Keras Applications - using images larger than the default size
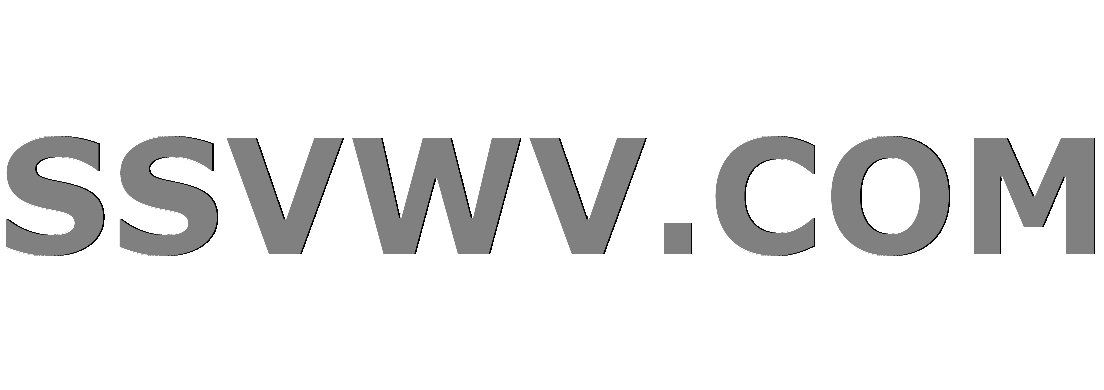
Multi tool use
$begingroup$
I would like to use eg Xception network with default input size 299x299, but my images are 450x600. Are there any other options besides cropping and subsampling ?
keras tensorflow cnn
$endgroup$
add a comment |
$begingroup$
I would like to use eg Xception network with default input size 299x299, but my images are 450x600. Are there any other options besides cropping and subsampling ?
keras tensorflow cnn
$endgroup$
add a comment |
$begingroup$
I would like to use eg Xception network with default input size 299x299, but my images are 450x600. Are there any other options besides cropping and subsampling ?
keras tensorflow cnn
$endgroup$
I would like to use eg Xception network with default input size 299x299, but my images are 450x600. Are there any other options besides cropping and subsampling ?
keras tensorflow cnn
keras tensorflow cnn
asked 13 hours ago
I.D.MI.D.M
135
135
add a comment |
add a comment |
1 Answer
1
active
oldest
votes
$begingroup$
Have a look at where the reshaping happens. Just before that, you can insert a global average pooling layer in. This way you can handle any size.
However, I recommend cropping and scamming. Create multiple crops if necessary and average the results. That is likely still faster than using a bigger image.
How to use Xception
#!/usr/bin/env python
# -*- coding: utf-8 -*-
"""See https://martin-thoma.com/image-classification/ for details."""
from __future__ import print_function
import numpy as np
import json
import os
import time
from keras import backend as K
from keras.preprocessing import image
from keras.applications.xception import Xception
from keras.utils.data_utils import get_file
CLASS_INDEX = None
CLASS_INDEX_PATH = ('https://s3.amazonaws.com/deep-learning-models/'
'image-models/imagenet_class_index.json')
def preprocess_input(x, dim_ordering='default'):
"""
Standard preprocessing of image data.
1. Make sure the order of the channels is correct (RGB, BGR, depending on
the backend)
2. Mean subtraction by channel.
Parameters
----------
x : numpy array
The image
dim_ordering : string, optional (default: 'default')
Either 'th' for Theano or 'tf' for Tensorflow
Returns
-------
numpy array
The preprocessed image
"""
if dim_ordering == 'default':
dim_ordering = K.image_dim_ordering()
assert dim_ordering in {'tf', 'th'}
if dim_ordering == 'th':
x[:, 0, :, :] -= 103.939
x[:, 1, :, :] -= 116.779
x[:, 2, :, :] -= 123.68
# 'RGB'->'BGR'
x = x[:, ::-1, :, :]
else:
x[:, :, :, 0] -= 103.939
x[:, :, :, 1] -= 116.779
x[:, :, :, 2] -= 123.68
# 'RGB'->'BGR'
x = x[:, :, :, ::-1]
return x
def decode_predictions(preds, top=5):
"""
Decode the predictionso of the ImageNet trained network.
Parameters
----------
preds : numpy array
top : int
How many predictions to return
Returns
-------
list of tuples
e.g. (u'n02206856', u'bee', 0.71072823) for the WordNet identifier,
the class name and the probability.
