Python: Fast indexing of strings in nested list without loop
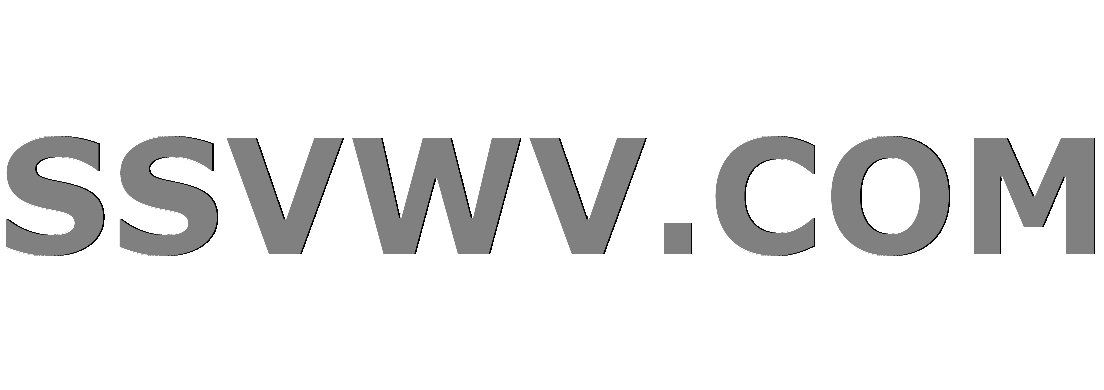
Multi tool use
$begingroup$
I have an array dataset from which I need to build D whose each element is a list of strings (Third column of dataset). The dataset looks like:
600,900,3418309
600,900,3418309
600,900,3418314
600,900,3418314
600,900,3418319
600,900,3418319
610,800,3418324
610,700,3418324
600,900,3418329
620,900,3418329
600,900,3418329
600,900,3418334
610,900,3418334
600,900,3418339
600,900,3418339
600,900,3418339
660,700,3418339
610,800,3418339
660,700,3418339
600,900,3418339
600,900,3418339
I want to check for every new string, and if it is not a part of any of the array element then append new string to only that array element.
Since the data on new strings to be added is large, I do not want to use loop. Is there any method to do it fast. I have to use Python.
Right now I am using the code, which is very slow:
for i in range(len(dataset)):
for j in range(int(int(dataset[i,0]-600)),int(int(dataset[i,1]-600)+1)):
if(str(dataset[i,2]) not in D[j]):
D[j].append(str(dataset[i,2]))
python
$endgroup$
|
show 3 more comments
$begingroup$
I have an array dataset from which I need to build D whose each element is a list of strings (Third column of dataset). The dataset looks like:
600,900,3418309
600,900,3418309
600,900,3418314
600,900,3418314
600,900,3418319
600,900,3418319
610,800,3418324
610,700,3418324
600,900,3418329
620,900,3418329
600,900,3418329
600,900,3418334
610,900,3418334
600,900,3418339
600,900,3418339
600,900,3418339
660,700,3418339
610,800,3418339
660,700,3418339
600,900,3418339
600,900,3418339
I want to check for every new string, and if it is not a part of any of the array element then append new string to only that array element.
Since the data on new strings to be added is large, I do not want to use loop. Is there any method to do it fast. I have to use Python.
Right now I am using the code, which is very slow:
for i in range(len(dataset)):
for j in range(int(int(dataset[i,0]-600)),int(int(dataset[i,1]-600)+1)):
if(str(dataset[i,2]) not in D[j]):
D[j].append(str(dataset[i,2]))
python
$endgroup$
$begingroup$
Welcome to the site! You are certainly welcome to leave your question here, but there are some serious python wizards on Stack Overflow and I'm sure that posting your question there can get you an answer in the matter of minutes.
