
leaky_re_lu_58 (LeakyReLU) (None, 8, 8, 256) 0 batch_normalization_58[0][0]
__________________________________________________________________________________________________
conv2d_transpose_11 (Conv2DTran (None, 16, 16, 128) 295040 leaky_re_lu_58[0][0]
__________________________________________________________________________________________________
concatenate_11 (Concatenate) (None, 16, 16, 256) 0 conv2d_transpose_11[0][0]
leaky_re_lu_56[0][0]
__________________________________________________________________________________________________
dropout_25 (Dropout) (None, 16, 16, 256) 0 concatenate_11[0][0]
__________________________________________________________________________________________________
conv2d_61 (Conv2D) (None, 16, 16, 128) 295040 dropout_25[0][0]
__________________________________________________________________________________________________
batch_normalization_59 (BatchNo (None, 16, 16, 128) 512 conv2d_61[0][0]
__________________________________________________________________________________________________
leaky_re_lu_59 (LeakyReLU) (None, 16, 16, 128) 0 batch_normalization_59[0][0]
__________________________________________________________________________________________________
conv2d_62 (Conv2D) (None, 16, 16, 128) 147584 leaky_re_lu_59[0][0]
__________________________________________________________________________________________________
batch_normalization_60 (BatchNo (None, 16, 16, 128) 512 conv2d_62[0][0]
__________________________________________________________________________________________________
leaky_re_lu_60 (LeakyReLU) (None, 16, 16, 128) 0 batch_normalization_60[0][0]
__________________________________________________________________________________________________
conv2d_transpose_12 (Conv2DTran (None, 32, 32, 64) 73792 leaky_re_lu_60[0][0]
__________________________________________________________________________________________________
concatenate_12 (Concatenate) (None, 32, 32, 128) 0 conv2d_transpose_12[0][0]
leaky_re_lu_54[0][0]
__________________________________________________________________________________________________
dropout_26 (Dropout) (None, 32, 32, 128) 0 concatenate_12[0][0]
__________________________________________________________________________________________________
conv2d_63 (Conv2D) (None, 32, 32, 64) 73792 dropout_26[0][0]
__________________________________________________________________________________________________
batch_normalization_61 (BatchNo (None, 32, 32, 64) 256 conv2d_63[0][0]
__________________________________________________________________________________________________
leaky_re_lu_61 (LeakyReLU) (None, 32, 32, 64) 0 batch_normalization_61[0][0]
__________________________________________________________________________________________________
conv2d_64 (Conv2D) (None, 32, 32, 64) 36928 leaky_re_lu_61[0][0]
__________________________________________________________________________________________________
batch_normalization_62 (BatchNo (None, 32, 32, 64) 256 conv2d_64[0][0]
__________________________________________________________________________________________________
leaky_re_lu_62 (LeakyReLU) (None, 32, 32, 64) 0 batch_normalization_62[0][0]
__________________________________________________________________________________________________
conv2d_transpose_13 (Conv2DTran (None, 64, 64, 32) 18464 leaky_re_lu_62[0][0]
__________________________________________________________________________________________________
concatenate_13 (Concatenate) (None, 64, 64, 64) 0 conv2d_transpose_13[0][0]
leaky_re_lu_52[0][0]
__________________________________________________________________________________________________
dropout_27 (Dropout) (None, 64, 64, 64) 0 concatenate_13[0][0]
__________________________________________________________________________________________________
conv2d_65 (Conv2D) (None, 64, 64, 32) 18464 dropout_27[0][0]
__________________________________________________________________________________________________
batch_normalization_63 (BatchNo (None, 64, 64, 32) 128 conv2d_65[0][0]
__________________________________________________________________________________________________
leaky_re_lu_63 (LeakyReLU) (None, 64, 64, 32) 0 batch_normalization_63[0][0]
__________________________________________________________________________________________________
conv2d_66 (Conv2D) (None, 64, 64, 32) 9248 leaky_re_lu_63[0][0]
__________________________________________________________________________________________________
batch_normalization_64 (BatchNo (None, 64, 64, 32) 128 conv2d_66[0][0]