"""
global CLASS_INDEX
if len(preds.shape) != 2 or preds.shape[1] != 1000:
raise ValueError('`decode_predictions` expects '
'a batch of predictions '
'(i.e. a 2D array of shape (samples, 1000)). '
'Found array with shape: ' + str(preds.shape))
if CLASS_INDEX is None:
fpath = get_file('imagenet_class_index.json',
CLASS_INDEX_PATH,
cache_subdir='models')
CLASS_INDEX = json.load(open(fpath))
results =
for pred in preds:
top_indices = pred.argsort()[-top:][::-1]
result = [tuple(CLASS_INDEX[str(i)]) + (pred[i],) for i in top_indices]
results.append(result)
return results
def is_valid_file(parser, arg):
"""
Check if arg is a valid file that already exists on the file system.
Parameters
----------
parser : argparse object
arg : str
Returns
-------
arg
"""
arg = os.path.abspath(arg)
if not os.path.exists(arg):
parser.error("The file %s does not exist!" % arg)
else:
return arg
def get_parser():
"""Get parser object."""
from argparse import ArgumentParser, ArgumentDefaultsHelpFormatter
parser = ArgumentParser(description=__doc__,
formatter_class=ArgumentDefaultsHelpFormatter)
parser.add_argument("-f", "--file",
dest="filename",
type=lambda x: is_valid_file(parser, x),
help="Classify image",
metavar="IMAGE",
required=True)
return parser
if __name__ == "__main__":
args = get_parser().parse_args()
# Load model
model = Xception(include_top=True, weights='imagenet')
img_path = args.filename
img = image.load_img(img_path, target_size=(224, 224))
x = image.img_to_array(img)
x = np.expand_dims(x, axis=0)
x = preprocess_input(x)
print('Input image shape:', x.shape)
t0 = time.time()
preds = model.predict(x)
t1 = time.time()
print("Prediction time: {:0.3f}s".format(t1 - t0))
for wordnet_id, class_name, prob in decode_predictions(preds)[0]:
print("{wid}t{prob:>6}%t{name}".format(wid=wordnet_id,
name=class_name,
prob="%0.2f" % (prob * 100)))
Why it works with any size
Look at the model.summary()
of Xception, especially the output shape. Notice the global average pooling layer? Before that, the shape is determined by the input. Meaning until that point, it can be anything.
Global pooling is another type of transition layer. It applies pooling over the complete feature map size to shrink the input to a constant 1 × 1 feature map and hence allows one network to have different input sizes.
Source: Me: Analysis and Optimization of Convolutional Neural Network Architectures
$endgroup$
$begingroup$
Reshaping of what exactly ? Input tensor ? In that case, would not we receive something like that for RGB images: [450, 600, 3] -> [1, 1, 3] ?
$endgroup$
– I.D.M
11 hours ago
$begingroup$
I've edited the example quite a bit. Turns out that you actually don't need to change anything.
$endgroup$
– Martin Thoma
10 hours ago
$begingroup$
Thank you very much for your extended response. I have a few more questions: 1. Why the documentation says that the default input size is 299x299 ? 2. It seems to me that there is some broken formatting at output shape - eg before GlobalAveragePooling2 it should probably be (None, None, None, 2048) instead (None, None, None, 2 3. Should I change only the Dense layer ?
