Precision vs. Recall
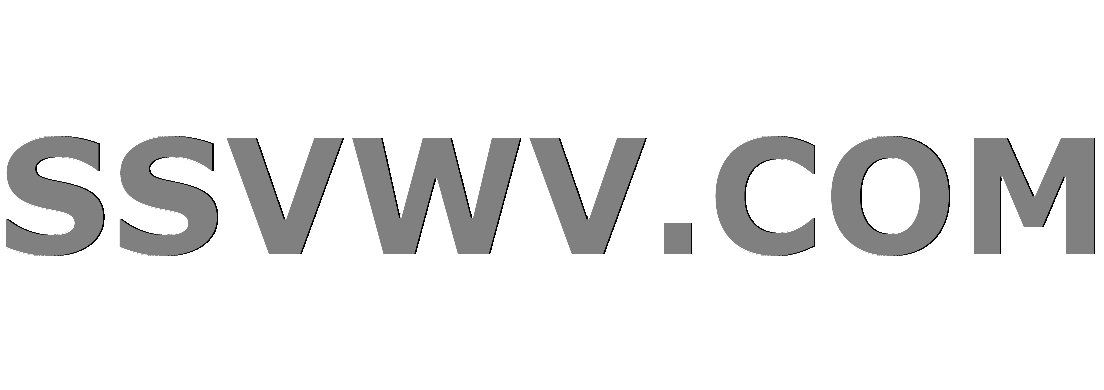
Multi tool use
$begingroup$
Can I make the following statement about a binary classification, please?
Precision 1: 0.10
Recall 1: 0.83
Statement: "We can expect 90% false alarms (1 - 0.10). But for the remaining 10%, we can be around 83% certain (Recall 1: 0.83), that we caught a label 1."
Thanks in advance!
machine-learning classification
$endgroup$
add a comment |
$begingroup$
Can I make the following statement about a binary classification, please?
Precision 1: 0.10
Recall 1: 0.83
Statement: "We can expect 90% false alarms (1 - 0.10). But for the remaining 10%, we can be around 83% certain (Recall 1: 0.83), that we caught a label 1."
Thanks in advance!
machine-learning classification
$endgroup$
add a comment |
$begingroup$
Can I make the following statement about a binary classification, please?
Precision 1: 0.10
Recall 1: 0.83
Statement: "We can expect 90% false alarms (1 - 0.10). But for the remaining 10%, we can be around 83% certain (Recall 1: 0.83), that we caught a label 1."
Thanks in advance!
machine-learning classification
$endgroup$
Can I make the following statement about a binary classification, please?
Precision 1: 0.10
Recall 1: 0.83
Statement: "We can expect 90% false alarms (1 - 0.10). But for the remaining 10%, we can be around 83% certain (Recall 1: 0.83), that we caught a label 1."
Thanks in advance!
machine-learning classification
machine-learning classification
edited yesterday
HFulcher
1228
1228
asked yesterday
FrancoSwissFrancoSwiss
7115
7115
add a comment |
add a comment |
1 Answer
1
active
oldest
votes
$begingroup$
I would phrase it like so:
"Of all records that were labelled 1 by the model, 10% were actually 1 (90% incorrect predictions). Of all records that were truly labelled 1 we predicted 83% correctly."
While this is out of context of your question, if support refers to the number of records then it would be beneficial to get a more balanced dataset. The reason your precision is so poor for label 1 is because there are many more "negatives" (0's) than "positives" (1) increasing the chance for false positives to occur, affecting your precision.
EDIT:
This on Cross Validated will help provide more explanation.
New contributor
HFulcher is a new contributor to this site. Take care in asking for clarification, commenting, and answering.
Check out our Code of Conduct.
$endgroup$
$begingroup$
Thank you for your explanation HFulcher! This helps a lot. Poor label? It's 35 million entries with 0.25% label 1. AUROC 0.97. I would call that a success.
