How to improve the time series predictions using Random Forest?
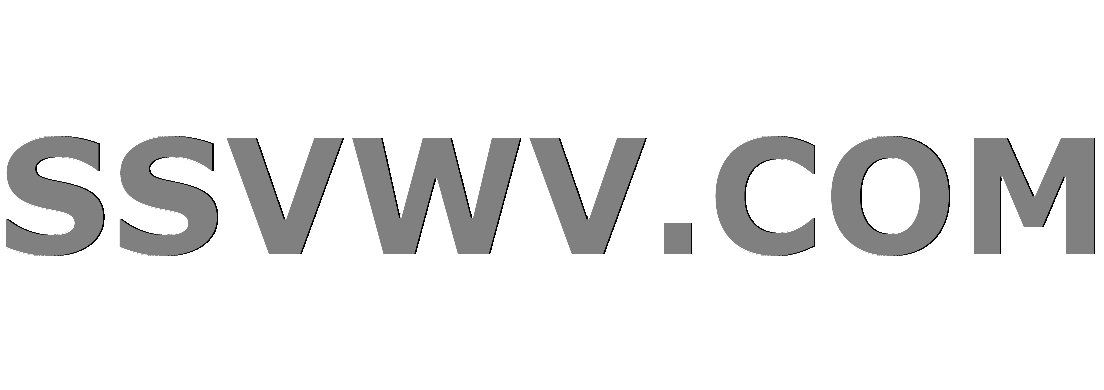
Multi tool use
$begingroup$
We are running RandomForest model on a time-series data. The model is run in real time and is refit every time a new row is added. Since it is a timeseries data, we set shuffle to false while splitting into train and test dataset.
We observed that there is a drastic change in scores when shuffle is True and when shuffle is false
The code being used is as follows
# Set shuffle = 'True' or 'False'
df = pandas.read_csv('data.csv', index_col=0)
X = df.drop(columns=['label'])
y = df['label']
X_train, X_test, y_train, y_test = train_test_split(X, y, test_size=0.05, shuffle=True)
count = 0
predictions =
for idx in X_test.index.values:
# Train the model on training data
# print(count, X_train.shape, y_train.shape)
rf = RandomForestRegressor(n_estimators = 600, max_depth = 7, random_state = 12345)
rf.fit(X_train, y_train)
predictions.append(rf.predict(X_test.loc[X_test.index == idx]))
# print(len(predictions))
X_train.loc[len(X_train)] = X_test.loc[idx]
y_train.loc[len(y_train)] = y_test.loc[idx]
count+=1
Initially, we thought the difference is due to covariance shift in the data. But that shouldn't affect this much for continuous fit
Here are screenshots for the prediction plot
With shuffle = False
With shuffle = True
time-series predictive-modeling random-forest training transfer-learning
New contributor
Sumesh Surendran is a new contributor to this site. Take care in asking for clarification, commenting, and answering.
Check out our Code of Conduct.
$endgroup$
add a comment |
$begingroup$
We are running RandomForest model on a time-series data. The model is run in real time and is refit every time a new row is added. Since it is a timeseries data, we set shuffle to false while splitting into train and test dataset.
We observed that there is a drastic change in scores when shuffle is True and when shuffle is false
The code being used is as follows
# Set shuffle = 'True' or 'False'
df = pandas.read_csv('data.csv', index_col=0)
X = df.drop(columns=['label'])
y = df['label']
X_train, X_test, y_train, y_test = train_test_split(X, y, test_size=0.05, shuffle=True)
count = 0
predictions =
for idx in X_test.index.values:
# Train the model on training data
# print(count, X_train.shape, y_train.shape)
rf = RandomForestRegressor(n_estimators = 600, max_depth = 7, random_state = 12345)
rf.fit(X_train, y_train)
predictions.append(rf.predict(X_test.loc[X_test.index == idx]))
# print(len(predictions))
X_train.loc[len(X_train)] = X_test.loc[idx]
y_train.loc[len(y_train)] = y_test.loc[idx]
count+=1
Initially, we thought the difference is due to covariance shift in the data. But that shouldn't affect this much for continuous fit
Here are screenshots for the prediction plot
With shuffle = False
With shuffle = True
time-series predictive-modeling random-forest training transfer-learning
New contributor
Sumesh Surendran is a new contributor to this site. Take care in asking for clarification, commenting, and answering.