$endgroup$
– I.D.M
9 hours ago
add a comment |
Your Answer
StackExchange.ifUsing("editor", function () {
return StackExchange.using("mathjaxEditing", function () {
StackExchange.MarkdownEditor.creationCallbacks.add(function (editor, postfix) {
StackExchange.mathjaxEditing.prepareWmdForMathJax(editor, postfix, [["$", "$"], ["\\(","\\)"]]);
});
});
}, "mathjax-editing");
StackExchange.ready(function() {
var channelOptions = {
tags: "".split(" "),
id: "557"
};
initTagRenderer("".split(" "), "".split(" "), channelOptions);
StackExchange.using("externalEditor", function() {
// Have to fire editor after snippets, if snippets enabled
if (StackExchange.settings.snippets.snippetsEnabled) {
StackExchange.using("snippets", function() {
createEditor();
});
}
else {
createEditor();
}
});
function createEditor() {
StackExchange.prepareEditor({
heartbeatType: 'answer',
autoActivateHeartbeat: false,
convertImagesToLinks: false,
noModals: true,
showLowRepImageUploadWarning: true,
reputationToPostImages: null,
bindNavPrevention: true,
postfix: "",
imageUploader: {
brandingHtml: "Powered by u003ca class="icon-imgur-white" href="https://imgur.com/"u003eu003c/au003e",
contentPolicyHtml: "User contributions licensed under u003ca href="https://creativecommons.org/licenses/by-sa/3.0/"u003ecc by-sa 3.0 with attribution requiredu003c/au003e u003ca href="https://stackoverflow.com/legal/content-policy"u003e(content policy)u003c/au003e",
allowUrls: true
},
onDemand: true,
discardSelector: ".discard-answer"
,immediatelyShowMarkdownHelp:true
});
}
});
Sign up or log in
StackExchange.ready(function () {
StackExchange.helpers.onClickDraftSave('#login-link');
});
Sign up using Google
Sign up using Facebook
Sign up using Email and Password
Post as a guest
Required, but never shown
StackExchange.ready(
function () {
StackExchange.openid.initPostLogin('.new-post-login', 'https%3a%2f%2fdatascience.stackexchange.com%2fquestions%2f45393%2fkeras-applications-using-images-larger-than-the-default-size%23new-answer', 'question_page');
}
);
Post as a guest
Required, but never shown
1 Answer
1
active
oldest
votes
1 Answer
1
active
oldest
votes
active
oldest
votes
active
oldest
votes
$begingroup$
Have a look at where the reshaping happens. Just before that, you can insert a global average pooling layer in. This way you can handle any size.
However, I recommend cropping and scamming. Create multiple crops if necessary and average the results. That is likely still faster than using a bigger image.
How to use Xception
#!/usr/bin/env python
# -*- coding: utf-8 -*-
"""See https://martin-thoma.com/image-classification/ for details."""
from __future__ import print_function
import numpy as np
import json
import os
import time
from keras import backend as K
from keras.preprocessing import image
from keras.applications.xception import Xception
from keras.utils.data_utils import get_file
CLASS_INDEX = None
CLASS_INDEX_PATH = ('https://s3.amazonaws.com/deep-learning-models/'
'image-models/imagenet_class_index.json')
def preprocess_input(x, dim_ordering='default'):
"""
Standard preprocessing of image data.
1. Make sure the order of the channels is correct (RGB, BGR, depending on
the backend)
2. Mean subtraction by channel.
Parameters
----------
x : numpy array
The image
dim_ordering : string, optional (default: 'default')
Either 'th' for Theano or 'tf' for Tensorflow
Returns
-------
numpy array
The preprocessed image
"""
if dim_ordering == 'default':
dim_ordering = K.image_dim_ordering()
assert dim_ordering in {'tf', 'th'}
if dim_ordering == 'th':
x[:, 0, :, :] -= 103.939
x[:, 1, :, :] -= 116.779
x[:, 2, :, :] -= 123.68
# 'RGB'->'BGR'
x = x[:, ::-1, :, :]
else:
x[:, :, :, 0] -= 103.939
x[:, :, :, 1] -= 116.779
x[:, :, :, 2] -= 123.68
# 'RGB'->'BGR'
x = x[:, :, :, ::-1]
return x
def decode_predictions(preds, top=5):
"""
Decode the predictionso of the ImageNet trained network.
Parameters
----------
preds : numpy array
top : int
How many predictions to return
Returns
-------
list of tuples
e.g. (u'n02206856', u'bee', 0.71072823) for the WordNet identifier,
the class name and the probability.
"""
global CLASS_INDEX
if len(preds.shape) != 2 or preds.shape[1] != 1000:
raise ValueError('`decode_predictions` expects '
'a batch of predictions '
'(i.e. a 2D array of shape (samples, 1000)). '
'Found array with shape: ' + str(preds.shape))
if CLASS_INDEX is None:
fpath = get_file('imagenet_class_index.json',
CLASS_INDEX_PATH,
cache_subdir='models')
CLASS_INDEX = json.load(open(fpath))
results =
for pred in preds:
top_indices = pred.argsort()[-top:][::-1]
result = [tuple(CLASS_INDEX[str(i)]) + (pred[i],) for i in top_indices]
results.append(result)
return results
def is_valid_file(parser, arg):
"""
Check if arg is a valid file that already exists on the file system.