__________________________________________________________________________________________________
leaky_re_lu_64 (LeakyReLU) (None, 64, 64, 32) 0 batch_normalization_64[0][0]
__________________________________________________________________________________________________
conv2d_transpose_14 (Conv2DTran (None, 128, 128, 16) 4624 leaky_re_lu_64[0][0]
__________________________________________________________________________________________________
concatenate_14 (Concatenate) (None, 128, 128, 32) 0 conv2d_transpose_14[0][0]
leaky_re_lu_50[0][0]
__________________________________________________________________________________________________
dropout_28 (Dropout) (None, 128, 128, 32) 0 concatenate_14[0][0]
__________________________________________________________________________________________________
conv2d_67 (Conv2D) (None, 128, 128, 16) 4624 dropout_28[0][0]
__________________________________________________________________________________________________
batch_normalization_65 (BatchNo (None, 128, 128, 16) 64 conv2d_67[0][0]
__________________________________________________________________________________________________
leaky_re_lu_65 (LeakyReLU) (None, 128, 128, 16) 0 batch_normalization_65[0][0]
__________________________________________________________________________________________________
conv2d_68 (Conv2D) (None, 128, 128, 16) 2320 leaky_re_lu_65[0][0]
__________________________________________________________________________________________________
batch_normalization_66 (BatchNo (None, 128, 128, 16) 64 conv2d_68[0][0]
__________________________________________________________________________________________________
leaky_re_lu_66 (LeakyReLU) (None, 128, 128, 16) 0 batch_normalization_66[0][0]
__________________________________________________________________________________________________
conv2d_69 (Conv2D) (None, 128, 128, 255 4335 leaky_re_lu_66[0][0]
__________________________________________________________________________________________________
batch_normalization_67 (BatchNo (None, 128, 128, 255 1020 conv2d_69[0][0]
__________________________________________________________________________________________________
leaky_re_lu_67 (LeakyReLU) (None, 128, 128, 255 0 batch_normalization_67[0][0]
__________________________________________________________________________________________________
conv2d_70 (Conv2D) (None, 128, 128, 255 65280 leaky_re_lu_67[0][0]
__________________________________________________________________________________________________
batch_normalization_68 (BatchNo (None, 128, 128, 255 1020 conv2d_70[0][0]
__________________________________________________________________________________________________
leaky_re_lu_68 (LeakyReLU) (None, 128, 128, 255 0 batch_normalization_68[0][0]
__________________________________________________________________________________________________
conv2d_71 (Conv2D) (None, 128, 128, 255 65280 leaky_re_lu_68[0][0]
==================================================================================================
Total params: 2,302,087
Trainable params: 2,298,123
Non-trainable params: 3,964
Then I created callbacks on validation loss:
callbacks = [
EarlyStopping(patience=10, verbose=1),
ReduceLROnPlateau(factor=0.1, patience=2, min_lr=0.0000001, verbose=1),
ModelCheckpoint('model.h5', verbose=1, save_best_only=True, save_weights_only=True)
]
results = model.fit_generator(get_data(train_ids, batch_size=2), steps_per_epoch=675 , epochs=100, verbose=1 , callbacks=callbacks, validation_data = get_data(valid_ids,batch_size=1) , validation_steps = 243)
The results came like this:
Epoch 1/100
675/675 [==============================] - 1087s 2s/step - loss: 1.8353 - acc: 0.4686 - val_loss: 3.2239 - val_acc: 0.3724
Epoch 00001: val_loss improved from inf to 3.22391, saving model to model.h5
Epoch 2/100
675/675 [==============================] - 1049s 2s/step - loss: 1.8136 - acc: 0.4762 - val_loss: 1.9655 - val_acc: 0.4165
Epoch 00002: val_loss improved from 3.22391 to 1.96552, saving model to model.h5
Epoch 3/100
675/675 [==============================] - 1041s 2s/step - loss: 1.8003 - acc: 0.4810 - val_loss: 6.6811 - val_acc: 0.2085
Epoch 00003: val_loss did not improve from 1.96552
Epoch 4/100
675/675 [==============================] - 1053s 2s/step - loss: 1.7834 - acc: 0.4863 - val_loss: 10.5597 - val_acc: 0.1563
Epoch 00004: ReduceLROnPlateau reducing learning rate to 0.0009999999776482583.
