What are features for state-action pairs in RL?
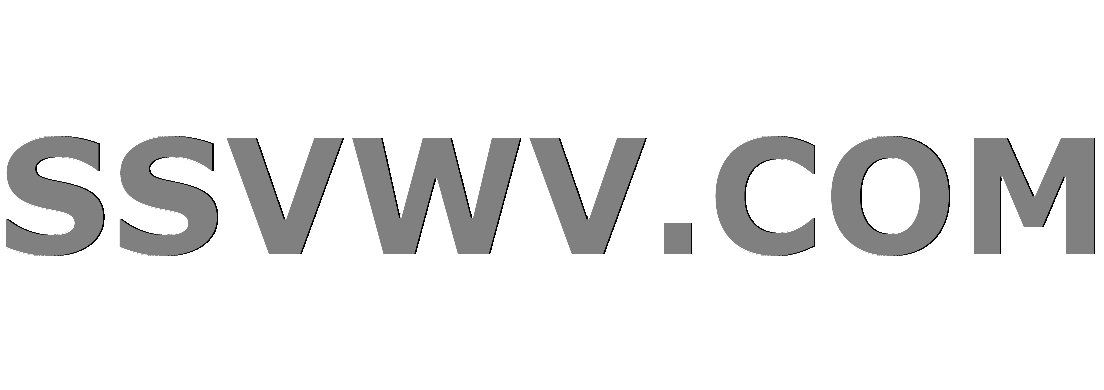
Multi tool use
$begingroup$
I read this answer: What are features in the context of reinforcement learning?
But it only describes features for the state only in the context of cartpole, ie. Cart Position, Cart Velocity, Pole Angle, Pole Velocity At Tip
On slide 18 here: http://www.cs.cmu.edu/~rsalakhu/10703/Lecture_VFA.pdf
It states:
But does not give examples. I started reading from p. 198 in Sutton's book for Value Function Approximation but also did not see examples for "features of state-action pairs" .
My best guess is for example in Cartpole-V1 (discrete action space) would be to add one more number to the tuple describing the state-action pair, ie. (Cart Position, Cart Velocity, Pole Angle, Pole Velocity At Tip, push_right) .
In the case of Cartpole I guess each state action pair could be described with a feature vector of length 3 where the final input for the tuple is either "push_left", "do_nothing", "push_right".
Would the immediate reward from taking one of the actions also be included in the tuples that form the state-action feature vector?
reinforcement-learning feature-construction
New contributor
flexitarian33 is a new contributor to this site. Take care in asking for clarification, commenting, and answering.
Check out our Code of Conduct.
$endgroup$
add a comment |
$begingroup$
I read this answer: What are features in the context of reinforcement learning?
But it only describes features for the state only in the context of cartpole, ie. Cart Position, Cart Velocity, Pole Angle, Pole Velocity At Tip
On slide 18 here: http://www.cs.cmu.edu/~rsalakhu/10703/Lecture_VFA.pdf
It states:
But does not give examples. I started reading from p. 198 in Sutton's book for Value Function Approximation but also did not see examples for "features of state-action pairs" .
My best guess is for example in Cartpole-V1 (discrete action space) would be to add one more number to the tuple describing the state-action pair, ie. (Cart Position, Cart Velocity, Pole Angle, Pole Velocity At Tip, push_right) .
In the case of Cartpole I guess each state action pair could be described with a feature vector of length 3 where the final input for the tuple is either "push_left", "do_nothing", "push_right".
Would the immediate reward from taking one of the actions also be included in the tuples that form the state-action feature vector?
reinforcement-learning feature-construction
New contributor
flexitarian33 is a new contributor to this site. Take care in asking for clarification, commenting, and answering.
Check out our Code of Conduct.
$endgroup$
1
$begingroup$
Your questions about David Silver's policy gradient lecture should be posted separately. He wasn't talking about feature construction. He was talking about how the policy is parameterized and learned.