Parameters
----------
parser : argparse object
arg : str
Returns
-------
arg
"""
arg = os.path.abspath(arg)
if not os.path.exists(arg):
parser.error("The file %s does not exist!" % arg)
else:
return arg
def get_parser():
"""Get parser object."""
from argparse import ArgumentParser, ArgumentDefaultsHelpFormatter
parser = ArgumentParser(description=__doc__,
formatter_class=ArgumentDefaultsHelpFormatter)
parser.add_argument("-f", "--file",
dest="filename",
type=lambda x: is_valid_file(parser, x),
help="Classify image",
metavar="IMAGE",
required=True)
return parser
if __name__ == "__main__":
args = get_parser().parse_args()
# Load model
model = Xception(include_top=True, weights='imagenet')
img_path = args.filename
img = image.load_img(img_path, target_size=(224, 224))
x = image.img_to_array(img)
x = np.expand_dims(x, axis=0)
x = preprocess_input(x)
print('Input image shape:', x.shape)
t0 = time.time()
preds = model.predict(x)
t1 = time.time()
print("Prediction time: {:0.3f}s".format(t1 - t0))
for wordnet_id, class_name, prob in decode_predictions(preds)[0]:
print("{wid}t{prob:>6}%t{name}".format(wid=wordnet_id,
name=class_name,
prob="%0.2f" % (prob * 100)))
Why it works with any size
Look at the model.summary()
of Xception, especially the output shape. Notice the global average pooling layer? Before that, the shape is determined by the input. Meaning until that point, it can be anything.
Global pooling is another type of transition layer. It applies pooling over the complete feature map size to shrink the input to a constant 1 × 1 feature map and hence allows one network to have different input sizes.
Source: Me: Analysis and Optimization of Convolutional Neural Network Architectures
$endgroup$
$begingroup$
Reshaping of what exactly ? Input tensor ? In that case, would not we receive something like that for RGB images: [450, 600, 3] -> [1, 1, 3] ?
$endgroup$
– I.D.M
11 hours ago
$begingroup$
I've edited the example quite a bit. Turns out that you actually don't need to change anything.
$endgroup$
– Martin Thoma
10 hours ago
$begingroup$
Thank you very much for your extended response. I have a few more questions: 1. Why the documentation says that the default input size is 299x299 ? 2. It seems to me that there is some broken formatting at output shape - eg before GlobalAveragePooling2 it should probably be (None, None, None, 2048) instead (None, None, None, 2 3. Should I change only the Dense layer ?
$endgroup$
– I.D.M
9 hours ago
add a comment |
$begingroup$
Have a look at where the reshaping happens. Just before that, you can insert a global average pooling layer in. This way you can handle any size.
However, I recommend cropping and scamming. Create multiple crops if necessary and average the results. That is likely still faster than using a bigger image.
How to use Xception
#!/usr/bin/env python
# -*- coding: utf-8 -*-
"""See https://martin-thoma.com/image-classification/ for details."""
from __future__ import print_function
import numpy as np
import json
import os
import time
from keras import backend as K
from keras.preprocessing import image
from keras.applications.xception import Xception
from keras.utils.data_utils import get_file
CLASS_INDEX = None
CLASS_INDEX_PATH = ('https://s3.amazonaws.com/deep-learning-models/'
'image-models/imagenet_class_index.json')
def preprocess_input(x, dim_ordering='default'):
"""
Standard preprocessing of image data.
1. Make sure the order of the channels is correct (RGB, BGR, depending on
the backend)
2. Mean subtraction by channel.
Parameters
----------
x : numpy array
The image
dim_ordering : string, optional (default: 'default')
Either 'th' for Theano or 'tf' for Tensorflow
Returns
-------
numpy array
The preprocessed image
"""
if dim_ordering == 'default':
dim_ordering = K.image_dim_ordering()
assert dim_ordering in {'tf', 'th'}
if dim_ordering == 'th':
x[:, 0, :, :] -= 103.939
x[:, 1, :, :] -= 116.779
x[:, 2, :, :] -= 123.68
# 'RGB'->'BGR'
x = x[:, ::-1, :, :]
else:
x[:, :, :, 0] -= 103.939
x[:, :, :, 1] -= 116.779
x[:, :, :, 2] -= 123.68
# 'RGB'->'BGR'
x = x[:, :, :, ::-1]
return x
def decode_predictions(preds, top=5):
"""
Decode the predictionso of the ImageNet trained network.
Parameters
----------
preds : numpy array
top : int
How many predictions to return
Returns
-------
list of tuples
e.g. (u'n02206856', u'bee', 0.71072823) for the WordNet identifier,
the class name and the probability.
