Woden
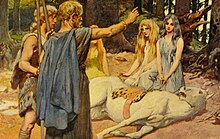
Woden Equum Balderanum Curat, pictura Aemilii Doepler (1855–1922).
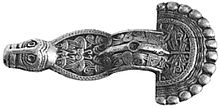
Fibula Nordendorfensis II.
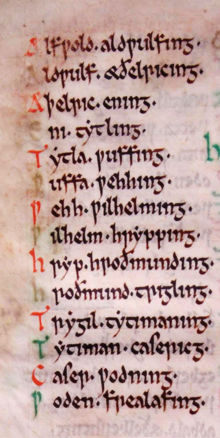
Woden progenies Ælfwald Angliae Orientalis in Textu Roffensi (saeculum 12) perscribitur. Vide versum ultimum.
Woden vel Wodan (lingua Anglica antiqua Ƿōden,[1]lingua Theodisca alta antiqua Wôdan,[2]lingua Saxonica antiqua Uuôden[3]) est maior deitas Anglosaxonica et Germanica continentalis. Qui, cum Odin, eius pari Nordico,[4] progressum *Wōđanaz, dei Protogermanici, repraesentat. Ex eo appellatur Wednesday, dies Anglicus pro die Mercurii.
Nexus interni
- Christianitas Germanica
- Paganismus Anglosaxonicus
- Polytheismus Anglosaxonicus
Notae |
↑ David Wilson, Anglo-Saxon Paganism (Routledge, 1992, ISBN 978-0-415-01897-5), 11.
↑ Brian Murdoch, ed., German Literature of the Early Middle Ages (Camden House Publishing, 2004, ISBN 978-1-57113-240-6), 62.
↑ Edward Turville-Petre, Myth and Religion of the North: The Religion of Ancient Scandinavia (Greenwood Press, 1975, ISBN 978-0-8371-7420-4), 100.
↑ Ellis Davidson, Myths and Symbols in Pagan Europe: Early Scandinavian and Celtic Religions (Manchester University Press, 1989, 978-0-7190-2579-2), 1.
Bibliographia |
- Branston, Brian. 1974. The Lost Gods of England. Ed. 2a. Londinii: Thames and Hudson. ISBN 0-500-11013-1.
- Herbert, Kathleen. 1995. Looking for the Lost Gods of England. Anglo-Saxon Books. ISBN 1-898281-04-1.
- Pettit, E. 2001. Anglo-Saxon Remedies, Charms, and Prayers from British Library MS Harley 585: The 'Lacnunga.' 2 vol. Edwin Mellen Press.
- Stanley, E. G. 2000. Imagining the Anglo-Saxon Past: The Search for Anglo-Saxon Paganism and Anglo-Saxon Trial by Jury. D. S. Brewer. ISBN 0-85991-588-3.
- Wood, Michael. 2001. In Search of the Dark Ages. Checkmark Books. ISBN 0-8160-4702-2.
- Walter Keating Kelly. 1863. Curiosities of Indo-European Tradition and Folk-lore. Londinii: Chapman &, 266–291.