$endgroup$
– Philip Raeisghasem
yesterday
$begingroup$
Hey didn't realize I was off topic as I was just trying to show my chain-of-thought and what I was concurrently looking at, ie. I was trying to find some common ground for gradients across a wide range of algorithms.
$endgroup$
– flexitarian33
yesterday
$begingroup$
No problem! If you have questions about policy gradients you can't find the answers to, I or someone else here would be happy to answer them.
$endgroup$
– Philip Raeisghasem
yesterday
add a comment |
$begingroup$
I read this answer: What are features in the context of reinforcement learning?
But it only describes features for the state only in the context of cartpole, ie. Cart Position, Cart Velocity, Pole Angle, Pole Velocity At Tip
On slide 18 here: http://www.cs.cmu.edu/~rsalakhu/10703/Lecture_VFA.pdf
It states:
But does not give examples. I started reading from p. 198 in Sutton's book for Value Function Approximation but also did not see examples for "features of state-action pairs" .
My best guess is for example in Cartpole-V1 (discrete action space) would be to add one more number to the tuple describing the state-action pair, ie. (Cart Position, Cart Velocity, Pole Angle, Pole Velocity At Tip, push_right) .
In the case of Cartpole I guess each state action pair could be described with a feature vector of length 3 where the final input for the tuple is either "push_left", "do_nothing", "push_right".
Would the immediate reward from taking one of the actions also be included in the tuples that form the state-action feature vector?
reinforcement-learning feature-construction
New contributor
flexitarian33 is a new contributor to this site. Take care in asking for clarification, commenting, and answering.
Check out our Code of Conduct.
$endgroup$
I read this answer: What are features in the context of reinforcement learning?
But it only describes features for the state only in the context of cartpole, ie. Cart Position, Cart Velocity, Pole Angle, Pole Velocity At Tip
On slide 18 here: http://www.cs.cmu.edu/~rsalakhu/10703/Lecture_VFA.pdf
It states:
But does not give examples. I started reading from p. 198 in Sutton's book for Value Function Approximation but also did not see examples for "features of state-action pairs" .
My best guess is for example in Cartpole-V1 (discrete action space) would be to add one more number to the tuple describing the state-action pair, ie. (Cart Position, Cart Velocity, Pole Angle, Pole Velocity At Tip, push_right) .
In the case of Cartpole I guess each state action pair could be described with a feature vector of length 3 where the final input for the tuple is either "push_left", "do_nothing", "push_right".
Would the immediate reward from taking one of the actions also be included in the tuples that form the state-action feature vector?
reinforcement-learning feature-construction
reinforcement-learning feature-construction
New contributor
flexitarian33 is a new contributor to this site. Take care in asking for clarification, commenting, and answering.
Check out our Code of Conduct.
New contributor
flexitarian33 is a new contributor to this site. Take care in asking for clarification, commenting, and answering.
Check out our Code of Conduct.
edited yesterday


Philip Raeisghasem
1735
1735
New contributor
flexitarian33 is a new contributor to this site. Take care in asking for clarification, commenting, and answering.
Check out our Code of Conduct.
asked yesterday
flexitarian33flexitarian33
256
256
New contributor
flexitarian33 is a new contributor to this site. Take care in asking for clarification, commenting, and answering.
Check out our Code of Conduct.
New contributor
flexitarian33 is a new contributor to this site. Take care in asking for clarification, commenting, and answering.
Check out our Code of Conduct.
flexitarian33 is a new contributor to this site. Take care in asking for clarification, commenting, and answering.
Check out our Code of Conduct.
1
$begingroup$
Your questions about David Silver's policy gradient lecture should be posted separately. He wasn't talking about feature construction. He was talking about how the policy is parameterized and learned.
$endgroup$
– Philip Raeisghasem
yesterday
$begingroup$
Hey didn't realize I was off topic as I was just trying to show my chain-of-thought and what I was concurrently looking at, ie. I was trying to find some common ground for gradients across a wide range of algorithms.