$endgroup$
– I_Play_With_Data
2 days ago
$begingroup$
Why do you do if then pass them else? You could negate the if and drop the pass/else
$endgroup$
– kbrose
15 hours ago
$begingroup$
@kbrose I have updated the code in question based on your suggestion. But still it is very slow. Can it be further improvised?
$endgroup$
– shaifali Gupta
14 hours ago
$begingroup$
i am not an expert, but pandas couldnt work in this case? They have highly optimized performance for some tasks.
$endgroup$
– Victor Oliveira
14 hours ago
$begingroup$
Have you tried making it a set instead of a list? Sets are optimized for containment checking.
$endgroup$
– kbrose
14 hours ago
|
show 3 more comments
$begingroup$
I have an array dataset from which I need to build D whose each element is a list of strings (Third column of dataset). The dataset looks like:
600,900,3418309
600,900,3418309
600,900,3418314
600,900,3418314
600,900,3418319
600,900,3418319
610,800,3418324
610,700,3418324
600,900,3418329
620,900,3418329
600,900,3418329
600,900,3418334
610,900,3418334
600,900,3418339
600,900,3418339
600,900,3418339
660,700,3418339
610,800,3418339
660,700,3418339
600,900,3418339
600,900,3418339
I want to check for every new string, and if it is not a part of any of the array element then append new string to only that array element.
Since the data on new strings to be added is large, I do not want to use loop. Is there any method to do it fast. I have to use Python.
Right now I am using the code, which is very slow:
for i in range(len(dataset)):
for j in range(int(int(dataset[i,0]-600)),int(int(dataset[i,1]-600)+1)):
if(str(dataset[i,2]) not in D[j]):
D[j].append(str(dataset[i,2]))
python
$endgroup$
I have an array dataset from which I need to build D whose each element is a list of strings (Third column of dataset). The dataset looks like:
600,900,3418309
600,900,3418309
600,900,3418314
600,900,3418314
600,900,3418319
600,900,3418319
610,800,3418324
610,700,3418324
600,900,3418329
620,900,3418329
600,900,3418329
600,900,3418334
610,900,3418334
600,900,3418339
600,900,3418339
600,900,3418339
660,700,3418339
610,800,3418339
660,700,3418339
600,900,3418339
600,900,3418339
I want to check for every new string, and if it is not a part of any of the array element then append new string to only that array element.
Since the data on new strings to be added is large, I do not want to use loop. Is there any method to do it fast. I have to use Python.
Right now I am using the code, which is very slow:
for i in range(len(dataset)):
for j in range(int(int(dataset[i,0]-600)),int(int(dataset[i,1]-600)+1)):
if(str(dataset[i,2]) not in D[j]):
D[j].append(str(dataset[i,2]))
python
python
edited 13 hours ago
shaifali Gupta
asked 2 days ago
shaifali Guptashaifali Gupta
719
719
$begingroup$
Welcome to the site! You are certainly welcome to leave your question here, but there are some serious python wizards on Stack Overflow and I'm sure that posting your question there can get you an answer in the matter of minutes.
$endgroup$
– I_Play_With_Data
2 days ago
$begingroup$
Why do you do if then pass them else? You could negate the if and drop the pass/else
$endgroup$
– kbrose
15 hours ago
$begingroup$
@kbrose I have updated the code in question based on your suggestion. But still it is very slow. Can it be further improvised?
$endgroup$
– shaifali Gupta
14 hours ago
$begingroup$
i am not an expert, but pandas couldnt work in this case? They have highly optimized performance for some tasks.
$endgroup$
– Victor Oliveira
14 hours ago
$begingroup$
Have you tried making it a set instead of a list? Sets are optimized for containment checking.
$endgroup$
– kbrose
14 hours ago
|
show 3 more comments
$begingroup$
Welcome to the site! You are certainly welcome to leave your question here, but there are some serious python wizards on Stack Overflow and I'm sure that posting your question there can get you an answer in the matter of minutes.
