Keras NN - Learning a simple formula
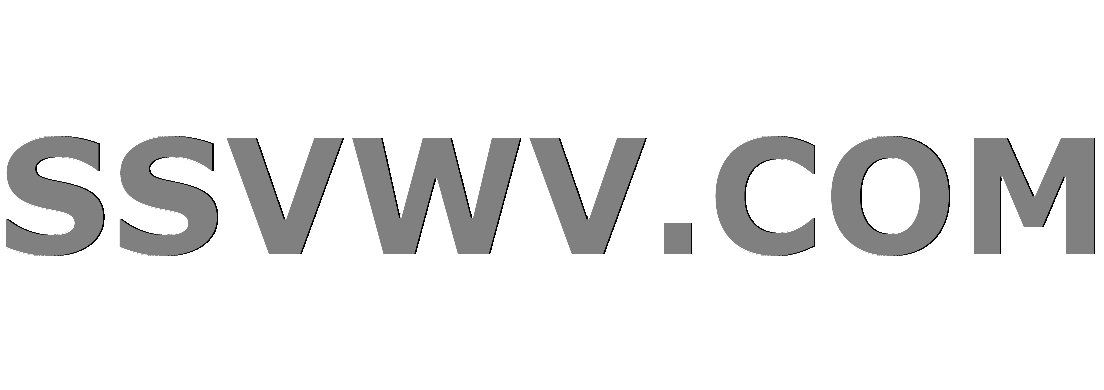
Multi tool use
$begingroup$
I'm struggling with a seemingly simple problem and could really use your help! I'm starting to learn about neural networks. I thought I would create a simple example to get started, using one dimensional inputs X and one dimensional outputs Y. When I first started with Y = 2X, the neural network was able to learn it very well over 1000 epochs. However, when I increased the complexity of the formula to below, it completely breaks apart (the MSE is very high).
X = random numbers between 0 and 100 (one-dimensional)
Y = (8(x^3)) + 5
I have tried adding more hidden layers and epochs without much success. Could anyone provide me with some guidance on where I'm going wrong in my method?
Much appreciated!
Code:
from keras.models import Sequential
from keras.layers import Dense
import numpy as np
# fix random seed for reproducibility
np.random.seed(7)
def generate_Y(X):
return np.add(np.power(np.multiply(X, 2.0), 3.0), 5.0).T[0]
X = np.random.rand(100,1)
X = np.multiply(X, 100.0)
Y = generate_Y(X)
# create model
model = Sequential()
model.add(Dense(12, input_dim=1))
model.add(Dense(12))
model.add(Dense(12))
model.add(Dense(12))
model.add(Dense(1))
# Compile model
model.compile(loss='mean_squared_error', optimizer='adam', metrics=['mse'])
# Fit the model
model.fit(X, Y, epochs=10000, batch_size=10)
# evaluate the model
scores = model.evaluate(X, Y)
print("n%s: %.2f%%" % (model.metrics_names[1], scores[1]*100))
test_X = np.random.rand(10,1)
test_X = np.multiply(test_X, 500.0)
test_Y = generate_Y(test_X)
preds = model.predict(test_X)
print preds
diffs = np.subtract(preds.T[0], test_Y)
print diffs
MSE: mean_squared_error: 73360074604544.00%
Prediction vs actual diffs:
[ 4.97601229e+05 -1.60322447e+06 -7.85835700e+08 -7.00977203e+08
-7.52094155e+08 -1.88780991e+08 -1.64643340e+08 -9.38229901e+08
-2.02173897e+08 4.45327360e+05]
python neural-network keras
New contributor
RB25 is a new contributor to this site. Take care in asking for clarification, commenting, and answering.
Check out our Code of Conduct.
$endgroup$
add a comment |
$begingroup$
I'm struggling with a seemingly simple problem and could really use your help! I'm starting to learn about neural networks. I thought I would create a simple example to get started, using one dimensional inputs X and one dimensional outputs Y. When I first started with Y = 2X, the neural network was able to learn it very well over 1000 epochs. However, when I increased the complexity of the formula to below, it completely breaks apart (the MSE is very high).
