logistic regression : highly sensitive model
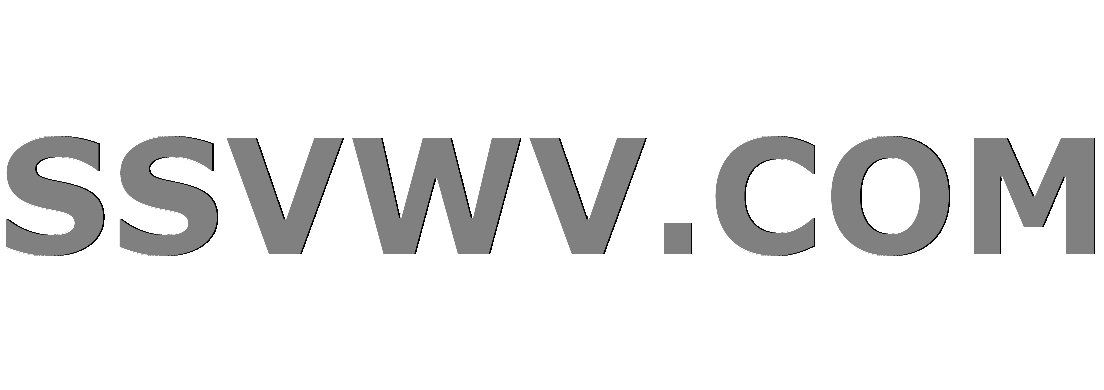
Multi tool use
$begingroup$
I am a newbie to data science and ML. I am working on a classification problem where the task is to predict loan status (granted/not granted).
I am running a logistic regression model on the data. The accuracy of my model is 82%. However, my model is more sensitive (sensitivity = 97%) and less specific(specificity = 53%).
I want to increase the model's specificity. At this stage, after referring to a bunch of internet resources, I am confused about how to proceed.
Below is my observation :
In Testing data,
a percentage of 1's in the class label is 73.17073170731707.
Testing data has more 1's than 0's in the class label. Is this the reason behind model being highly sensitive.
I am attaching my data file and code file. Please take a look at it.
Data sample :
Process :
Data --> missing value imputation -->distribution analysis-->log transformation for normal distribution ---> one hot encoding --> feature selection --> splitting data --> model selection and evaluation
Code snippets :
Here I have selected "3 best features": Credit History, Property Area
How should I proceed? Any help (even if it's just a kick in the right direction) would be appreciated.
machine-learning logistic-regression
New contributor
blueWings is a new contributor to this site. Take care in asking for clarification, commenting, and answering.
Check out our Code of Conduct.
$endgroup$
add a comment |
$begingroup$
I am a newbie to data science and ML. I am working on a classification problem where the task is to predict loan status (granted/not granted).
I am running a logistic regression model on the data. The accuracy of my model is 82%. However, my model is more sensitive (sensitivity = 97%) and less specific(specificity = 53%).
I want to increase the model's specificity. At this stage, after referring to a bunch of internet resources, I am confused about how to proceed.
Below is my observation :
In Testing data,
a percentage of 1's in the class label is 73.17073170731707.
Testing data has more 1's than 0's in the class label. Is this the reason behind model being highly sensitive.
I am attaching my data file and code file. Please take a look at it.
Data sample :
Process :
Data --> missing value imputation -->distribution analysis-->log transformation for normal distribution ---> one hot encoding --> feature selection --> splitting data --> model selection and evaluation
Code snippets :
Here I have selected "3 best features": Credit History, Property Area
How should I proceed? Any help (even if it's just a kick in the right direction) would be appreciated.
machine-learning logistic-regression
New contributor
blueWings is a new contributor to this site. Take care in asking for clarification, commenting, and answering.
Check out our Code of Conduct.
$endgroup$
$begingroup$
Can you share some instances of the data? Perhaps there are more appropriate models than a logistic regression. Also you can weight the loss caused by 1's more heavily.
$endgroup$
– JahKnows
yesterday
$begingroup$
The Google Drive link will sooner or later be dead. Keep in mind that your question may be useful for somebody in the future. So, could you please add some sample lines of your data and the relevant code snippets to your question.
$endgroup$
– georg_un
yesterday
1
$begingroup$
@georg_un I have updated the question.
$endgroup$
– blueWings
yesterday
$begingroup$
@JahKnows I have tried SVM with RBF kernel. But still, I am getting the same sensitivity and specificity. Also, I didn't get intuition behind weighting the loss by 1's
$endgroup$
– blueWings
yesterday
add a comment |
$begingroup$
I am a newbie to data science and ML. I am working on a classification problem where the task is to predict loan status (granted/not granted).