$endgroup$
– I_Play_With_Data
2 days ago
$begingroup$
Why do you do if then pass them else? You could negate the if and drop the pass/else
$endgroup$
– kbrose
15 hours ago
$begingroup$
@kbrose I have updated the code in question based on your suggestion. But still it is very slow. Can it be further improvised?
$endgroup$
– shaifali Gupta
14 hours ago
$begingroup$
i am not an expert, but pandas couldnt work in this case? They have highly optimized performance for some tasks.
$endgroup$
– Victor Oliveira
14 hours ago
$begingroup$
Have you tried making it a set instead of a list? Sets are optimized for containment checking.
$endgroup$
– kbrose
14 hours ago
$begingroup$
Welcome to the site! You are certainly welcome to leave your question here, but there are some serious python wizards on Stack Overflow and I'm sure that posting your question there can get you an answer in the matter of minutes.
$endgroup$
– I_Play_With_Data
2 days ago
$begingroup$
Welcome to the site! You are certainly welcome to leave your question here, but there are some serious python wizards on Stack Overflow and I'm sure that posting your question there can get you an answer in the matter of minutes.
$endgroup$
– I_Play_With_Data
2 days ago
$begingroup$
Why do you do if then pass them else? You could negate the if and drop the pass/else
$endgroup$
– kbrose
15 hours ago
$begingroup$
Why do you do if then pass them else? You could negate the if and drop the pass/else
$endgroup$
– kbrose
15 hours ago
$begingroup$
@kbrose I have updated the code in question based on your suggestion. But still it is very slow. Can it be further improvised?
$endgroup$
– shaifali Gupta
14 hours ago
$begingroup$
@kbrose I have updated the code in question based on your suggestion. But still it is very slow. Can it be further improvised?
$endgroup$
– shaifali Gupta
14 hours ago
$begingroup$
i am not an expert, but pandas couldnt work in this case? They have highly optimized performance for some tasks.
$endgroup$
– Victor Oliveira
14 hours ago
$begingroup$
i am not an expert, but pandas couldnt work in this case? They have highly optimized performance for some tasks.
$endgroup$
– Victor Oliveira
14 hours ago
$begingroup$
Have you tried making it a set instead of a list? Sets are optimized for containment checking.
$endgroup$
– kbrose
14 hours ago
$begingroup$
Have you tried making it a set instead of a list? Sets are optimized for containment checking.
$endgroup$
– kbrose
14 hours ago
|
show 3 more comments
1 Answer
1
active
oldest
votes
$begingroup$
Assuming I have understood your question... I might alter my answer if OP updates the question with more details
Using your example data, you can use Pandas easily drop all duplicates.
Setup
First dump your data above into a dataframe with three columns (wone for each of the items in each row:
import pandas:
import pandas as pd
import your data - assuming it is a list of lists (each of your rows a is a list of three items!):
df = pd.DataFrame.from_records(your_list_of_lists, columns=["col1", "col2", "col3"])
Have a look at the first 5 rows:
df.head()
col1 col2 col3
0 600 900 3418309
1 600 900 3418309
2 600 900 3418314
3 600 900 3418314
4 600 900 3418319
The values will be integers by default, not strings (if they all were).