X = random numbers between 0 and 100 (one-dimensional)
Y = (8(x^3)) + 5
I have tried adding more hidden layers and epochs without much success. Could anyone provide me with some guidance on where I'm going wrong in my method?
Much appreciated!
Code:
from keras.models import Sequential
from keras.layers import Dense
import numpy as np
# fix random seed for reproducibility
np.random.seed(7)
def generate_Y(X):
return np.add(np.power(np.multiply(X, 2.0), 3.0), 5.0).T[0]
X = np.random.rand(100,1)
X = np.multiply(X, 100.0)
Y = generate_Y(X)
# create model
model = Sequential()
model.add(Dense(12, input_dim=1))
model.add(Dense(12))
model.add(Dense(12))
model.add(Dense(12))
model.add(Dense(1))
# Compile model
model.compile(loss='mean_squared_error', optimizer='adam', metrics=['mse'])
# Fit the model
model.fit(X, Y, epochs=10000, batch_size=10)
# evaluate the model
scores = model.evaluate(X, Y)
print("n%s: %.2f%%" % (model.metrics_names[1], scores[1]*100))
test_X = np.random.rand(10,1)
test_X = np.multiply(test_X, 500.0)
test_Y = generate_Y(test_X)
preds = model.predict(test_X)
print preds
diffs = np.subtract(preds.T[0], test_Y)
print diffs
MSE: mean_squared_error: 73360074604544.00%
Prediction vs actual diffs:
[ 4.97601229e+05 -1.60322447e+06 -7.85835700e+08 -7.00977203e+08
-7.52094155e+08 -1.88780991e+08 -1.64643340e+08 -9.38229901e+08
-2.02173897e+08 4.45327360e+05]
python neural-network keras
New contributor
RB25 is a new contributor to this site. Take care in asking for clarification, commenting, and answering.
Check out our Code of Conduct.
$endgroup$
$begingroup$
You are not using any of the activation functions like sigmoid or ReLU.
$endgroup$
– Shubham Panchal
yesterday
$begingroup$
I thought the sigmoid function was better used for classification rather than regression problems? I tried adding those activation functions and it didn't improve the result.
$endgroup$
– RB25
yesterday
$begingroup$
The formula you are trying to learn is clearly non linear, therefore you have to use some non linearity activation as @shubam Panchal suggested. Please show the code after this update.
$endgroup$
– Francesco Pegoraro
yesterday
$begingroup$
andgenerate_Y
returns just a value... are you shure about the usage of.T[0]
?
$endgroup$
– Francesco Pegoraro
yesterday
add a comment |
$begingroup$
I'm struggling with a seemingly simple problem and could really use your help! I'm starting to learn about neural networks. I thought I would create a simple example to get started, using one dimensional inputs X and one dimensional outputs Y. When I first started with Y = 2X, the neural network was able to learn it very well over 1000 epochs. However, when I increased the complexity of the formula to below, it completely breaks apart (the MSE is very high).
X = random numbers between 0 and 100 (one-dimensional)
Y = (8(x^3)) + 5
I have tried adding more hidden layers and epochs without much success. Could anyone provide me with some guidance on where I'm going wrong in my method?
Much appreciated!
