What is the correct way to compute lift in lift charts
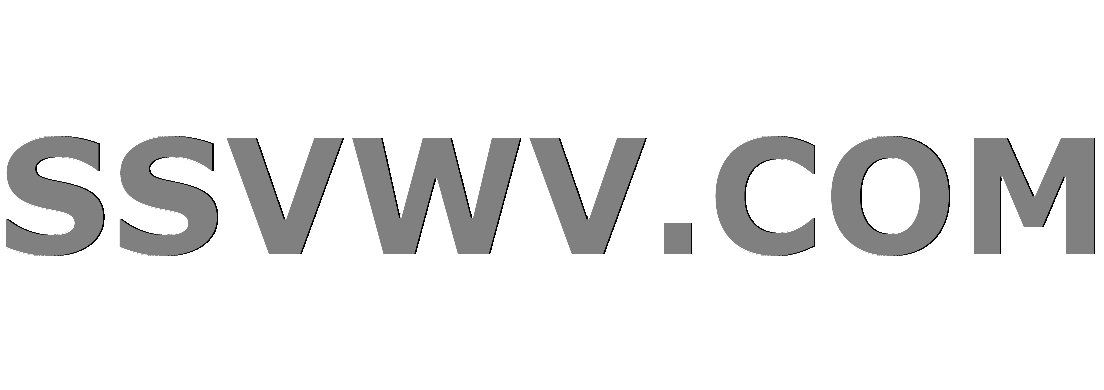
Multi tool use
$begingroup$
How is "lift" computed? i was reading about "Gain and lift charts" in data science.
I picked the following example from https://www.listendata.com/2014/08/excel-template-gain-and-lift-charts.html
I am clear on how the gain values are computed. Not clear about lift values are computed? (last column in table)
machine-learning metric
$endgroup$
bumped to the homepage by Community♦ 4 mins ago
This question has answers that may be good or bad; the system has marked it active so that they can be reviewed.
add a comment |
$begingroup$
How is "lift" computed? i was reading about "Gain and lift charts" in data science.
I picked the following example from https://www.listendata.com/2014/08/excel-template-gain-and-lift-charts.html
I am clear on how the gain values are computed. Not clear about lift values are computed? (last column in table)
machine-learning metric
$endgroup$
bumped to the homepage by Community♦ 4 mins ago
This question has answers that may be good or bad; the system has marked it active so that they can be reviewed.
add a comment |
$begingroup$
How is "lift" computed? i was reading about "Gain and lift charts" in data science.
I picked the following example from https://www.listendata.com/2014/08/excel-template-gain-and-lift-charts.html
I am clear on how the gain values are computed. Not clear about lift values are computed? (last column in table)
machine-learning metric
$endgroup$
How is "lift" computed? i was reading about "Gain and lift charts" in data science.
I picked the following example from https://www.listendata.com/2014/08/excel-template-gain-and-lift-charts.html
I am clear on how the gain values are computed. Not clear about lift values are computed? (last column in table)
machine-learning metric
machine-learning metric
asked Jul 27 '18 at 10:34


Anuj GuptaAnuj Gupta
1667
1667
bumped to the homepage by Community♦ 4 mins ago
This question has answers that may be good or bad; the system has marked it active so that they can be reviewed.
bumped to the homepage by Community♦ 4 mins ago
This question has answers that may be good or bad; the system has marked it active so that they can be reviewed.
add a comment |
add a comment |
1 Answer
1
active
oldest
votes
$begingroup$
Lift is computed by comparing performance with a random selection model. I'll explain with your example below,
- assume that we didn't have any statistical/ML model for ranking/scoring the respondents.
- In that case assume we did a random ordering of respondents.
- A decile (10% of total population) is expected to have 10% of the respondents. In your case, there should've been (approximately) 488 respondents in 2500 cases.
- But after ordering the cases by score, you are seeing 44.71% of the cases in first decile against expected 10% (in random/no model case). This gives the gain of 44.71/10 = 4.471.
- For next decile, cumulatively you have covered 20% of the cases. You'd expect a random/no model scenario covers 20% of the respondents. But using scores, we covered 80% of them. That gives a cumulative lift of 80/20 = 4.
$endgroup$
add a comment |
Your Answer
StackExchange.ready(function() {
var channelOptions = {
tags: "".split(" "),
id: "557"
};
initTagRenderer("".split(" "), "".split(" "), channelOptions);
StackExchange.using("externalEditor", function() {
// Have to fire editor after snippets, if snippets enabled
if (StackExchange.settings.snippets.snippetsEnabled) {
StackExchange.using("snippets", function() {
createEditor();
});
}
else {
createEditor();
}
});
function createEditor() {
StackExchange.prepareEditor({
heartbeatType: 'answer',
autoActivateHeartbeat: false,
convertImagesToLinks: false,
noModals: true,
showLowRepImageUploadWarning: true,
reputationToPostImages: null,
bindNavPrevention: true,
postfix: "",
imageUploader: {
brandingHtml: "Powered by u003ca class="icon-imgur-white" href="https://imgur.com/"u003eu003c/au003e",
contentPolicyHtml: "User contributions licensed under u003ca href="https://creativecommons.org/licenses/by-sa/3.0/"u003ecc by-sa 3.0 with attribution requiredu003c/au003e u003ca href="https://stackoverflow.com/legal/content-policy"u003e(content policy)u003c/au003e",
allowUrls: true
},
onDemand: true,
discardSelector: ".discard-answer"
,immediatelyShowMarkdownHelp:true
});
}
});
Sign up or log in
StackExchange.ready(function () {
StackExchange.helpers.onClickDraftSave('#login-link');
});
Sign up using Google
Sign up using Facebook
Sign up using Email and Password
Post as a guest
Required, but never shown
StackExchange.ready(
function () {
StackExchange.openid.initPostLogin('.new-post-login', 'https%3a%2f%2fdatascience.stackexchange.com%2fquestions%2f36092%2fwhat-is-the-correct-way-to-compute-lift-in-lift-charts%23new-answer', 'question_page');
}
);
Post as a guest
Required, but never shown
1 Answer
1
active
oldest
votes
1 Answer
1
active
oldest
votes
active
oldest
votes
active
oldest
votes
$begingroup$
Lift is computed by comparing performance with a random selection model. I'll explain with your example below,
- assume that we didn't have any statistical/ML model for ranking/scoring the respondents.