Solutions
If you want to get all unique values of col3
, you can do one of the following:
uniques1 = set(df.col3) # returns a Python set
uniques2 = df.col3.unique() # returns a NumPy ndarray
uniques3 = df.col3.drop_duplicates() # returns a pandas Series object
Performance
There are many other ways to achieve the same result. Of the above, the first method is the fastest (on your small dataset example). Here are the benchmarks:
In [23]: %timeit df.col3.drop_duplicates()
%263 µs ± 883 ns per loop (mean ± std. dev. of 7 runs, 1000 loops each)
In [24]: %timeit df.col3.unique()
%37.2 µs ± 3.19 µs per loop (mean ± std. dev. of 7 runs, 10000 loops each)
In [25]: %timeit set(df.col3)
10.5 µs ± 45.7 ns per loop (mean ± std. dev. of 7 runs, 100000 loops each)
$endgroup$
add a comment |
Your Answer
StackExchange.ifUsing("editor", function () {
return StackExchange.using("mathjaxEditing", function () {
StackExchange.MarkdownEditor.creationCallbacks.add(function (editor, postfix) {
StackExchange.mathjaxEditing.prepareWmdForMathJax(editor, postfix, [["$", "$"], ["\\(","\\)"]]);
});
});
}, "mathjax-editing");
StackExchange.ready(function() {
var channelOptions = {
tags: "".split(" "),
id: "557"
};
initTagRenderer("".split(" "), "".split(" "), channelOptions);
StackExchange.using("externalEditor", function() {
// Have to fire editor after snippets, if snippets enabled
if (StackExchange.settings.snippets.snippetsEnabled) {
StackExchange.using("snippets", function() {
createEditor();
});
}
else {
createEditor();
}
});
function createEditor() {
StackExchange.prepareEditor({
heartbeatType: 'answer',
autoActivateHeartbeat: false,
convertImagesToLinks: false,
noModals: true,
showLowRepImageUploadWarning: true,
reputationToPostImages: null,
bindNavPrevention: true,
postfix: "",
imageUploader: {
brandingHtml: "Powered by u003ca class="icon-imgur-white" href="https://imgur.com/"u003eu003c/au003e",
contentPolicyHtml: "User contributions licensed under u003ca href="https://creativecommons.org/licenses/by-sa/3.0/"u003ecc by-sa 3.0 with attribution requiredu003c/au003e u003ca href="https://stackoverflow.com/legal/content-policy"u003e(content policy)u003c/au003e",
allowUrls: true
},
onDemand: true,
discardSelector: ".discard-answer"
,immediatelyShowMarkdownHelp:true
});
}
});
Sign up or log in
StackExchange.ready(function () {
StackExchange.helpers.onClickDraftSave('#login-link');
});
Sign up using Google
Sign up using Facebook
Sign up using Email and Password
Post as a guest
Required, but never shown
StackExchange.ready(
function () {
StackExchange.openid.initPostLogin('.new-post-login', 'https%3a%2f%2fdatascience.stackexchange.com%2fquestions%2f46882%2fpython-fast-indexing-of-strings-in-nested-list-without-loop%23new-answer', 'question_page');
}
);
Post as a guest
Required, but never shown
1 Answer
1
active
oldest
votes
1 Answer
1
active
oldest
votes
active
oldest
votes
active
oldest
votes
$begingroup$
Assuming I have understood your question... I might alter my answer if OP updates the question with more details
Using your example data, you can use Pandas easily drop all duplicates.
Setup
First dump your data above into a dataframe with three columns (wone for each of the items in each row:
import pandas:
import pandas as pd
import your data - assuming it is a list of lists (each of your rows a is a list of three items!):
df = pd.DataFrame.from_records(your_list_of_lists, columns=["col1", "col2", "col3"])
Have a look at the first 5 rows:
df.head()
col1 col2 col3
0 600 900 3418309
1 600 900 3418309
2 600 900 3418314
3 600 900 3418314
4 600 900 3418319
The values will be integers by default, not strings (if they all were).
Solutions
If you want to get all unique values of col3
, you can do one of the following:
uniques1 = set(df.col3) # returns a Python set
uniques2 = df.col3.unique() # returns a NumPy ndarray
uniques3 = df.col3.drop_duplicates() # returns a pandas Series object
Performance
There are many other ways to achieve the same result. Of the above, the first method is the fastest (on your small dataset example). Here are the benchmarks:
In [23]: %timeit df.col3.drop_duplicates()
%263 µs ± 883 ns per loop (mean ± std. dev. of 7 runs, 1000 loops each)
In [24]: %timeit df.col3.unique()
%37.2 µs ± 3.19 µs per loop (mean ± std. dev. of 7 runs, 10000 loops each)
In [25]: %timeit set(df.col3)
10.5 µs ± 45.7 ns per loop (mean ± std. dev. of 7 runs, 100000 loops each)
$endgroup$
add a comment |
$begingroup$
Assuming I have understood your question... I might alter my answer if OP updates the question with more details
Using your example data, you can use Pandas easily drop all duplicates.