"""
global CLASS_INDEX
if len(preds.shape) != 2 or preds.shape[1] != 1000:
raise ValueError('`decode_predictions` expects '
'a batch of predictions '
'(i.e. a 2D array of shape (samples, 1000)). '
'Found array with shape: ' + str(preds.shape))
if CLASS_INDEX is None:
fpath = get_file('imagenet_class_index.json',
CLASS_INDEX_PATH,
cache_subdir='models')
CLASS_INDEX = json.load(open(fpath))
results =
for pred in preds:
top_indices = pred.argsort()[-top:][::-1]
result = [tuple(CLASS_INDEX[str(i)]) + (pred[i],) for i in top_indices]
results.append(result)
return results
def is_valid_file(parser, arg):
"""
Check if arg is a valid file that already exists on the file system.
Parameters
----------
parser : argparse object
arg : str
Returns
-------
arg
"""
arg = os.path.abspath(arg)
if not os.path.exists(arg):
parser.error("The file %s does not exist!" % arg)
else:
return arg
def get_parser():
"""Get parser object."""
from argparse import ArgumentParser, ArgumentDefaultsHelpFormatter
parser = ArgumentParser(description=__doc__,
formatter_class=ArgumentDefaultsHelpFormatter)
parser.add_argument("-f", "--file",
dest="filename",
type=lambda x: is_valid_file(parser, x),
help="Classify image",
metavar="IMAGE",
required=True)
return parser
if __name__ == "__main__":
args = get_parser().parse_args()
# Load model
model = Xception(include_top=True, weights='imagenet')
img_path = args.filename
img = image.load_img(img_path, target_size=(224, 224))
x = image.img_to_array(img)
x = np.expand_dims(x, axis=0)
x = preprocess_input(x)
print('Input image shape:', x.shape)
t0 = time.time()
preds = model.predict(x)
t1 = time.time()
print("Prediction time: {:0.3f}s".format(t1 - t0))
for wordnet_id, class_name, prob in decode_predictions(preds)[0]:
print("{wid}t{prob:>6}%t{name}".format(wid=wordnet_id,
name=class_name,
prob="%0.2f" % (prob * 100)))
Why it works with any size
Look at the model.summary()
of Xception, especially the output shape. Notice the global average pooling layer? Before that, the shape is determined by the input. Meaning until that point, it can be anything.
Global pooling is another type of transition layer. It applies pooling over the complete feature map size to shrink the input to a constant 1 × 1 feature map and hence allows one network to have different input sizes.
Source: Me: Analysis and Optimization of Convolutional Neural Network Architectures
$endgroup$
$begingroup$
Reshaping of what exactly ? Input tensor ? In that case, would not we receive something like that for RGB images: [450, 600, 3] -> [1, 1, 3] ?
$endgroup$
– I.D.M
11 hours ago
$begingroup$
I've edited the example quite a bit. Turns out that you actually don't need to change anything.
$endgroup$
– Martin Thoma
10 hours ago
$begingroup$
Thank you very much for your extended response. I have a few more questions: 1. Why the documentation says that the default input size is 299x299 ? 2. It seems to me that there is some broken formatting at output shape - eg before GlobalAveragePooling2 it should probably be (None, None, None, 2048) instead (None, None, None, 2 3. Should I change only the Dense layer ?
$endgroup$
– I.D.M
9 hours ago
add a comment |
$begingroup$
Have a look at where the reshaping happens. Just before that, you can insert a global average pooling layer in. This way you can handle any size.
However, I recommend cropping and scamming. Create multiple crops if necessary and average the results. That is likely still faster than using a bigger image.
How to use Xception
#!/usr/bin/env python
# -*- coding: utf-8 -*-
"""See https://martin-thoma.com/image-classification/ for details."""
from __future__ import print_function
import numpy as np
import json
import os
import time
from keras import backend as K
from keras.preprocessing import image
from keras.applications.xception import Xception
from keras.utils.data_utils import get_file
CLASS_INDEX = None
CLASS_INDEX_PATH = ('https://s3.amazonaws.com/deep-learning-models/'
'image-models/imagenet_class_index.json')
def preprocess_input(x, dim_ordering='default'):
"""
Standard preprocessing of image data.