I am running a logistic regression model on the data. The accuracy of my model is 82%. However, my model is more sensitive (sensitivity = 97%) and less specific(specificity = 53%).
I want to increase the model's specificity. At this stage, after referring to a bunch of internet resources, I am confused about how to proceed.
Below is my observation :
In Testing data,
a percentage of 1's in the class label is 73.17073170731707.
Testing data has more 1's than 0's in the class label. Is this the reason behind model being highly sensitive.
I am attaching my data file and code file. Please take a look at it.
Data sample :
Process :
Data --> missing value imputation -->distribution analysis-->log transformation for normal distribution ---> one hot encoding --> feature selection --> splitting data --> model selection and evaluation
Code snippets :
Here I have selected "3 best features": Credit History, Property Area
How should I proceed? Any help (even if it's just a kick in the right direction) would be appreciated.
machine-learning logistic-regression
New contributor
blueWings is a new contributor to this site. Take care in asking for clarification, commenting, and answering.
Check out our Code of Conduct.
$endgroup$
I am a newbie to data science and ML. I am working on a classification problem where the task is to predict loan status (granted/not granted).
I am running a logistic regression model on the data. The accuracy of my model is 82%. However, my model is more sensitive (sensitivity = 97%) and less specific(specificity = 53%).
I want to increase the model's specificity. At this stage, after referring to a bunch of internet resources, I am confused about how to proceed.
Below is my observation :
In Testing data,
a percentage of 1's in the class label is 73.17073170731707.
Testing data has more 1's than 0's in the class label. Is this the reason behind model being highly sensitive.
I am attaching my data file and code file. Please take a look at it.
Data sample :
Process :
Data --> missing value imputation -->distribution analysis-->log transformation for normal distribution ---> one hot encoding --> feature selection --> splitting data --> model selection and evaluation
Code snippets :
Here I have selected "3 best features": Credit History, Property Area
How should I proceed? Any help (even if it's just a kick in the right direction) would be appreciated.
machine-learning logistic-regression
machine-learning logistic-regression
New contributor
blueWings is a new contributor to this site. Take care in asking for clarification, commenting, and answering.
Check out our Code of Conduct.
New contributor
blueWings is a new contributor to this site. Take care in asking for clarification, commenting, and answering.
Check out our Code of Conduct.
edited yesterday
blueWings
New contributor
blueWings is a new contributor to this site. Take care in asking for clarification, commenting, and answering.
Check out our Code of Conduct.
asked yesterday
blueWingsblueWings
133
133
New contributor
blueWings is a new contributor to this site. Take care in asking for clarification, commenting, and answering.
Check out our Code of Conduct.
New contributor
blueWings is a new contributor to this site. Take care in asking for clarification, commenting, and answering.
Check out our Code of Conduct.
blueWings is a new contributor to this site. Take care in asking for clarification, commenting, and answering.
Check out our Code of Conduct.
$begingroup$
Can you share some instances of the data? Perhaps there are more appropriate models than a logistic regression. Also you can weight the loss caused by 1's more heavily.
$endgroup$
– JahKnows
yesterday
$begingroup$
The Google Drive link will sooner or later be dead. Keep in mind that your question may be useful for somebody in the future. So, could you please add some sample lines of your data and the relevant code snippets to your question.
$endgroup$
– georg_un
yesterday
1
$begingroup$
@georg_un I have updated the question.
$endgroup$
– blueWings
yesterday
$begingroup$
@JahKnows I have tried SVM with RBF kernel. But still, I am getting the same sensitivity and specificity. Also, I didn't get intuition behind weighting the loss by 1's
$endgroup$
– blueWings
yesterday
add a comment |
$begingroup$
Can you share some instances of the data? Perhaps there are more appropriate models than a logistic regression. Also you can weight the loss caused by 1's more heavily.
$endgroup$
– JahKnows
yesterday
$begingroup$
The Google Drive link will sooner or later be dead. Keep in mind that your question may be useful for somebody in the future. So, could you please add some sample lines of your data and the relevant code snippets to your question.
$endgroup$
– georg_un
yesterday
1
$begingroup$
@georg_un I have updated the question.
$endgroup$
– blueWings
yesterday
$begingroup$
@JahKnows I have tried SVM with RBF kernel. But still, I am getting the same sensitivity and specificity. Also, I didn't get intuition behind weighting the loss by 1's
$endgroup$
– blueWings
yesterday
$begingroup$
Can you share some instances of the data? Perhaps there are more appropriate models than a logistic regression. Also you can weight the loss caused by 1's more heavily.