$endgroup$
– FrancoSwiss
yesterday
$begingroup$
@FrancoSwiss happy to help! A high ROC score doesn't necessarily mean that your model has succeeded in dealing with one of the labels well. You can see from the F1 scores that the model is heavily biased towards predicting 0 due to the imbalance in the training set. I don't know the constraints of your dataset so this could very well be a success in this context! If you feel that I have answered your question sufficiently please mark it as answered, otherwise I would be happy to elaborate :)
$endgroup$
– HFulcher
yesterday
add a comment |
Your Answer
StackExchange.ifUsing("editor", function () {
return StackExchange.using("mathjaxEditing", function () {
StackExchange.MarkdownEditor.creationCallbacks.add(function (editor, postfix) {
StackExchange.mathjaxEditing.prepareWmdForMathJax(editor, postfix, [["$", "$"], ["\\(","\\)"]]);
});
});
}, "mathjax-editing");
StackExchange.ready(function() {
var channelOptions = {
tags: "".split(" "),
id: "557"
};
initTagRenderer("".split(" "), "".split(" "), channelOptions);
StackExchange.using("externalEditor", function() {
// Have to fire editor after snippets, if snippets enabled
if (StackExchange.settings.snippets.snippetsEnabled) {
StackExchange.using("snippets", function() {
createEditor();
});
}
else {
createEditor();
}
});
function createEditor() {
StackExchange.prepareEditor({
heartbeatType: 'answer',
autoActivateHeartbeat: false,
convertImagesToLinks: false,
noModals: true,
showLowRepImageUploadWarning: true,
reputationToPostImages: null,
bindNavPrevention: true,
postfix: "",
imageUploader: {
brandingHtml: "Powered by u003ca class="icon-imgur-white" href="https://imgur.com/"u003eu003c/au003e",
contentPolicyHtml: "User contributions licensed under u003ca href="https://creativecommons.org/licenses/by-sa/3.0/"u003ecc by-sa 3.0 with attribution requiredu003c/au003e u003ca href="https://stackoverflow.com/legal/content-policy"u003e(content policy)u003c/au003e",
allowUrls: true
},
onDemand: true,
discardSelector: ".discard-answer"
,immediatelyShowMarkdownHelp:true
});
}
});
Sign up or log in
StackExchange.ready(function () {
StackExchange.helpers.onClickDraftSave('#login-link');
});
Sign up using Google
Sign up using Facebook
Sign up using Email and Password
Post as a guest
Required, but never shown
StackExchange.ready(
function () {
StackExchange.openid.initPostLogin('.new-post-login', 'https%3a%2f%2fdatascience.stackexchange.com%2fquestions%2f46044%2fprecision-vs-recall%23new-answer', 'question_page');
}
);
Post as a guest
Required, but never shown
1 Answer
1
active
oldest
votes
1 Answer
1
active
oldest
votes
active
oldest
votes
active
oldest
votes
$begingroup$
I would phrase it like so:
"Of all records that were labelled 1 by the model, 10% were actually 1 (90% incorrect predictions). Of all records that were truly labelled 1 we predicted 83% correctly."
While this is out of context of your question, if support refers to the number of records then it would be beneficial to get a more balanced dataset. The reason your precision is so poor for label 1 is because there are many more "negatives" (0's) than "positives" (1) increasing the chance for false positives to occur, affecting your precision.
EDIT:
This on Cross Validated will help provide more explanation.
New contributor
HFulcher is a new contributor to this site. Take care in asking for clarification, commenting, and answering.
Check out our Code of Conduct.
$endgroup$
$begingroup$
Thank you for your explanation HFulcher! This helps a lot. Poor label? It's 35 million entries with 0.25% label 1. AUROC 0.97. I would call that a success.
$endgroup$
– FrancoSwiss
yesterday
$begingroup$
@FrancoSwiss happy to help! A high ROC score doesn't necessarily mean that your model has succeeded in dealing with one of the labels well. You can see from the F1 scores that the model is heavily biased towards predicting 0 due to the imbalance in the training set. I don't know the constraints of your dataset so this could very well be a success in this context! If you feel that I have answered your question sufficiently please mark it as answered, otherwise I would be happy to elaborate :)
$endgroup$
– HFulcher
yesterday
add a comment |
$begingroup$
I would phrase it like so:
"Of all records that were labelled 1 by the model, 10% were actually 1 (90% incorrect predictions). Of all records that were truly labelled 1 we predicted 83% correctly."