$endgroup$
– flexitarian33
yesterday
$begingroup$
No problem! If you have questions about policy gradients you can't find the answers to, I or someone else here would be happy to answer them.
$endgroup$
– Philip Raeisghasem
yesterday
add a comment |
1
$begingroup$
Your questions about David Silver's policy gradient lecture should be posted separately. He wasn't talking about feature construction. He was talking about how the policy is parameterized and learned.
$endgroup$
– Philip Raeisghasem
yesterday
$begingroup$
Hey didn't realize I was off topic as I was just trying to show my chain-of-thought and what I was concurrently looking at, ie. I was trying to find some common ground for gradients across a wide range of algorithms.
$endgroup$
– flexitarian33
yesterday
$begingroup$
No problem! If you have questions about policy gradients you can't find the answers to, I or someone else here would be happy to answer them.
$endgroup$
– Philip Raeisghasem
yesterday
1
1
$begingroup$
Your questions about David Silver's policy gradient lecture should be posted separately. He wasn't talking about feature construction. He was talking about how the policy is parameterized and learned.
$endgroup$
– Philip Raeisghasem
yesterday
$begingroup$
Your questions about David Silver's policy gradient lecture should be posted separately. He wasn't talking about feature construction. He was talking about how the policy is parameterized and learned.
$endgroup$
– Philip Raeisghasem
yesterday
$begingroup$
Hey didn't realize I was off topic as I was just trying to show my chain-of-thought and what I was concurrently looking at, ie. I was trying to find some common ground for gradients across a wide range of algorithms.
$endgroup$
– flexitarian33
yesterday
$begingroup$
Hey didn't realize I was off topic as I was just trying to show my chain-of-thought and what I was concurrently looking at, ie. I was trying to find some common ground for gradients across a wide range of algorithms.
$endgroup$
– flexitarian33
yesterday
$begingroup$
No problem! If you have questions about policy gradients you can't find the answers to, I or someone else here would be happy to answer them.
$endgroup$
– Philip Raeisghasem
yesterday
$begingroup$
No problem! If you have questions about policy gradients you can't find the answers to, I or someone else here would be happy to answer them.
$endgroup$
– Philip Raeisghasem
yesterday
add a comment |
1 Answer
1
active
oldest
votes
$begingroup$
In the cartpole example, a state-action feature would be
$$begin{bmatrix}
text{Cart Position}\
text{Cart Velocity}\
text{Pole Angle}\
text{Pole Tip Velocity}\
text{Action}
end{bmatrix}$$
where Action is either left, right, or do nothing. The reward is not part of the feature vector because reward does not describe the state of the agent; it is not an input. It is a (possibly stochastic) signal received from the environment that the agent is trying to predict/control with the use of feature vectors.
New contributor
Philip Raeisghasem is a new contributor to this site. Take care in asking for clarification, commenting, and answering.
Check out our Code of Conduct.
$endgroup$
add a comment |
Your Answer
StackExchange.ifUsing("editor", function () {
return StackExchange.using("mathjaxEditing", function () {
StackExchange.MarkdownEditor.creationCallbacks.add(function (editor, postfix) {
StackExchange.mathjaxEditing.prepareWmdForMathJax(editor, postfix, [["$", "$"], ["\\(","\\)"]]);
});
});
}, "mathjax-editing");
StackExchange.ready(function() {
var channelOptions = {
tags: "".split(" "),
id: "557"
};
initTagRenderer("".split(" "), "".split(" "), channelOptions);
StackExchange.using("externalEditor", function() {
// Have to fire editor after snippets, if snippets enabled
if (StackExchange.settings.snippets.snippetsEnabled) {
StackExchange.using("snippets", function() {
createEditor();
});
}
else {
createEditor();
}
});
function createEditor() {
StackExchange.prepareEditor({
heartbeatType: 'answer',
autoActivateHeartbeat: false,
convertImagesToLinks: false,
noModals: true,
showLowRepImageUploadWarning: true,
reputationToPostImages: null,
bindNavPrevention: true,
postfix: "",
imageUploader: {
brandingHtml: "Powered by u003ca class="icon-imgur-white" href="https://imgur.com/"u003eu003c/au003e",
contentPolicyHtml: "User contributions licensed under u003ca href="https://creativecommons.org/licenses/by-sa/3.0/"u003ecc by-sa 3.0 with attribution requiredu003c/au003e u003ca href="https://stackoverflow.com/legal/content-policy"u003e(content policy)u003c/au003e",
allowUrls: true
},
onDemand: true,
discardSelector: ".discard-answer"
,immediatelyShowMarkdownHelp:true
});
}
});
flexitarian33 is a new contributor. Be nice, and check out our Code of Conduct.