Check out our Code of Conduct.
$endgroup$
add a comment |
$begingroup$
We are running RandomForest model on a time-series data. The model is run in real time and is refit every time a new row is added. Since it is a timeseries data, we set shuffle to false while splitting into train and test dataset.
We observed that there is a drastic change in scores when shuffle is True and when shuffle is false
The code being used is as follows
# Set shuffle = 'True' or 'False'
df = pandas.read_csv('data.csv', index_col=0)
X = df.drop(columns=['label'])
y = df['label']
X_train, X_test, y_train, y_test = train_test_split(X, y, test_size=0.05, shuffle=True)
count = 0
predictions =
for idx in X_test.index.values:
# Train the model on training data
# print(count, X_train.shape, y_train.shape)
rf = RandomForestRegressor(n_estimators = 600, max_depth = 7, random_state = 12345)
rf.fit(X_train, y_train)
predictions.append(rf.predict(X_test.loc[X_test.index == idx]))
# print(len(predictions))
X_train.loc[len(X_train)] = X_test.loc[idx]
y_train.loc[len(y_train)] = y_test.loc[idx]
count+=1
Initially, we thought the difference is due to covariance shift in the data. But that shouldn't affect this much for continuous fit
Here are screenshots for the prediction plot
With shuffle = False
With shuffle = True
time-series predictive-modeling random-forest training transfer-learning
New contributor
Sumesh Surendran is a new contributor to this site. Take care in asking for clarification, commenting, and answering.
Check out our Code of Conduct.
$endgroup$
We are running RandomForest model on a time-series data. The model is run in real time and is refit every time a new row is added. Since it is a timeseries data, we set shuffle to false while splitting into train and test dataset.
We observed that there is a drastic change in scores when shuffle is True and when shuffle is false
The code being used is as follows
# Set shuffle = 'True' or 'False'
df = pandas.read_csv('data.csv', index_col=0)
X = df.drop(columns=['label'])
y = df['label']
X_train, X_test, y_train, y_test = train_test_split(X, y, test_size=0.05, shuffle=True)
count = 0
predictions =
for idx in X_test.index.values:
# Train the model on training data
# print(count, X_train.shape, y_train.shape)
rf = RandomForestRegressor(n_estimators = 600, max_depth = 7, random_state = 12345)
rf.fit(X_train, y_train)
predictions.append(rf.predict(X_test.loc[X_test.index == idx]))
# print(len(predictions))
X_train.loc[len(X_train)] = X_test.loc[idx]
y_train.loc[len(y_train)] = y_test.loc[idx]
count+=1
Initially, we thought the difference is due to covariance shift in the data. But that shouldn't affect this much for continuous fit
Here are screenshots for the prediction plot
With shuffle = False
With shuffle = True
time-series predictive-modeling random-forest training transfer-learning
time-series predictive-modeling random-forest training transfer-learning
New contributor
Sumesh Surendran is a new contributor to this site. Take care in asking for clarification, commenting, and answering.
Check out our Code of Conduct.
New contributor
Sumesh Surendran is a new contributor to this site. Take care in asking for clarification, commenting, and answering.
Check out our Code of Conduct.
New contributor
Sumesh Surendran is a new contributor to this site. Take care in asking for clarification, commenting, and answering.
Check out our Code of Conduct.
asked 4 mins ago


Sumesh SurendranSumesh Surendran
1
1
New contributor
Sumesh Surendran is a new contributor to this site. Take care in asking for clarification, commenting, and answering.
Check out our Code of Conduct.
New contributor
Sumesh Surendran is a new contributor to this site. Take care in asking for clarification, commenting, and answering.
Check out our Code of Conduct.
Sumesh Surendran is a new contributor to this site. Take care in asking for clarification, commenting, and answering.