1. Make sure the order of the channels is correct (RGB, BGR, depending on
the backend)
2. Mean subtraction by channel.
Parameters
----------
x : numpy array
The image
dim_ordering : string, optional (default: 'default')
Either 'th' for Theano or 'tf' for Tensorflow
Returns
-------
numpy array
The preprocessed image
"""
if dim_ordering == 'default':
dim_ordering = K.image_dim_ordering()
assert dim_ordering in {'tf', 'th'}
if dim_ordering == 'th':
x[:, 0, :, :] -= 103.939
x[:, 1, :, :] -= 116.779
x[:, 2, :, :] -= 123.68
# 'RGB'->'BGR'
x = x[:, ::-1, :, :]
else:
x[:, :, :, 0] -= 103.939
x[:, :, :, 1] -= 116.779
x[:, :, :, 2] -= 123.68
# 'RGB'->'BGR'
x = x[:, :, :, ::-1]
return x
def decode_predictions(preds, top=5):
"""
Decode the predictionso of the ImageNet trained network.
Parameters
----------
preds : numpy array
top : int
How many predictions to return
Returns
-------
list of tuples
e.g. (u'n02206856', u'bee', 0.71072823) for the WordNet identifier,
the class name and the probability.
"""
global CLASS_INDEX
if len(preds.shape) != 2 or preds.shape[1] != 1000:
raise ValueError('`decode_predictions` expects '
'a batch of predictions '
'(i.e. a 2D array of shape (samples, 1000)). '
'Found array with shape: ' + str(preds.shape))
if CLASS_INDEX is None:
fpath = get_file('imagenet_class_index.json',
CLASS_INDEX_PATH,
cache_subdir='models')
CLASS_INDEX = json.load(open(fpath))
results =
for pred in preds:
top_indices = pred.argsort()[-top:][::-1]
result = [tuple(CLASS_INDEX[str(i)]) + (pred[i],) for i in top_indices]
results.append(result)
return results
def is_valid_file(parser, arg):
"""
Check if arg is a valid file that already exists on the file system.
Parameters
----------
parser : argparse object
arg : str
Returns
-------
arg
"""
arg = os.path.abspath(arg)
if not os.path.exists(arg):
parser.error("The file %s does not exist!" % arg)
else:
return arg
def get_parser():
"""Get parser object."""
from argparse import ArgumentParser, ArgumentDefaultsHelpFormatter
parser = ArgumentParser(description=__doc__,
formatter_class=ArgumentDefaultsHelpFormatter)
parser.add_argument("-f", "--file",
dest="filename",
type=lambda x: is_valid_file(parser, x),
help="Classify image",
metavar="IMAGE",
required=True)
return parser
if __name__ == "__main__":
args = get_parser().parse_args()
# Load model
model = Xception(include_top=True, weights='imagenet')
img_path = args.filename
img = image.load_img(img_path, target_size=(224, 224))
x = image.img_to_array(img)
x = np.expand_dims(x, axis=0)
x = preprocess_input(x)
print('Input image shape:', x.shape)
t0 = time.time()
preds = model.predict(x)
t1 = time.time()
print("Prediction time: {:0.3f}s".format(t1 - t0))
for wordnet_id, class_name, prob in decode_predictions(preds)[0]:
print("{wid}t{prob:>6}%t{name}".format(wid=wordnet_id,
name=class_name,
prob="%0.2f" % (prob * 100)))
Why it works with any size
Look at the model.summary()
of Xception, especially the output shape. Notice the global average pooling layer? Before that, the shape is determined by the input. Meaning until that point, it can be anything.
Global pooling is another type of transition layer. It applies pooling over the complete feature map size to shrink the input to a constant 1 × 1 feature map and hence allows one network to have different input sizes.
Source: Me: Analysis and Optimization of Convolutional Neural Network Architectures
$endgroup$
Have a look at where the reshaping happens. Just before that, you can insert a global average pooling layer in. This way you can handle any size.
However, I recommend cropping and scamming. Create multiple crops if necessary and average the results. That is likely still faster than using a bigger image.
How to use Xception
#!/usr/bin/env python
# -*- coding: utf-8 -*-
"""See https://martin-thoma.com/image-classification/ for details."""
from __future__ import print_function
import numpy as np
import json
import os
import time
from keras import backend as K
from keras.preprocessing import image
from keras.applications.xception import Xception
from keras.utils.data_utils import get_file
CLASS_INDEX = None
CLASS_INDEX_PATH = ('https://s3.amazonaws.com/deep-learning-models/'
'image-models/imagenet_class_index.json')
def preprocess_input(x, dim_ordering='default'):
"""
Standard preprocessing of image data.