Epoch 00004: val_loss did not improve from 1.96552
Epoch 5/100
675/675 [==============================] - 1057s 2s/step - loss: 1.7254 - acc: 0.5030 - val_loss: 2.1023 - val_acc: 0.4267
Epoch 00005: val_loss did not improve from 1.96552
Epoch 6/100
675/675 [==============================] - 1052s 2s/step - loss: 1.7088 - acc: 0.5083 - val_loss: 2.1336 - val_acc: 0.4183
Epoch 00006: ReduceLROnPlateau reducing learning rate to 9.999999310821295e-05.
Epoch 00006: val_loss did not improve from 1.96552
Epoch 7/100
675/675 [==============================] - 1052s 2s/step - loss: 1.6963 - acc: 0.5126 - val_loss: 1.6861 - val_acc: 0.5166
Epoch 00007: val_loss improved from 1.96552 to 1.68613, saving model to model.h5
Epoch 8/100
675/675 [==============================] - 1040s 2s/step - loss: 1.6937 - acc: 0.5137 - val_loss: 1.6918 - val_acc: 0.5150
Epoch 00008: val_loss did not improve from 1.68613
Epoch 9/100
675/675 [==============================] - 1019s 2s/step - loss: 1.6928 - acc: 0.5137 - val_loss: 1.6943 - val_acc: 0.5139
Epoch 00009: ReduceLROnPlateau reducing learning rate to 9.999999019782991e-06.
Epoch 00009: val_loss did not improve from 1.68613
Epoch 10/100
454/675 [===================>..........] - ETA: 4:39 - loss: 1.6907 - acc: 0.5154
As you can see the accuracy is not at all improving after later epochs. Loss is decreasing very very slowly. What's wrong with my model? How to get rid of this problem?
Help is appreciated. Thanks in advance.
neural-network deep-learning keras
neural-network deep-learning keras
New contributor
Sank_BE is a new contributor to this site. Take care in asking for clarification, commenting, and answering.
Check out our Code of Conduct.
New contributor
Sank_BE is a new contributor to this site. Take care in asking for clarification, commenting, and answering.
Check out our Code of Conduct.
edited 2 days ago
Sank_BE
New contributor
Sank_BE is a new contributor to this site. Take care in asking for clarification, commenting, and answering.
Check out our Code of Conduct.
asked 2 days ago
Sank_BESank_BE
11
11
New contributor
Sank_BE is a new contributor to this site. Take care in asking for clarification, commenting, and answering.
Check out our Code of Conduct.
New contributor
Sank_BE is a new contributor to this site. Take care in asking for clarification, commenting, and answering.
Check out our Code of Conduct.
Sank_BE is a new contributor to this site. Take care in asking for clarification, commenting, and answering.
Check out our Code of Conduct.
$begingroup$
Anyone please help me? How can I set bounty on this question? I see no such option below.
$endgroup$
– Sank_BE
12 hours ago
add a comment |
$begingroup$
Anyone please help me? How can I set bounty on this question? I see no such option below.
$endgroup$
– Sank_BE
12 hours ago
$begingroup$
Anyone please help me? How can I set bounty on this question? I see no such option below.
$endgroup$
– Sank_BE
12 hours ago
$begingroup$
Anyone please help me? How can I set bounty on this question? I see no such option below.