$endgroup$
– JahKnows
yesterday
$begingroup$
Can you share some instances of the data? Perhaps there are more appropriate models than a logistic regression. Also you can weight the loss caused by 1's more heavily.
$endgroup$
– JahKnows
yesterday
$begingroup$
The Google Drive link will sooner or later be dead. Keep in mind that your question may be useful for somebody in the future. So, could you please add some sample lines of your data and the relevant code snippets to your question.
$endgroup$
– georg_un
yesterday
$begingroup$
The Google Drive link will sooner or later be dead. Keep in mind that your question may be useful for somebody in the future. So, could you please add some sample lines of your data and the relevant code snippets to your question.
$endgroup$
– georg_un
yesterday
1
1
$begingroup$
@georg_un I have updated the question.
$endgroup$
– blueWings
yesterday
$begingroup$
@georg_un I have updated the question.
$endgroup$
– blueWings
yesterday
$begingroup$
@JahKnows I have tried SVM with RBF kernel. But still, I am getting the same sensitivity and specificity. Also, I didn't get intuition behind weighting the loss by 1's
$endgroup$
– blueWings
yesterday
$begingroup$
@JahKnows I have tried SVM with RBF kernel. But still, I am getting the same sensitivity and specificity. Also, I didn't get intuition behind weighting the loss by 1's
$endgroup$
– blueWings
yesterday
add a comment |
2 Answers
2
active
oldest
votes
$begingroup$
Actually, what is happening is natural. There is a trade-off between sensitivity and specificity. If you want to increase the specificity, you should increase the threshold of your decision function but note that it comes at a price and the price is reducing the sensitivity.
$endgroup$
$begingroup$
I see. Thank you
$endgroup$
– blueWings
yesterday
$begingroup$
@blueWings You’re welcome. So, have you tried changing the threshold?
$endgroup$
– pythinker
yesterday
$begingroup$
Yes. I set the threshold to 0.7 and now my specificity increased to 57%. But as you said it came with less sensitivity that is 82%. ROC remained same 0.70.
$endgroup$
– blueWings
yesterday
add a comment |
$begingroup$
Just an idea. Have you tried 'playing' with C?
C is the inverse of regularization strength. Large values of C give more freedom to the model. Default C is 1.
A high C like 1000 can (not always) give you a higher variance and lower bias while you might overfit though.
Good luck!
$endgroup$
$begingroup$
I haven't. Thank you for useful insights.
$endgroup$
– blueWings
5 hours ago
add a comment |
Your Answer
StackExchange.ifUsing("editor", function () {
return StackExchange.using("mathjaxEditing", function () {
StackExchange.MarkdownEditor.creationCallbacks.add(function (editor, postfix) {
StackExchange.mathjaxEditing.prepareWmdForMathJax(editor, postfix, [["$", "$"], ["\\(","\\)"]]);
});
});
}, "mathjax-editing");
StackExchange.ready(function() {
var channelOptions = {
tags: "".split(" "),
id: "557"
};
initTagRenderer("".split(" "), "".split(" "), channelOptions);
StackExchange.using("externalEditor", function() {
// Have to fire editor after snippets, if snippets enabled
if (StackExchange.settings.snippets.snippetsEnabled) {
StackExchange.using("snippets", function() {
createEditor();
});
}
else {
createEditor();
}
});
function createEditor() {
StackExchange.prepareEditor({
heartbeatType: 'answer',
autoActivateHeartbeat: false,
convertImagesToLinks: false,
noModals: true,
showLowRepImageUploadWarning: true,
reputationToPostImages: null,
bindNavPrevention: true,
postfix: "",
imageUploader: {
brandingHtml: "Powered by u003ca class="icon-imgur-white" href="https://imgur.com/"u003eu003c/au003e",
contentPolicyHtml: "User contributions licensed under u003ca href="https://creativecommons.org/licenses/by-sa/3.0/"u003ecc by-sa 3.0 with attribution requiredu003c/au003e u003ca href="https://stackoverflow.com/legal/content-policy"u003e(content policy)u003c/au003e",
allowUrls: true
},
onDemand: true,
discardSelector: ".discard-answer"
,immediatelyShowMarkdownHelp:true
});
}
});
blueWings is a new contributor. Be nice, and check out our Code of Conduct.