Check out our Code of Conduct.
add a comment |
add a comment |
0
active
oldest
votes
Your Answer
StackExchange.ifUsing("editor", function () {
return StackExchange.using("mathjaxEditing", function () {
StackExchange.MarkdownEditor.creationCallbacks.add(function (editor, postfix) {
StackExchange.mathjaxEditing.prepareWmdForMathJax(editor, postfix, [["$", "$"], ["\\(","\\)"]]);
});
});
}, "mathjax-editing");
StackExchange.ready(function() {
var channelOptions = {
tags: "".split(" "),
id: "557"
};
initTagRenderer("".split(" "), "".split(" "), channelOptions);
StackExchange.using("externalEditor", function() {
// Have to fire editor after snippets, if snippets enabled
if (StackExchange.settings.snippets.snippetsEnabled) {
StackExchange.using("snippets", function() {
createEditor();
});
}
else {
createEditor();
}
});
function createEditor() {
StackExchange.prepareEditor({
heartbeatType: 'answer',
autoActivateHeartbeat: false,
convertImagesToLinks: false,
noModals: true,
showLowRepImageUploadWarning: true,
reputationToPostImages: null,
bindNavPrevention: true,
postfix: "",
imageUploader: {
brandingHtml: "Powered by u003ca class="icon-imgur-white" href="https://imgur.com/"u003eu003c/au003e",
contentPolicyHtml: "User contributions licensed under u003ca href="https://creativecommons.org/licenses/by-sa/3.0/"u003ecc by-sa 3.0 with attribution requiredu003c/au003e u003ca href="https://stackoverflow.com/legal/content-policy"u003e(content policy)u003c/au003e",
allowUrls: true
},
onDemand: true,
discardSelector: ".discard-answer"
,immediatelyShowMarkdownHelp:true
});
}
});
Sumesh Surendran is a new contributor. Be nice, and check out our Code of Conduct.
Sign up or log in
StackExchange.ready(function () {
StackExchange.helpers.onClickDraftSave('#login-link');
});
Sign up using Google
Sign up using Facebook
Sign up using Email and Password
Post as a guest
Required, but never shown
StackExchange.ready(
function () {
StackExchange.openid.initPostLogin('.new-post-login', 'https%3a%2f%2fdatascience.stackexchange.com%2fquestions%2f45672%2fhow-to-improve-the-time-series-predictions-using-random-forest%23new-answer', 'question_page');
}
);
Post as a guest
Required, but never shown
0
active
oldest
votes
0
active
oldest
votes
active
oldest
votes
active
oldest
votes
Sumesh Surendran is a new contributor. Be nice, and check out our Code of Conduct.
Sumesh Surendran is a new contributor. Be nice, and check out our Code of Conduct.
Sumesh Surendran is a new contributor. Be nice, and check out our Code of Conduct.
Sumesh Surendran is a new contributor. Be nice, and check out our Code of Conduct.
Thanks for contributing an answer to Data Science Stack Exchange!
- Please be sure to answer the question. Provide details and share your research!
But avoid …
- Asking for help, clarification, or responding to other answers.
- Making statements based on opinion; back them up with references or personal experience.
Use MathJax to format equations. MathJax reference.
To learn more, see our tips on writing great answers.
Sign up or log in
StackExchange.ready(function () {
StackExchange.helpers.onClickDraftSave('#login-link');
});
Sign up using Google
Sign up using Facebook
Sign up using Email and Password
Post as a guest
Required, but never shown
StackExchange.ready(
function () {
StackExchange.openid.initPostLogin('.new-post-login', 'https%3a%2f%2fdatascience.stackexchange.com%2fquestions%2f45672%2fhow-to-improve-the-time-series-predictions-using-random-forest%23new-answer', 'question_page');
}
);
Post as a guest
Required, but never shown
Sign up or log in
StackExchange.ready(function () {
StackExchange.helpers.onClickDraftSave('#login-link');
});
Sign up using Google
Sign up using Facebook
Sign up using Email and Password
Post as a guest
Required, but never shown
Sign up or log in
StackExchange.ready(function () {
StackExchange.helpers.onClickDraftSave('#login-link');
});
Sign up using Google
Sign up using Facebook
Sign up using Email and Password
Post as a guest
Required, but never shown
Sign up or log in
StackExchange.ready(function () {
StackExchange.helpers.onClickDraftSave('#login-link');
});
Sign up using Google
Sign up using Facebook
Sign up using Email and Password
Sign up using Google
Sign up using Facebook
Sign up using Email and Password
Post as a guest
Required, but never shown
Required, but never shown
Required, but never shown
Required, but never shown
Required, but never shown
Required, but never shown
Required, but never shown
Required, but never shown
Required, but never shown
dMhsr2 1e q upsO5XcrDW3yIC0iyWkYA VntHJD tJfV UJIzYxpN iFGitywW mKuh g3,lr1GmwjrR40znQRQ5OCL1szsAHm5UIHgAYi