While this is out of context of your question, if support refers to the number of records then it would be beneficial to get a more balanced dataset. The reason your precision is so poor for label 1 is because there are many more "negatives" (0's) than "positives" (1) increasing the chance for false positives to occur, affecting your precision.
EDIT:
This on Cross Validated will help provide more explanation.
New contributor
HFulcher is a new contributor to this site. Take care in asking for clarification, commenting, and answering.
Check out our Code of Conduct.
$endgroup$
$begingroup$
Thank you for your explanation HFulcher! This helps a lot. Poor label? It's 35 million entries with 0.25% label 1. AUROC 0.97. I would call that a success.
$endgroup$
– FrancoSwiss
yesterday
$begingroup$
@FrancoSwiss happy to help! A high ROC score doesn't necessarily mean that your model has succeeded in dealing with one of the labels well. You can see from the F1 scores that the model is heavily biased towards predicting 0 due to the imbalance in the training set. I don't know the constraints of your dataset so this could very well be a success in this context! If you feel that I have answered your question sufficiently please mark it as answered, otherwise I would be happy to elaborate :)
$endgroup$
– HFulcher
yesterday
add a comment |
$begingroup$
I would phrase it like so:
"Of all records that were labelled 1 by the model, 10% were actually 1 (90% incorrect predictions). Of all records that were truly labelled 1 we predicted 83% correctly."
While this is out of context of your question, if support refers to the number of records then it would be beneficial to get a more balanced dataset. The reason your precision is so poor for label 1 is because there are many more "negatives" (0's) than "positives" (1) increasing the chance for false positives to occur, affecting your precision.
EDIT:
This on Cross Validated will help provide more explanation.
New contributor
HFulcher is a new contributor to this site. Take care in asking for clarification, commenting, and answering.
Check out our Code of Conduct.
$endgroup$
I would phrase it like so:
"Of all records that were labelled 1 by the model, 10% were actually 1 (90% incorrect predictions). Of all records that were truly labelled 1 we predicted 83% correctly."
While this is out of context of your question, if support refers to the number of records then it would be beneficial to get a more balanced dataset. The reason your precision is so poor for label 1 is because there are many more "negatives" (0's) than "positives" (1) increasing the chance for false positives to occur, affecting your precision.
EDIT:
This on Cross Validated will help provide more explanation.
New contributor
HFulcher is a new contributor to this site. Take care in asking for clarification, commenting, and answering.
Check out our Code of Conduct.
New contributor
HFulcher is a new contributor to this site. Take care in asking for clarification, commenting, and answering.
Check out our Code of Conduct.
answered yesterday
HFulcherHFulcher
1228
1228
New contributor
HFulcher is a new contributor to this site. Take care in asking for clarification, commenting, and answering.
Check out our Code of Conduct.
New contributor
HFulcher is a new contributor to this site. Take care in asking for clarification, commenting, and answering.
Check out our Code of Conduct.
HFulcher is a new contributor to this site. Take care in asking for clarification, commenting, and answering.
Check out our Code of Conduct.
$begingroup$
Thank you for your explanation HFulcher! This helps a lot. Poor label? It's 35 million entries with 0.25% label 1. AUROC 0.97. I would call that a success.
$endgroup$
– FrancoSwiss
yesterday
$begingroup$
@FrancoSwiss happy to help! A high ROC score doesn't necessarily mean that your model has succeeded in dealing with one of the labels well. You can see from the F1 scores that the model is heavily biased towards predicting 0 due to the imbalance in the training set. I don't know the constraints of your dataset so this could very well be a success in this context! If you feel that I have answered your question sufficiently please mark it as answered, otherwise I would be happy to elaborate :)
$endgroup$
– HFulcher
yesterday
add a comment |
$begingroup$
Thank you for your explanation HFulcher! This helps a lot. Poor label? It's 35 million entries with 0.25% label 1. AUROC 0.97. I would call that a success.