Sign up or log in
StackExchange.ready(function () {
StackExchange.helpers.onClickDraftSave('#login-link');
});
Sign up using Google
Sign up using Facebook
Sign up using Email and Password
Post as a guest
Required, but never shown
StackExchange.ready(
function () {
StackExchange.openid.initPostLogin('.new-post-login', 'https%3a%2f%2fdatascience.stackexchange.com%2fquestions%2f48761%2flogistic-regression-highly-sensitive-model%23new-answer', 'question_page');
}
);
Post as a guest
Required, but never shown
2 Answers
2
active
oldest
votes
2 Answers
2
active
oldest
votes
active
oldest
votes
active
oldest
votes
$begingroup$
Actually, what is happening is natural. There is a trade-off between sensitivity and specificity. If you want to increase the specificity, you should increase the threshold of your decision function but note that it comes at a price and the price is reducing the sensitivity.
$endgroup$
$begingroup$
I see. Thank you
$endgroup$
– blueWings
yesterday
$begingroup$
@blueWings You’re welcome. So, have you tried changing the threshold?
$endgroup$
– pythinker
yesterday
$begingroup$
Yes. I set the threshold to 0.7 and now my specificity increased to 57%. But as you said it came with less sensitivity that is 82%. ROC remained same 0.70.
$endgroup$
– blueWings
yesterday
add a comment |
$begingroup$
Actually, what is happening is natural. There is a trade-off between sensitivity and specificity. If you want to increase the specificity, you should increase the threshold of your decision function but note that it comes at a price and the price is reducing the sensitivity.
$endgroup$
$begingroup$
I see. Thank you
$endgroup$
– blueWings
yesterday
$begingroup$
@blueWings You’re welcome. So, have you tried changing the threshold?
$endgroup$
– pythinker
yesterday
$begingroup$
Yes. I set the threshold to 0.7 and now my specificity increased to 57%. But as you said it came with less sensitivity that is 82%. ROC remained same 0.70.
$endgroup$
– blueWings
yesterday
add a comment |
$begingroup$
Actually, what is happening is natural. There is a trade-off between sensitivity and specificity. If you want to increase the specificity, you should increase the threshold of your decision function but note that it comes at a price and the price is reducing the sensitivity.
$endgroup$
Actually, what is happening is natural. There is a trade-off between sensitivity and specificity. If you want to increase the specificity, you should increase the threshold of your decision function but note that it comes at a price and the price is reducing the sensitivity.
answered yesterday


pythinkerpythinker
5031211
5031211
$begingroup$
I see. Thank you
$endgroup$
– blueWings
yesterday
$begingroup$
@blueWings You’re welcome. So, have you tried changing the threshold?
$endgroup$
– pythinker
yesterday
$begingroup$
Yes. I set the threshold to 0.7 and now my specificity increased to 57%. But as you said it came with less sensitivity that is 82%. ROC remained same 0.70.
$endgroup$
– blueWings
yesterday
add a comment |
$begingroup$
I see. Thank you
$endgroup$
– blueWings
yesterday
$begingroup$
@blueWings You’re welcome. So, have you tried changing the threshold?
$endgroup$
– pythinker
yesterday
$begingroup$
Yes. I set the threshold to 0.7 and now my specificity increased to 57%. But as you said it came with less sensitivity that is 82%. ROC remained same 0.70.
$endgroup$
– blueWings
yesterday
$begingroup$
I see. Thank you
$endgroup$
– blueWings
yesterday
$begingroup$
I see. Thank you
$endgroup$
– blueWings
yesterday
$begingroup$
@blueWings You’re welcome. So, have you tried changing the threshold?
$endgroup$
– pythinker
yesterday
$begingroup$
@blueWings You’re welcome. So, have you tried changing the threshold?
$endgroup$
– pythinker
yesterday
$begingroup$
Yes. I set the threshold to 0.7 and now my specificity increased to 57%. But as you said it came with less sensitivity that is 82%. ROC remained same 0.70.
$endgroup$
– blueWings
yesterday
$begingroup$
Yes. I set the threshold to 0.7 and now my specificity increased to 57%. But as you said it came with less sensitivity that is 82%. ROC remained same 0.70.
$endgroup$
– blueWings
yesterday
add a comment |
$begingroup$
Just an idea. Have you tried 'playing' with C?
C is the inverse of regularization strength. Large values of C give more freedom to the model. Default C is 1.
A high C like 1000 can (not always) give you a higher variance and lower bias while you might overfit though.
Good luck!
$endgroup$
$begingroup$
I haven't. Thank you for useful insights.