Code:
from keras.models import Sequential
from keras.layers import Dense
import numpy as np
# fix random seed for reproducibility
np.random.seed(7)
def generate_Y(X):
return np.add(np.power(np.multiply(X, 2.0), 3.0), 5.0).T[0]
X = np.random.rand(100,1)
X = np.multiply(X, 100.0)
Y = generate_Y(X)
# create model
model = Sequential()
model.add(Dense(12, input_dim=1))
model.add(Dense(12))
model.add(Dense(12))
model.add(Dense(12))
model.add(Dense(1))
# Compile model
model.compile(loss='mean_squared_error', optimizer='adam', metrics=['mse'])
# Fit the model
model.fit(X, Y, epochs=10000, batch_size=10)
# evaluate the model
scores = model.evaluate(X, Y)
print("n%s: %.2f%%" % (model.metrics_names[1], scores[1]*100))
test_X = np.random.rand(10,1)
test_X = np.multiply(test_X, 500.0)
test_Y = generate_Y(test_X)
preds = model.predict(test_X)
print preds
diffs = np.subtract(preds.T[0], test_Y)
print diffs
MSE: mean_squared_error: 73360074604544.00%
Prediction vs actual diffs:
[ 4.97601229e+05 -1.60322447e+06 -7.85835700e+08 -7.00977203e+08
-7.52094155e+08 -1.88780991e+08 -1.64643340e+08 -9.38229901e+08
-2.02173897e+08 4.45327360e+05]
python neural-network keras
New contributor
RB25 is a new contributor to this site. Take care in asking for clarification, commenting, and answering.
Check out our Code of Conduct.
$endgroup$
I'm struggling with a seemingly simple problem and could really use your help! I'm starting to learn about neural networks. I thought I would create a simple example to get started, using one dimensional inputs X and one dimensional outputs Y. When I first started with Y = 2X, the neural network was able to learn it very well over 1000 epochs. However, when I increased the complexity of the formula to below, it completely breaks apart (the MSE is very high).
X = random numbers between 0 and 100 (one-dimensional)
Y = (8(x^3)) + 5
I have tried adding more hidden layers and epochs without much success. Could anyone provide me with some guidance on where I'm going wrong in my method?
Much appreciated!
Code:
from keras.models import Sequential
from keras.layers import Dense
import numpy as np
# fix random seed for reproducibility
np.random.seed(7)
def generate_Y(X):
return np.add(np.power(np.multiply(X, 2.0), 3.0), 5.0).T[0]
X = np.random.rand(100,1)
X = np.multiply(X, 100.0)
Y = generate_Y(X)
# create model
model = Sequential()
model.add(Dense(12, input_dim=1))
model.add(Dense(12))
model.add(Dense(12))
model.add(Dense(12))
model.add(Dense(1))
# Compile model
model.compile(loss='mean_squared_error', optimizer='adam', metrics=['mse'])
# Fit the model
model.fit(X, Y, epochs=10000, batch_size=10)
# evaluate the model
scores = model.evaluate(X, Y)
print("n%s: %.2f%%" % (model.metrics_names[1], scores[1]*100))
test_X = np.random.rand(10,1)
test_X = np.multiply(test_X, 500.0)
test_Y = generate_Y(test_X)
preds = model.predict(test_X)
print preds
diffs = np.subtract(preds.T[0], test_Y)
print diffs
MSE: mean_squared_error: 73360074604544.00%
Prediction vs actual diffs:
[ 4.97601229e+05 -1.60322447e+06 -7.85835700e+08 -7.00977203e+08
-7.52094155e+08 -1.88780991e+08 -1.64643340e+08 -9.38229901e+08
-2.02173897e+08 4.45327360e+05]
python neural-network keras
python neural-network keras
New contributor
RB25 is a new contributor to this site. Take care in asking for clarification, commenting, and answering.
Check out our Code of Conduct.
New contributor
RB25 is a new contributor to this site. Take care in asking for clarification, commenting, and answering.
Check out our Code of Conduct.
New contributor
RB25 is a new contributor to this site. Take care in asking for clarification, commenting, and answering.
Check out our Code of Conduct.
asked yesterday
RB25RB25
1
1
New contributor
RB25 is a new contributor to this site. Take care in asking for clarification, commenting, and answering.
Check out our Code of Conduct.
New contributor
RB25 is a new contributor to this site. Take care in asking for clarification, commenting, and answering.
Check out our Code of Conduct.
RB25 is a new contributor to this site. Take care in asking for clarification, commenting, and answering.
Check out our Code of Conduct.
$begingroup$
You are not using any of the activation functions like sigmoid or ReLU.