1. Make sure the order of the channels is correct (RGB, BGR, depending on
the backend)
2. Mean subtraction by channel.
Parameters
----------
x : numpy array
The image
dim_ordering : string, optional (default: 'default')
Either 'th' for Theano or 'tf' for Tensorflow
Returns
-------
numpy array
The preprocessed image
"""
if dim_ordering == 'default':
dim_ordering = K.image_dim_ordering()
assert dim_ordering in {'tf', 'th'}
if dim_ordering == 'th':
x[:, 0, :, :] -= 103.939
x[:, 1, :, :] -= 116.779
x[:, 2, :, :] -= 123.68
# 'RGB'->'BGR'
x = x[:, ::-1, :, :]
else:
x[:, :, :, 0] -= 103.939
x[:, :, :, 1] -= 116.779
x[:, :, :, 2] -= 123.68
# 'RGB'->'BGR'
x = x[:, :, :, ::-1]
return x
def decode_predictions(preds, top=5):
"""
Decode the predictionso of the ImageNet trained network.
Parameters
----------
preds : numpy array
top : int
How many predictions to return
Returns
-------
list of tuples
e.g. (u'n02206856', u'bee', 0.71072823) for the WordNet identifier,
the class name and the probability.
"""
global CLASS_INDEX
if len(preds.shape) != 2 or preds.shape[1] != 1000:
raise ValueError('`decode_predictions` expects '
'a batch of predictions '
'(i.e. a 2D array of shape (samples, 1000)). '
'Found array with shape: ' + str(preds.shape))
if CLASS_INDEX is None:
fpath = get_file('imagenet_class_index.json',
CLASS_INDEX_PATH,
cache_subdir='models')
CLASS_INDEX = json.load(open(fpath))
results =
for pred in preds:
top_indices = pred.argsort()[-top:][::-1]
result = [tuple(CLASS_INDEX[str(i)]) + (pred[i],) for i in top_indices]
results.append(result)
return results
def is_valid_file(parser, arg):
"""
Check if arg is a valid file that already exists on the file system.
Parameters
----------
parser : argparse object
arg : str
Returns
-------
arg
"""
arg = os.path.abspath(arg)
if not os.path.exists(arg):
parser.error("The file %s does not exist!" % arg)
else:
return arg
def get_parser():
"""Get parser object."""
from argparse import ArgumentParser, ArgumentDefaultsHelpFormatter
parser = ArgumentParser(description=__doc__,
formatter_class=ArgumentDefaultsHelpFormatter)
parser.add_argument("-f", "--file",
dest="filename",
type=lambda x: is_valid_file(parser, x),
help="Classify image",
metavar="IMAGE",
required=True)
return parser
if __name__ == "__main__":
args = get_parser().parse_args()
# Load model
model = Xception(include_top=True, weights='imagenet')
img_path = args.filename
img = image.load_img(img_path, target_size=(224, 224))
x = image.img_to_array(img)
x = np.expand_dims(x, axis=0)
x = preprocess_input(x)
print('Input image shape:', x.shape)
t0 = time.time()
preds = model.predict(x)
t1 = time.time()
print("Prediction time: {:0.3f}s".format(t1 - t0))
for wordnet_id, class_name, prob in decode_predictions(preds)[0]:
print("{wid}t{prob:>6}%t{name}".format(wid=wordnet_id,
name=class_name,
prob="%0.2f" % (prob * 100)))
Why it works with any size
Look at the model.summary()
of Xception, especially the output shape. Notice the global average pooling layer? Before that, the shape is determined by the input. Meaning until that point, it can be anything.
Global pooling is another type of transition layer. It applies pooling over the complete feature map size to shrink the input to a constant 1 × 1 feature map and hence allows one network to have different input sizes.
Source: Me: Analysis and Optimization of Convolutional Neural Network Architectures
edited 10 hours ago
answered 12 hours ago
Martin ThomaMartin Thoma
6,1501353127
6,1501353127
$begingroup$
Reshaping of what exactly ? Input tensor ? In that case, would not we receive something like that for RGB images: [450, 600, 3] -> [1, 1, 3] ?
$endgroup$
– I.D.M
11 hours ago
$begingroup$
I've edited the example quite a bit. Turns out that you actually don't need to change anything.
$endgroup$
– Martin Thoma
10 hours ago
$begingroup$
Thank you very much for your extended response. I have a few more questions: 1. Why the documentation says that the default input size is 299x299 ? 2. It seems to me that there is some broken formatting at output shape - eg before GlobalAveragePooling2 it should probably be (None, None, None, 2048) instead (None, None, None, 2 3. Should I change only the Dense layer ?