$endgroup$
– blueWings
5 hours ago
add a comment |
$begingroup$
Just an idea. Have you tried 'playing' with C?
C is the inverse of regularization strength. Large values of C give more freedom to the model. Default C is 1.
A high C like 1000 can (not always) give you a higher variance and lower bias while you might overfit though.
Good luck!
$endgroup$
$begingroup$
I haven't. Thank you for useful insights.
$endgroup$
– blueWings
5 hours ago
add a comment |
$begingroup$
Just an idea. Have you tried 'playing' with C?
C is the inverse of regularization strength. Large values of C give more freedom to the model. Default C is 1.
A high C like 1000 can (not always) give you a higher variance and lower bias while you might overfit though.
Good luck!
$endgroup$
Just an idea. Have you tried 'playing' with C?
C is the inverse of regularization strength. Large values of C give more freedom to the model. Default C is 1.
A high C like 1000 can (not always) give you a higher variance and lower bias while you might overfit though.
Good luck!
answered 11 hours ago
FrancoSwissFrancoSwiss
10115
10115
$begingroup$
I haven't. Thank you for useful insights.
$endgroup$
– blueWings
5 hours ago
add a comment |
$begingroup$
I haven't. Thank you for useful insights.
$endgroup$
– blueWings
5 hours ago
$begingroup$
I haven't. Thank you for useful insights.
$endgroup$
– blueWings
5 hours ago
$begingroup$
I haven't. Thank you for useful insights.
$endgroup$
– blueWings
5 hours ago
add a comment |
blueWings is a new contributor. Be nice, and check out our Code of Conduct.
blueWings is a new contributor. Be nice, and check out our Code of Conduct.
blueWings is a new contributor. Be nice, and check out our Code of Conduct.
blueWings is a new contributor. Be nice, and check out our Code of Conduct.
Thanks for contributing an answer to Data Science Stack Exchange!
- Please be sure to answer the question. Provide details and share your research!
But avoid …
- Asking for help, clarification, or responding to other answers.
- Making statements based on opinion; back them up with references or personal experience.
Use MathJax to format equations. MathJax reference.
To learn more, see our tips on writing great answers.
Sign up or log in
StackExchange.ready(function () {
StackExchange.helpers.onClickDraftSave('#login-link');
});
Sign up using Google
Sign up using Facebook
Sign up using Email and Password
Post as a guest
Required, but never shown
StackExchange.ready(
function () {
StackExchange.openid.initPostLogin('.new-post-login', 'https%3a%2f%2fdatascience.stackexchange.com%2fquestions%2f48761%2flogistic-regression-highly-sensitive-model%23new-answer', 'question_page');
}
);
Post as a guest
Required, but never shown
Sign up or log in
StackExchange.ready(function () {
StackExchange.helpers.onClickDraftSave('#login-link');
});
Sign up using Google
Sign up using Facebook
Sign up using Email and Password
Post as a guest
Required, but never shown
Sign up or log in
StackExchange.ready(function () {
StackExchange.helpers.onClickDraftSave('#login-link');
});
Sign up using Google
Sign up using Facebook
Sign up using Email and Password
Post as a guest
Required, but never shown
Sign up or log in
StackExchange.ready(function () {
StackExchange.helpers.onClickDraftSave('#login-link');
});
Sign up using Google
Sign up using Facebook
Sign up using Email and Password
Sign up using Google
Sign up using Facebook
Sign up using Email and Password
Post as a guest
Required, but never shown
Required, but never shown
Required, but never shown
Required, but never shown
Required, but never shown
Required, but never shown
Required, but never shown
Required, but never shown
Required, but never shown
XbWl6uGwEGKvN9V,IlYF3qDcnbKbUMZUmfic f4d57 nyq4yv3,QRU8vzYseEirp1 ESAmGo,hNW
$begingroup$
Can you share some instances of the data? Perhaps there are more appropriate models than a logistic regression. Also you can weight the loss caused by 1's more heavily.
$endgroup$
– JahKnows
yesterday
$begingroup$
The Google Drive link will sooner or later be dead. Keep in mind that your question may be useful for somebody in the future. So, could you please add some sample lines of your data and the relevant code snippets to your question.
$endgroup$
– georg_un
yesterday
1
$begingroup$
@georg_un I have updated the question.
$endgroup$
– blueWings
yesterday
$begingroup$
@JahKnows I have tried SVM with RBF kernel. But still, I am getting the same sensitivity and specificity. Also, I didn't get intuition behind weighting the loss by 1's
$endgroup$
– blueWings
yesterday