$endgroup$
– Shubham Panchal
yesterday
$begingroup$
I thought the sigmoid function was better used for classification rather than regression problems? I tried adding those activation functions and it didn't improve the result.
$endgroup$
– RB25
yesterday
$begingroup$
The formula you are trying to learn is clearly non linear, therefore you have to use some non linearity activation as @shubam Panchal suggested. Please show the code after this update.
$endgroup$
– Francesco Pegoraro
yesterday
$begingroup$
andgenerate_Y
returns just a value... are you shure about the usage of.T[0]
?
$endgroup$
– Francesco Pegoraro
yesterday
add a comment |
$begingroup$
You are not using any of the activation functions like sigmoid or ReLU.
$endgroup$
– Shubham Panchal
yesterday
$begingroup$
I thought the sigmoid function was better used for classification rather than regression problems? I tried adding those activation functions and it didn't improve the result.
$endgroup$
– RB25
yesterday
$begingroup$
The formula you are trying to learn is clearly non linear, therefore you have to use some non linearity activation as @shubam Panchal suggested. Please show the code after this update.
$endgroup$
– Francesco Pegoraro
yesterday
$begingroup$
andgenerate_Y
returns just a value... are you shure about the usage of.T[0]
?
$endgroup$
– Francesco Pegoraro
yesterday
$begingroup$
You are not using any of the activation functions like sigmoid or ReLU.
$endgroup$
– Shubham Panchal
yesterday
$begingroup$
You are not using any of the activation functions like sigmoid or ReLU.
$endgroup$
– Shubham Panchal
yesterday
$begingroup$
I thought the sigmoid function was better used for classification rather than regression problems? I tried adding those activation functions and it didn't improve the result.
$endgroup$
– RB25
yesterday
$begingroup$
I thought the sigmoid function was better used for classification rather than regression problems? I tried adding those activation functions and it didn't improve the result.
$endgroup$
– RB25
yesterday
$begingroup$
The formula you are trying to learn is clearly non linear, therefore you have to use some non linearity activation as @shubam Panchal suggested. Please show the code after this update.
$endgroup$
– Francesco Pegoraro
yesterday
$begingroup$
The formula you are trying to learn is clearly non linear, therefore you have to use some non linearity activation as @shubam Panchal suggested. Please show the code after this update.
$endgroup$
– Francesco Pegoraro
yesterday
$begingroup$
and
generate_Y
returns just a value... are you shure about the usage of .T[0]
?$endgroup$
– Francesco Pegoraro
yesterday
$begingroup$
and
generate_Y
returns just a value... are you shure about the usage of .T[0]
?$endgroup$
– Francesco Pegoraro
yesterday
add a comment |
0
active
oldest
votes
Your Answer
StackExchange.ifUsing("editor", function () {
return StackExchange.using("mathjaxEditing", function () {
StackExchange.MarkdownEditor.creationCallbacks.add(function (editor, postfix) {
StackExchange.mathjaxEditing.prepareWmdForMathJax(editor, postfix, [["$", "$"], ["\\(","\\)"]]);
});
});
}, "mathjax-editing");
StackExchange.ready(function() {
var channelOptions = {
tags: "".split(" "),
id: "557"
};
initTagRenderer("".split(" "), "".split(" "), channelOptions);
StackExchange.using("externalEditor", function() {
// Have to fire editor after snippets, if snippets enabled
if (StackExchange.settings.snippets.snippetsEnabled) {
StackExchange.using("snippets", function() {
createEditor();
});
}
else {
createEditor();
}
});
function createEditor() {
StackExchange.prepareEditor({
heartbeatType: 'answer',
autoActivateHeartbeat: false,
convertImagesToLinks: false,
noModals: true,
showLowRepImageUploadWarning: true,
reputationToPostImages: null,
bindNavPrevention: true,
postfix: "",
imageUploader: {
brandingHtml: "Powered by u003ca class="icon-imgur-white" href="https://imgur.com/"u003eu003c/au003e",
contentPolicyHtml: "User contributions licensed under u003ca href="https://creativecommons.org/licenses/by-sa/3.0/"u003ecc by-sa 3.0 with attribution requiredu003c/au003e u003ca href="https://stackoverflow.com/legal/content-policy"u003e(content policy)u003c/au003e",
allowUrls: true
},
onDemand: true,
discardSelector: ".discard-answer"
,immediatelyShowMarkdownHelp:true
});
}
});
RB25 is a new contributor. Be nice, and check out our Code of Conduct.