$endgroup$
– Sank_BE
12 hours ago
add a comment |
0
active
oldest
votes
Your Answer
StackExchange.ifUsing("editor", function () {
return StackExchange.using("mathjaxEditing", function () {
StackExchange.MarkdownEditor.creationCallbacks.add(function (editor, postfix) {
StackExchange.mathjaxEditing.prepareWmdForMathJax(editor, postfix, [["$", "$"], ["\\(","\\)"]]);
});
});
}, "mathjax-editing");
StackExchange.ready(function() {
var channelOptions = {
tags: "".split(" "),
id: "557"
};
initTagRenderer("".split(" "), "".split(" "), channelOptions);
StackExchange.using("externalEditor", function() {
// Have to fire editor after snippets, if snippets enabled
if (StackExchange.settings.snippets.snippetsEnabled) {
StackExchange.using("snippets", function() {
createEditor();
});
}
else {
createEditor();
}
});
function createEditor() {
StackExchange.prepareEditor({
heartbeatType: 'answer',
autoActivateHeartbeat: false,
convertImagesToLinks: false,
noModals: true,
showLowRepImageUploadWarning: true,
reputationToPostImages: null,
bindNavPrevention: true,
postfix: "",
imageUploader: {
brandingHtml: "Powered by u003ca class="icon-imgur-white" href="https://imgur.com/"u003eu003c/au003e",
contentPolicyHtml: "User contributions licensed under u003ca href="https://creativecommons.org/licenses/by-sa/3.0/"u003ecc by-sa 3.0 with attribution requiredu003c/au003e u003ca href="https://stackoverflow.com/legal/content-policy"u003e(content policy)u003c/au003e",
allowUrls: true
},
onDemand: true,
discardSelector: ".discard-answer"
,immediatelyShowMarkdownHelp:true
});
}
});
Sank_BE is a new contributor. Be nice, and check out our Code of Conduct.
Sign up or log in
StackExchange.ready(function () {
StackExchange.helpers.onClickDraftSave('#login-link');
});
Sign up using Google
Sign up using Facebook
Sign up using Email and Password
Post as a guest
Required, but never shown
StackExchange.ready(
function () {
StackExchange.openid.initPostLogin('.new-post-login', 'https%3a%2f%2fdatascience.stackexchange.com%2fquestions%2f47044%2fmulticlass-segmentation-using-u-net-my-training-loss-is-not-decreasing-after-ce%23new-answer', 'question_page');
}
);
Post as a guest
Required, but never shown
0
active
oldest
votes
0
active
oldest
votes
active
oldest
votes
active
oldest
votes
Sank_BE is a new contributor. Be nice, and check out our Code of Conduct.
Sank_BE is a new contributor. Be nice, and check out our Code of Conduct.
Sank_BE is a new contributor. Be nice, and check out our Code of Conduct.
Sank_BE is a new contributor. Be nice, and check out our Code of Conduct.
Thanks for contributing an answer to Data Science Stack Exchange!
- Please be sure to answer the question. Provide details and share your research!
But avoid …
- Asking for help, clarification, or responding to other answers.
- Making statements based on opinion; back them up with references or personal experience.
Use MathJax to format equations. MathJax reference.
To learn more, see our tips on writing great answers.
Sign up or log in
StackExchange.ready(function () {
StackExchange.helpers.onClickDraftSave('#login-link');
});
Sign up using Google
Sign up using Facebook
Sign up using Email and Password
Post as a guest
Required, but never shown
StackExchange.ready(
function () {
StackExchange.openid.initPostLogin('.new-post-login', 'https%3a%2f%2fdatascience.stackexchange.com%2fquestions%2f47044%2fmulticlass-segmentation-using-u-net-my-training-loss-is-not-decreasing-after-ce%23new-answer', 'question_page');
}
);
Post as a guest
Required, but never shown
Sign up or log in
StackExchange.ready(function () {
StackExchange.helpers.onClickDraftSave('#login-link');
});
Sign up using Google
Sign up using Facebook
Sign up using Email and Password
Post as a guest
Required, but never shown
Sign up or log in
StackExchange.ready(function () {
StackExchange.helpers.onClickDraftSave('#login-link');
});
Sign up using Google
Sign up using Facebook
Sign up using Email and Password
Post as a guest
Required, but never shown
Sign up or log in
StackExchange.ready(function () {
StackExchange.helpers.onClickDraftSave('#login-link');
});
Sign up using Google
Sign up using Facebook
Sign up using Email and Password
Sign up using Google
Sign up using Facebook
Sign up using Email and Password
Post as a guest
Required, but never shown
Required, but never shown
Required, but never shown
Required, but never shown
Required, but never shown
Required, but never shown
Required, but never shown
Required, but never shown
Required, but never shown
kXpMgcoijlB1StE1jPxaOz0isnfPJipMPrAFik9W,AbjltxV4Vil 0V,ibblyM5y,o
$begingroup$
Anyone please help me? How can I set bounty on this question? I see no such option below.
$endgroup$
– Sank_BE
12 hours ago