Sign up or log in
StackExchange.ready(function () {
StackExchange.helpers.onClickDraftSave('#login-link');
});
Sign up using Google
Sign up using Facebook
Sign up using Email and Password
Post as a guest
Required, but never shown
StackExchange.ready(
function () {
StackExchange.openid.initPostLogin('.new-post-login', 'https%3a%2f%2fdatascience.stackexchange.com%2fquestions%2f47456%2fwhat-are-features-for-state-action-pairs-in-rl%23new-answer', 'question_page');
}
);
Post as a guest
Required, but never shown
1 Answer
1
active
oldest
votes
1 Answer
1
active
oldest
votes
active
oldest
votes
active
oldest
votes
$begingroup$
In the cartpole example, a state-action feature would be
$$begin{bmatrix}
text{Cart Position}\
text{Cart Velocity}\
text{Pole Angle}\
text{Pole Tip Velocity}\
text{Action}
end{bmatrix}$$
where Action is either left, right, or do nothing. The reward is not part of the feature vector because reward does not describe the state of the agent; it is not an input. It is a (possibly stochastic) signal received from the environment that the agent is trying to predict/control with the use of feature vectors.
New contributor
Philip Raeisghasem is a new contributor to this site. Take care in asking for clarification, commenting, and answering.
Check out our Code of Conduct.
$endgroup$
add a comment |
$begingroup$
In the cartpole example, a state-action feature would be
$$begin{bmatrix}
text{Cart Position}\
text{Cart Velocity}\
text{Pole Angle}\
text{Pole Tip Velocity}\
text{Action}
end{bmatrix}$$
where Action is either left, right, or do nothing. The reward is not part of the feature vector because reward does not describe the state of the agent; it is not an input. It is a (possibly stochastic) signal received from the environment that the agent is trying to predict/control with the use of feature vectors.
New contributor
Philip Raeisghasem is a new contributor to this site. Take care in asking for clarification, commenting, and answering.
Check out our Code of Conduct.
$endgroup$
add a comment |
$begingroup$
In the cartpole example, a state-action feature would be
$$begin{bmatrix}
text{Cart Position}\
text{Cart Velocity}\
text{Pole Angle}\
text{Pole Tip Velocity}\
text{Action}
end{bmatrix}$$
where Action is either left, right, or do nothing. The reward is not part of the feature vector because reward does not describe the state of the agent; it is not an input. It is a (possibly stochastic) signal received from the environment that the agent is trying to predict/control with the use of feature vectors.
New contributor
Philip Raeisghasem is a new contributor to this site. Take care in asking for clarification, commenting, and answering.
Check out our Code of Conduct.
$endgroup$
In the cartpole example, a state-action feature would be
$$begin{bmatrix}
text{Cart Position}\
text{Cart Velocity}\
text{Pole Angle}\
text{Pole Tip Velocity}\
text{Action}
end{bmatrix}$$
where Action is either left, right, or do nothing. The reward is not part of the feature vector because reward does not describe the state of the agent; it is not an input. It is a (possibly stochastic) signal received from the environment that the agent is trying to predict/control with the use of feature vectors.