$endgroup$
– FrancoSwiss
yesterday
$begingroup$
@FrancoSwiss happy to help! A high ROC score doesn't necessarily mean that your model has succeeded in dealing with one of the labels well. You can see from the F1 scores that the model is heavily biased towards predicting 0 due to the imbalance in the training set. I don't know the constraints of your dataset so this could very well be a success in this context! If you feel that I have answered your question sufficiently please mark it as answered, otherwise I would be happy to elaborate :)
$endgroup$
– HFulcher
yesterday
$begingroup$
Thank you for your explanation HFulcher! This helps a lot. Poor label? It's 35 million entries with 0.25% label 1. AUROC 0.97. I would call that a success.
$endgroup$
– FrancoSwiss
yesterday
$begingroup$
Thank you for your explanation HFulcher! This helps a lot. Poor label? It's 35 million entries with 0.25% label 1. AUROC 0.97. I would call that a success.
$endgroup$
– FrancoSwiss
yesterday
$begingroup$
@FrancoSwiss happy to help! A high ROC score doesn't necessarily mean that your model has succeeded in dealing with one of the labels well. You can see from the F1 scores that the model is heavily biased towards predicting 0 due to the imbalance in the training set. I don't know the constraints of your dataset so this could very well be a success in this context! If you feel that I have answered your question sufficiently please mark it as answered, otherwise I would be happy to elaborate :)
$endgroup$
– HFulcher
yesterday
$begingroup$
@FrancoSwiss happy to help! A high ROC score doesn't necessarily mean that your model has succeeded in dealing with one of the labels well. You can see from the F1 scores that the model is heavily biased towards predicting 0 due to the imbalance in the training set. I don't know the constraints of your dataset so this could very well be a success in this context! If you feel that I have answered your question sufficiently please mark it as answered, otherwise I would be happy to elaborate :)
$endgroup$
– HFulcher
yesterday
add a comment |
Thanks for contributing an answer to Data Science Stack Exchange!
- Please be sure to answer the question. Provide details and share your research!
But avoid …
- Asking for help, clarification, or responding to other answers.
- Making statements based on opinion; back them up with references or personal experience.
Use MathJax to format equations. MathJax reference.
To learn more, see our tips on writing great answers.
Sign up or log in
StackExchange.ready(function () {
StackExchange.helpers.onClickDraftSave('#login-link');
});
Sign up using Google
Sign up using Facebook
Sign up using Email and Password
Post as a guest
Required, but never shown
StackExchange.ready(
function () {
StackExchange.openid.initPostLogin('.new-post-login', 'https%3a%2f%2fdatascience.stackexchange.com%2fquestions%2f46044%2fprecision-vs-recall%23new-answer', 'question_page');
}
);
Post as a guest
Required, but never shown
Sign up or log in
StackExchange.ready(function () {
StackExchange.helpers.onClickDraftSave('#login-link');
});
Sign up using Google
Sign up using Facebook
Sign up using Email and Password
Post as a guest
Required, but never shown
Sign up or log in
StackExchange.ready(function () {
StackExchange.helpers.onClickDraftSave('#login-link');
});
Sign up using Google
Sign up using Facebook
Sign up using Email and Password
Post as a guest
Required, but never shown
Sign up or log in
StackExchange.ready(function () {
StackExchange.helpers.onClickDraftSave('#login-link');
});
Sign up using Google
Sign up using Facebook
Sign up using Email and Password
Sign up using Google
Sign up using Facebook
Sign up using Email and Password
Post as a guest
Required, but never shown
Required, but never shown
Required, but never shown
Required, but never shown
Required, but never shown
Required, but never shown
Required, but never shown
Required, but never shown
Required, but never shown
6WWblrd,fZVHUWG,YUdNKuR,lTSp,OUXFJQg3XpXngPAHid4bEO6UYmZBCJx