Sign up or log in
StackExchange.ready(function () {
StackExchange.helpers.onClickDraftSave('#login-link');
});
Sign up using Google
Sign up using Facebook
Sign up using Email and Password
Post as a guest
Required, but never shown
StackExchange.ready(
function () {
StackExchange.openid.initPostLogin('.new-post-login', 'https%3a%2f%2fdatascience.stackexchange.com%2fquestions%2f48797%2fkeras-nn-learning-a-simple-formula%23new-answer', 'question_page');
}
);
Post as a guest
Required, but never shown
0
active
oldest
votes
0
active
oldest
votes
active
oldest
votes
active
oldest
votes
RB25 is a new contributor. Be nice, and check out our Code of Conduct.
RB25 is a new contributor. Be nice, and check out our Code of Conduct.
RB25 is a new contributor. Be nice, and check out our Code of Conduct.
RB25 is a new contributor. Be nice, and check out our Code of Conduct.
Thanks for contributing an answer to Data Science Stack Exchange!
- Please be sure to answer the question. Provide details and share your research!
But avoid …
- Asking for help, clarification, or responding to other answers.
- Making statements based on opinion; back them up with references or personal experience.
Use MathJax to format equations. MathJax reference.
To learn more, see our tips on writing great answers.
Sign up or log in
StackExchange.ready(function () {
StackExchange.helpers.onClickDraftSave('#login-link');
});
Sign up using Google
Sign up using Facebook
Sign up using Email and Password
Post as a guest
Required, but never shown
StackExchange.ready(
function () {
StackExchange.openid.initPostLogin('.new-post-login', 'https%3a%2f%2fdatascience.stackexchange.com%2fquestions%2f48797%2fkeras-nn-learning-a-simple-formula%23new-answer', 'question_page');
}
);
Post as a guest
Required, but never shown
Sign up or log in
StackExchange.ready(function () {
StackExchange.helpers.onClickDraftSave('#login-link');
});
Sign up using Google
Sign up using Facebook
Sign up using Email and Password
Post as a guest
Required, but never shown
Sign up or log in
StackExchange.ready(function () {
StackExchange.helpers.onClickDraftSave('#login-link');
});
Sign up using Google
Sign up using Facebook
Sign up using Email and Password
Post as a guest
Required, but never shown
Sign up or log in
StackExchange.ready(function () {
StackExchange.helpers.onClickDraftSave('#login-link');
});
Sign up using Google
Sign up using Facebook
Sign up using Email and Password
Sign up using Google
Sign up using Facebook
Sign up using Email and Password
Post as a guest
Required, but never shown
Required, but never shown
Required, but never shown
Required, but never shown
Required, but never shown
Required, but never shown
Required, but never shown
Required, but never shown
Required, but never shown
QxHpdJE0Ro6XNMbL7sv,L
$begingroup$
You are not using any of the activation functions like sigmoid or ReLU.
$endgroup$
– Shubham Panchal
yesterday
$begingroup$
I thought the sigmoid function was better used for classification rather than regression problems? I tried adding those activation functions and it didn't improve the result.
$endgroup$
– RB25
yesterday
$begingroup$
The formula you are trying to learn is clearly non linear, therefore you have to use some non linearity activation as @shubam Panchal suggested. Please show the code after this update.
$endgroup$
– Francesco Pegoraro
yesterday
$begingroup$
and
generate_Y
returns just a value... are you shure about the usage of.T[0]
?$endgroup$
– Francesco Pegoraro
yesterday