Setup
First dump your data above into a dataframe with three columns (wone for each of the items in each row:
import pandas:
import pandas as pd
import your data - assuming it is a list of lists (each of your rows a is a list of three items!):
df = pd.DataFrame.from_records(your_list_of_lists, columns=["col1", "col2", "col3"])
Have a look at the first 5 rows:
df.head()
col1 col2 col3
0 600 900 3418309
1 600 900 3418309
2 600 900 3418314
3 600 900 3418314
4 600 900 3418319
The values will be integers by default, not strings (if they all were).
Solutions
If you want to get all unique values of col3
, you can do one of the following:
uniques1 = set(df.col3) # returns a Python set
uniques2 = df.col3.unique() # returns a NumPy ndarray
uniques3 = df.col3.drop_duplicates() # returns a pandas Series object
Performance
There are many other ways to achieve the same result. Of the above, the first method is the fastest (on your small dataset example). Here are the benchmarks:
In [23]: %timeit df.col3.drop_duplicates()
%263 µs ± 883 ns per loop (mean ± std. dev. of 7 runs, 1000 loops each)
In [24]: %timeit df.col3.unique()
%37.2 µs ± 3.19 µs per loop (mean ± std. dev. of 7 runs, 10000 loops each)
In [25]: %timeit set(df.col3)
10.5 µs ± 45.7 ns per loop (mean ± std. dev. of 7 runs, 100000 loops each)
$endgroup$
add a comment |
$begingroup$
Assuming I have understood your question... I might alter my answer if OP updates the question with more details
Using your example data, you can use Pandas easily drop all duplicates.
Setup
First dump your data above into a dataframe with three columns (wone for each of the items in each row:
import pandas:
import pandas as pd
import your data - assuming it is a list of lists (each of your rows a is a list of three items!):
df = pd.DataFrame.from_records(your_list_of_lists, columns=["col1", "col2", "col3"])
Have a look at the first 5 rows:
df.head()
col1 col2 col3
0 600 900 3418309
1 600 900 3418309
2 600 900 3418314
3 600 900 3418314
4 600 900 3418319
The values will be integers by default, not strings (if they all were).
Solutions
If you want to get all unique values of col3
, you can do one of the following:
uniques1 = set(df.col3) # returns a Python set
uniques2 = df.col3.unique() # returns a NumPy ndarray
uniques3 = df.col3.drop_duplicates() # returns a pandas Series object
Performance
There are many other ways to achieve the same result. Of the above, the first method is the fastest (on your small dataset example). Here are the benchmarks:
In [23]: %timeit df.col3.drop_duplicates()
%263 µs ± 883 ns per loop (mean ± std. dev. of 7 runs, 1000 loops each)
In [24]: %timeit df.col3.unique()
%37.2 µs ± 3.19 µs per loop (mean ± std. dev. of 7 runs, 10000 loops each)
In [25]: %timeit set(df.col3)
10.5 µs ± 45.7 ns per loop (mean ± std. dev. of 7 runs, 100000 loops each)
$endgroup$
Assuming I have understood your question... I might alter my answer if OP updates the question with more details
Using your example data, you can use Pandas easily drop all duplicates.
Setup
First dump your data above into a dataframe with three columns (wone for each of the items in each row:
import pandas:
import pandas as pd
import your data - assuming it is a list of lists (each of your rows a is a list of three items!):
df = pd.DataFrame.from_records(your_list_of_lists, columns=["col1", "col2", "col3"])
Have a look at the first 5 rows:
df.head()
col1 col2 col3
0 600 900 3418309
1 600 900 3418309
2 600 900 3418314
3 600 900 3418314
4 600 900 3418319
The values will be integers by default, not strings (if they all were).