$endgroup$
– I.D.M
9 hours ago
add a comment |
$begingroup$
Reshaping of what exactly ? Input tensor ? In that case, would not we receive something like that for RGB images: [450, 600, 3] -> [1, 1, 3] ?
$endgroup$
– I.D.M
11 hours ago
$begingroup$
I've edited the example quite a bit. Turns out that you actually don't need to change anything.
$endgroup$
– Martin Thoma
10 hours ago
$begingroup$
Thank you very much for your extended response. I have a few more questions: 1. Why the documentation says that the default input size is 299x299 ? 2. It seems to me that there is some broken formatting at output shape - eg before GlobalAveragePooling2 it should probably be (None, None, None, 2048) instead (None, None, None, 2 3. Should I change only the Dense layer ?
$endgroup$
– I.D.M
9 hours ago
$begingroup$
Reshaping of what exactly ? Input tensor ? In that case, would not we receive something like that for RGB images: [450, 600, 3] -> [1, 1, 3] ?
$endgroup$
– I.D.M
11 hours ago
$begingroup$
Reshaping of what exactly ? Input tensor ? In that case, would not we receive something like that for RGB images: [450, 600, 3] -> [1, 1, 3] ?
$endgroup$
– I.D.M
11 hours ago
$begingroup$
I've edited the example quite a bit. Turns out that you actually don't need to change anything.
$endgroup$
– Martin Thoma
10 hours ago
$begingroup$
I've edited the example quite a bit. Turns out that you actually don't need to change anything.
$endgroup$
– Martin Thoma
10 hours ago
$begingroup$
Thank you very much for your extended response. I have a few more questions: 1. Why the documentation says that the default input size is 299x299 ? 2. It seems to me that there is some broken formatting at output shape - eg before GlobalAveragePooling2 it should probably be (None, None, None, 2048) instead (None, None, None, 2 3. Should I change only the Dense layer ?
$endgroup$
– I.D.M
9 hours ago
$begingroup$
Thank you very much for your extended response. I have a few more questions: 1. Why the documentation says that the default input size is 299x299 ? 2. It seems to me that there is some broken formatting at output shape - eg before GlobalAveragePooling2 it should probably be (None, None, None, 2048) instead (None, None, None, 2 3. Should I change only the Dense layer ?
$endgroup$
– I.D.M
9 hours ago
add a comment |
Thanks for contributing an answer to Data Science Stack Exchange!
- Please be sure to answer the question. Provide details and share your research!
But avoid …
- Asking for help, clarification, or responding to other answers.
- Making statements based on opinion; back them up with references or personal experience.
Use MathJax to format equations. MathJax reference.
To learn more, see our tips on writing great answers.
Sign up or log in
StackExchange.ready(function () {
StackExchange.helpers.onClickDraftSave('#login-link');
});
Sign up using Google
Sign up using Facebook
Sign up using Email and Password
Post as a guest
Required, but never shown
StackExchange.ready(
function () {
StackExchange.openid.initPostLogin('.new-post-login', 'https%3a%2f%2fdatascience.stackexchange.com%2fquestions%2f45393%2fkeras-applications-using-images-larger-than-the-default-size%23new-answer', 'question_page');
}
);
Post as a guest
Required, but never shown
Sign up or log in
StackExchange.ready(function () {
StackExchange.helpers.onClickDraftSave('#login-link');
});
Sign up using Google
Sign up using Facebook
Sign up using Email and Password
Post as a guest
Required, but never shown
Sign up or log in
StackExchange.ready(function () {
StackExchange.helpers.onClickDraftSave('#login-link');
});
Sign up using Google
Sign up using Facebook
Sign up using Email and Password
Post as a guest
Required, but never shown
Sign up or log in
StackExchange.ready(function () {
StackExchange.helpers.onClickDraftSave('#login-link');
});
Sign up using Google
Sign up using Facebook
Sign up using Email and Password
Sign up using Google
Sign up using Facebook
Sign up using Email and Password
Post as a guest
Required, but never shown
Required, but never shown
Required, but never shown
Required, but never shown
Required, but never shown
Required, but never shown
Required, but never shown
Required, but never shown
Required, but never shown
uvhDsYetPzZ7rnRDeSlskAs24hX,R2gVxqM38sRK4o5RfpF7J3f16MgpK j 0aRsAP