- In that case assume we did a random ordering of respondents.
- A decile (10% of total population) is expected to have 10% of the respondents. In your case, there should've been (approximately) 488 respondents in 2500 cases.
- But after ordering the cases by score, you are seeing 44.71% of the cases in first decile against expected 10% (in random/no model case). This gives the gain of 44.71/10 = 4.471.
- For next decile, cumulatively you have covered 20% of the cases. You'd expect a random/no model scenario covers 20% of the respondents. But using scores, we covered 80% of them. That gives a cumulative lift of 80/20 = 4.
$endgroup$
add a comment |
$begingroup$
Lift is computed by comparing performance with a random selection model. I'll explain with your example below,
- assume that we didn't have any statistical/ML model for ranking/scoring the respondents.
- In that case assume we did a random ordering of respondents.
- A decile (10% of total population) is expected to have 10% of the respondents. In your case, there should've been (approximately) 488 respondents in 2500 cases.
- But after ordering the cases by score, you are seeing 44.71% of the cases in first decile against expected 10% (in random/no model case). This gives the gain of 44.71/10 = 4.471.
- For next decile, cumulatively you have covered 20% of the cases. You'd expect a random/no model scenario covers 20% of the respondents. But using scores, we covered 80% of them. That gives a cumulative lift of 80/20 = 4.
$endgroup$
add a comment |
$begingroup$
Lift is computed by comparing performance with a random selection model. I'll explain with your example below,
- assume that we didn't have any statistical/ML model for ranking/scoring the respondents.
- In that case assume we did a random ordering of respondents.
- A decile (10% of total population) is expected to have 10% of the respondents. In your case, there should've been (approximately) 488 respondents in 2500 cases.
- But after ordering the cases by score, you are seeing 44.71% of the cases in first decile against expected 10% (in random/no model case). This gives the gain of 44.71/10 = 4.471.
- For next decile, cumulatively you have covered 20% of the cases. You'd expect a random/no model scenario covers 20% of the respondents. But using scores, we covered 80% of them. That gives a cumulative lift of 80/20 = 4.
$endgroup$
Lift is computed by comparing performance with a random selection model. I'll explain with your example below,
- assume that we didn't have any statistical/ML model for ranking/scoring the respondents.
- In that case assume we did a random ordering of respondents.
- A decile (10% of total population) is expected to have 10% of the respondents. In your case, there should've been (approximately) 488 respondents in 2500 cases.
- But after ordering the cases by score, you are seeing 44.71% of the cases in first decile against expected 10% (in random/no model case). This gives the gain of 44.71/10 = 4.471.
- For next decile, cumulatively you have covered 20% of the cases. You'd expect a random/no model scenario covers 20% of the respondents. But using scores, we covered 80% of them. That gives a cumulative lift of 80/20 = 4.
answered Jul 30 '18 at 9:16
hssayhssay
1,0931311
1,0931311
add a comment |
add a comment |
Thanks for contributing an answer to Data Science Stack Exchange!
- Please be sure to answer the question. Provide details and share your research!
But avoid …
- Asking for help, clarification, or responding to other answers.
- Making statements based on opinion; back them up with references or personal experience.
Use MathJax to format equations. MathJax reference.
To learn more, see our tips on writing great answers.
Sign up or log in
StackExchange.ready(function () {
StackExchange.helpers.onClickDraftSave('#login-link');
});
Sign up using Google
Sign up using Facebook
Sign up using Email and Password
Post as a guest
Required, but never shown
StackExchange.ready(
function () {
StackExchange.openid.initPostLogin('.new-post-login', 'https%3a%2f%2fdatascience.stackexchange.com%2fquestions%2f36092%2fwhat-is-the-correct-way-to-compute-lift-in-lift-charts%23new-answer', 'question_page');
}
);
Post as a guest
Required, but never shown
Sign up or log in
StackExchange.ready(function () {
StackExchange.helpers.onClickDraftSave('#login-link');
});
Sign up using Google
Sign up using Facebook
Sign up using Email and Password
Post as a guest
Required, but never shown
Sign up or log in
StackExchange.ready(function () {
StackExchange.helpers.onClickDraftSave('#login-link');
});
Sign up using Google
Sign up using Facebook
Sign up using Email and Password
Post as a guest
Required, but never shown
Sign up or log in
StackExchange.ready(function () {
StackExchange.helpers.onClickDraftSave('#login-link');
});
Sign up using Google
Sign up using Facebook
Sign up using Email and Password
Sign up using Google
Sign up using Facebook
Sign up using Email and Password
Post as a guest
Required, but never shown
Required, but never shown
Required, but never shown
Required, but never shown
Required, but never shown
Required, but never shown
Required, but never shown
Required, but never shown
Required, but never shown
dc5 YvHw0t7nW,N8vXb2a,v,X1WWeQXY1lx 28