New contributor
Philip Raeisghasem is a new contributor to this site. Take care in asking for clarification, commenting, and answering.
Check out our Code of Conduct.
New contributor
Philip Raeisghasem is a new contributor to this site. Take care in asking for clarification, commenting, and answering.
Check out our Code of Conduct.
answered yesterday


Philip RaeisghasemPhilip Raeisghasem
1735
1735
New contributor
Philip Raeisghasem is a new contributor to this site. Take care in asking for clarification, commenting, and answering.
Check out our Code of Conduct.
New contributor
Philip Raeisghasem is a new contributor to this site. Take care in asking for clarification, commenting, and answering.
Check out our Code of Conduct.
Philip Raeisghasem is a new contributor to this site. Take care in asking for clarification, commenting, and answering.
Check out our Code of Conduct.
add a comment |
add a comment |
flexitarian33 is a new contributor. Be nice, and check out our Code of Conduct.
flexitarian33 is a new contributor. Be nice, and check out our Code of Conduct.
flexitarian33 is a new contributor. Be nice, and check out our Code of Conduct.
flexitarian33 is a new contributor. Be nice, and check out our Code of Conduct.
Thanks for contributing an answer to Data Science Stack Exchange!
- Please be sure to answer the question. Provide details and share your research!
But avoid …
- Asking for help, clarification, or responding to other answers.
- Making statements based on opinion; back them up with references or personal experience.
Use MathJax to format equations. MathJax reference.
To learn more, see our tips on writing great answers.
Sign up or log in
StackExchange.ready(function () {
StackExchange.helpers.onClickDraftSave('#login-link');
});
Sign up using Google
Sign up using Facebook
Sign up using Email and Password
Post as a guest
Required, but never shown
StackExchange.ready(
function () {
StackExchange.openid.initPostLogin('.new-post-login', 'https%3a%2f%2fdatascience.stackexchange.com%2fquestions%2f47456%2fwhat-are-features-for-state-action-pairs-in-rl%23new-answer', 'question_page');
}
);
Post as a guest
Required, but never shown
Sign up or log in
StackExchange.ready(function () {
StackExchange.helpers.onClickDraftSave('#login-link');
});
Sign up using Google
Sign up using Facebook
Sign up using Email and Password
Post as a guest
Required, but never shown
Sign up or log in
StackExchange.ready(function () {
StackExchange.helpers.onClickDraftSave('#login-link');
});
Sign up using Google
Sign up using Facebook
Sign up using Email and Password
Post as a guest
Required, but never shown
Sign up or log in
StackExchange.ready(function () {
StackExchange.helpers.onClickDraftSave('#login-link');
});
Sign up using Google
Sign up using Facebook
Sign up using Email and Password
Sign up using Google
Sign up using Facebook
Sign up using Email and Password
Post as a guest
Required, but never shown
Required, but never shown
Required, but never shown
Required, but never shown
Required, but never shown
Required, but never shown
Required, but never shown
Required, but never shown
Required, but never shown
x9pTYt3TV1 Fp9P b3shCW1aTLuOoELOmqGhSqy ZXjUHilNikaapB,aj6Y7umN075ItmVbl3V2e7
1
$begingroup$
Your questions about David Silver's policy gradient lecture should be posted separately. He wasn't talking about feature construction. He was talking about how the policy is parameterized and learned.
$endgroup$
– Philip Raeisghasem
yesterday
$begingroup$
Hey didn't realize I was off topic as I was just trying to show my chain-of-thought and what I was concurrently looking at, ie. I was trying to find some common ground for gradients across a wide range of algorithms.
$endgroup$
– flexitarian33
yesterday
$begingroup$
No problem! If you have questions about policy gradients you can't find the answers to, I or someone else here would be happy to answer them.
$endgroup$
– Philip Raeisghasem
yesterday