Solutions
If you want to get all unique values of col3
, you can do one of the following:
uniques1 = set(df.col3) # returns a Python set
uniques2 = df.col3.unique() # returns a NumPy ndarray
uniques3 = df.col3.drop_duplicates() # returns a pandas Series object
Performance
There are many other ways to achieve the same result. Of the above, the first method is the fastest (on your small dataset example). Here are the benchmarks:
In [23]: %timeit df.col3.drop_duplicates()
%263 µs ± 883 ns per loop (mean ± std. dev. of 7 runs, 1000 loops each)
In [24]: %timeit df.col3.unique()
%37.2 µs ± 3.19 µs per loop (mean ± std. dev. of 7 runs, 10000 loops each)
In [25]: %timeit set(df.col3)
10.5 µs ± 45.7 ns per loop (mean ± std. dev. of 7 runs, 100000 loops each)
answered 10 hours ago


n1k31t4n1k31t4
6,3262319
6,3262319
add a comment |
add a comment |
Thanks for contributing an answer to Data Science Stack Exchange!
- Please be sure to answer the question. Provide details and share your research!
But avoid …
- Asking for help, clarification, or responding to other answers.
- Making statements based on opinion; back them up with references or personal experience.
Use MathJax to format equations. MathJax reference.
To learn more, see our tips on writing great answers.
Sign up or log in
StackExchange.ready(function () {
StackExchange.helpers.onClickDraftSave('#login-link');
});
Sign up using Google
Sign up using Facebook
Sign up using Email and Password
Post as a guest
Required, but never shown
StackExchange.ready(
function () {
StackExchange.openid.initPostLogin('.new-post-login', 'https%3a%2f%2fdatascience.stackexchange.com%2fquestions%2f46882%2fpython-fast-indexing-of-strings-in-nested-list-without-loop%23new-answer', 'question_page');
}
);
Post as a guest
Required, but never shown
Sign up or log in
StackExchange.ready(function () {
StackExchange.helpers.onClickDraftSave('#login-link');
});
Sign up using Google
Sign up using Facebook
Sign up using Email and Password
Post as a guest
Required, but never shown
Sign up or log in
StackExchange.ready(function () {
StackExchange.helpers.onClickDraftSave('#login-link');
});
Sign up using Google
Sign up using Facebook
Sign up using Email and Password
Post as a guest
Required, but never shown
Sign up or log in
StackExchange.ready(function () {
StackExchange.helpers.onClickDraftSave('#login-link');
});
Sign up using Google
Sign up using Facebook
Sign up using Email and Password
Sign up using Google
Sign up using Facebook
Sign up using Email and Password
Post as a guest
Required, but never shown
Required, but never shown
Required, but never shown
Required, but never shown
Required, but never shown
Required, but never shown
Required, but never shown
Required, but never shown
Required, but never shown
RFKunDZGVZn4v0AQj7Y,PRzSGY0ZGwsKC,o5 cUNlR0aWc djYikTacLJ
$begingroup$
Welcome to the site! You are certainly welcome to leave your question here, but there are some serious python wizards on Stack Overflow and I'm sure that posting your question there can get you an answer in the matter of minutes.
$endgroup$
– I_Play_With_Data
2 days ago
$begingroup$
Why do you do if then pass them else? You could negate the if and drop the pass/else
$endgroup$
– kbrose
15 hours ago
$begingroup$
@kbrose I have updated the code in question based on your suggestion. But still it is very slow. Can it be further improvised?
$endgroup$
– shaifali Gupta
14 hours ago
$begingroup$
i am not an expert, but pandas couldnt work in this case? They have highly optimized performance for some tasks.
$endgroup$
– Victor Oliveira
14 hours ago
$begingroup$
Have you tried making it a set instead of a list? Sets are optimized for containment checking.
$endgroup$
– kbrose
14 hours ago